Adding Immigrants to Microsimulation Models
Social Security Bulletin, Vol. 68, No. 1, 2008
Given immigration's recent resurgence as an important demographic fact in the U.S. economy, U.S. policy modelers are just beginning to grapple with how best to integrate immigrants into policy models. Building on the research reviewed in the first article of this series, this article puts forth a conceptual basis for incorporating immigration into a key type of policy model—microsimulation—with a focus on the projection of immigrant earnings.
The authors are with the Division of Economic Research, Office of Research, Evaluation, and Statistics, Office of Retirement and Disability Policy, Social Security Administration; Duleep is also a Research Professor with the Thomas Jefferson Program in Public Policy, College of William and Mary and a Research Fellow with IZA, Institute for the Study of Labor.
The findings and conclusions presented in the Bulletin are those of the authors and do not necessarily represent the views of the Social Security Administration.
Summary
Forecasts of the financial status of Social Security's Old-Age, Survivors, and Disability Insurance (OASDI) programs and forecasts of the effects of various OASDI policy options on Americans would be improved if information about the earnings and labor force behavior of various population subgroups were included in projection models. Focusing on the projection of immigrant earnings, this article proffers a conceptual basis for incorporating immigration into microsimulation models. Key results from research on immigrant earnings, as described in the first article in this trilogy—"Research on Immigrant Earnings"—are linked to methods for forecasting individual earnings in microsimulation models. The research on immigrant earnings also inspires new methods for forecasting earnings in microsimulation models as well as the projection of immigrant emigration. Forecasting immigrant earnings and emigration is discussed in the context of a "closed system"—that is, forecasts are only made for a given population, which is represented in the base sample of the microsimulation model. The third article in our trilogy—"Incorporating Immigrant Flows into Microsimulation Models"—explores how to project immigrant earnings in the context of an "open system," which includes future immigrants.
Introduction
With the end of World War II, but particularly since the 1960s, immigration to the United States increased dramatically. From 1941 through 1950, a million immigrants were issued permanent U.S. visas; for 1951–1960, 2.5 million; 1961–1970, 3.3 million; 1971–1980, 4.5 million, and for 1981–1990, 7.3 million.1 Over 9 million individuals immigrated in the 1990s rivaling in absolute (though not percentage) terms immigration in the 20th century's first 10 years, when a record number of immigrants entered the United States (U.S. Immigration and Naturalization Service (INS) 1998).2 Over the decade ending in 2005, foreign-born workers comprised more than half of the growth in the labor force (Congressional Budget Office 2005).
Models are used to project the financial status of programs and to project the effects of program changes. This can involve projecting key characteristics for a given population and may involve projecting future additions to that population. For the Old-Age, Survivors, and Disability Insurance (OASDI) programs, commonly known as Social Security, forecasts of the financial status involve projections of the contributions into and benefits from the system for the current population and those of the future.
Policy modelers are just beginning to grapple with how best to integrate immigration into their models. Once they have taken into account immigrant/native differences in demographic and human capital characteristics, modelers must decide whether to distinguish the labor force behavior of immigrants from that of seemingly similar U.S. natives and whether to differentially represent the earnings and work patterns of various immigrant groups. This article addresses these and other issues as it proffers a conceptual basis for incorporating immigrant earnings into a key type of policy model—microsimulation—with a focus on modeling the relationship between immigration and Social Security.
Social Security, Immigration, and Microsimulation
Each year the Social Security Administration (SSA) forecasts the financial status of Social Security. The explicit treatment of immigrants in the actuarial forecasts is purely demographic: The Office of the Chief Actuary (OCACT) projects net immigration as part of its projection of the population that contributes to and benefits from Social Security. OCACT also estimates trends in economic variables, such as how much people work and earn. The economic trends are estimated for the U.S. population that includes both natives and immigrants and are imposed upon the projected demographic trends. In general, there is no explicit treatment in the actuarial projections of potential differences between immigrants and natives in labor force variables such as earnings.3
Social Security benefits are based on lifetime earnings. Knowing more about how immigrant earnings trajectories compare with those of natives and how these trajectories vary across groups of immigrants would establish a more explicit basis for describing the economic impact immigrants have on the Social Security system. Ideally, modelers would predict contributions to and benefits from the Social Security system for the current immigrant population based on characteristics that capture distinctive features of their U.S. earnings trajectories. With continuously available data on these characteristics, the projections could be annually updated using information on each year's incoming immigrants. Such a procedure would supplement the long-range actuarial forecasts and more clearly illuminate the relationship of immigrants to the Social Security system.
Dynamic microsimulation models provide a vehicle for projecting the earnings of individuals and the concomitant impact of those earnings on contributions to and benefits from the Social Security system.4 Starting with data on individual characteristics such as age, years of schooling, and past work behavior for a representative sample of the population of interest, a microsimulation model forecasts the behavior of each individual in the sample. The simulations are based on the best information available concerning the relationship between the behavior in question (for example, earnings) and the selected predictors (for example, age, years of schooling, and past work behavior).
The simulations provide a snapshot of the current population's future. In this way, microsimulation makes it possible to incorporate distributional characteristics into the projection methodology and to produce distributional results.
To estimate aggregate values, such as population totals and averages, modelers can simply sum over the projected individual outcomes—one of several key advantages of microsimulation models. Most research on human behaviors relevant to Social Security—such as earnings, labor force behavior, disability and mortality, and disability—is done at the level of the individual. Moreover, the relationship between the explanatory variables (the determinants of the behaviors of interest) and the behaviors is nonlinear. A relationship is nonlinear if the effect of an explanatory variable on the variable of interest varies by the level of the explanatory variable or if its effect varies with the level of other explanatory variables. The relationship between income and mortality is an example of a nonlinear relationship. Changes in income have a very large effect on the probability of death for individuals at low levels of income and very small effects at high levels of income (Duleep 1986a).5 Moreover, income's effect on mortality is affected by other variables, such as marital status (Smith and Zick 1994; Zick and Smith 1991). Yet, despite the ubiquity of nonlinear relationships in models of human behavior, there is no known way of aggregating nonlinear relationships.6 The microsimulation approach—summing over individual outcomes to produce aggregate values of interest—provides a straightforward method of utilizing microanalytic research to project aggregate values of interest.
In addition to using microsimulation to predict Social Security contributions and benefit receipts of the current population, it also can help gauge the effects of proposed and actual policy changes on the financial status of the Social Security system (Burtless 1994; Social Security Administration 1995). The validity of such estimates rests on how accurately relevant behaviors of various population subgroups, such as immigrants, are modeled.
Incorporating Immigrant Earnings Research into Microsimulation Models
The preceding article's review of research on immigrant earnings patterns (Duleep and Dowhan 2008) highlights the following five findings with important implications for projecting immigrant earnings contributions to the Social Security system.
(1) Controlling for demographic and human capital characteristics, immigrants often start their U.S. lives with substantially lower earnings but experience faster earnings growth than natives with comparable years of schooling and experience. The lower immigrants' initial earnings are (relative to U.S. natives), the higher their subsequent earnings growth is. Thus the initial earnings of immigrants, relative to their U.S.-born statistical twins, provide valuable information about immigrant earning trajectories.
(2) The extent to which the earnings trajectories of immigrants and natives differ varies by country of origin, with the source country's level of economic development being the key determinant of the size of the U.S.-born/foreign-born difference. The earnings profiles of immigrants from economically developed countries such as Japan, Canada, or Western Europe resemble those of U.S. natives who are of the same age and education level. In contrast, the earnings of immigrants from developing nations tend to start well below those of U.S. natives with comparable years of schooling and experience, but rise more rapidly.
(3) Immigrant earnings profiles have changed over time. For both immigrant men and women, the earnings profiles of recent immigrants, particularly those who entered the United States in 1980 and afterwards, are characterized by low initial earnings and high earnings growth relative to statistically similar U.S. natives. Earlier immigrant cohorts, particularly those who entered the United States before 1970, have earnings profiles that resemble those of statistically similar U.S. natives. Compared with recent cohorts, earlier immigrant cohorts have high initial earnings and low earnings growth.
(4) Although immigrant earnings profiles have changed dramatically over time, the adjusted earnings profiles of post-1980 immigrant cohorts are remarkably similar.
(5) Holding age and years of schooling constant, a persistent pattern emerges over time and across groups: There is a strong inverse relationship between immigrant entry earnings and earnings growth.
The remainder of this article links these and other research results to various issues essential for incorporating immigrant earnings into microsimulation models. Beginning with the next section, we link key results from research on immigrant earnings, described in Duleep and Dowhan (2008), to extant methods for forecasting individual earnings in microsimulation models. Inspired by the research on immigrant earnings, we then propose new methods for forecasting individual earnings and then explore an often-overlooked phenomenon—some immigrants emigrate. Although illegal aliens, also known as undocumented immigrants, are represented to an unknown degree in many survey data sets, the penultimate section confronts the challenges of explicitly representing the undocumented in microsimulation models. The article concludes with a discussion about the choice of variables that can be used to predict immigrant earnings in microsimulation models.
The discussion proceeds in terms of a closed system. That is, we examine a system in which immigrant earnings (and emigration) are forecast for a given population that the base sample represents in the microsimulation model. The last article in the trilogy, which follows this one, addresses immigrant earnings projections for open systems—microsimulation models that include projections of future immigration.
Immigrant Earnings Research and Extant Methods for Forecasting Individual Earnings in Microsimulation Models
There are three general methods used to forecast individual earnings in microsimulation models: the "human capital" approach, the "past-is-prologue" approach, and the "donor" approach.7
The Human Capital Approach
The human capital approach to project earnings in microsimulation models estimates the relationship between individual earnings and demographic and human capital characteristics, most notably age and education. The estimated coefficients are applied to the characteristics of each individual in the model's base population sample to project his or her future earnings.
The earnings regressions that inform microsimulation models have typically been estimated across the adult population, not distinguishing between the foreign and native born. The research review of Duleep and Dowhan (2008) demonstrates that such earnings projections will misrepresent the earnings profiles of recent immigrants; even controlling for age and education, the earnings profiles of recent immigrants differ from those of the U.S. born. Nor will it suffice to estimate an earnings regression on a sample that pools the foreign and native born and include a categorical variable (also known as a dummy or zero-one variable) to identify the foreign born. Such a strategy would work if immigrant earnings profiles resembled those of natives, but were uniformly lower. Recent immigrants generally start at lower earnings than the U.S. born and experience higher earnings growth.
To project immigrant earnings accurately, the earnings regressions that inform microsimulation models must be estimated separately for the foreign and native born. An implication of the inverse relationship between immigrant entry earnings and earnings growth is that the earnings growth of different year-of-immigration entry cohorts needs to be separately estimated, as opposed to estimating a pooled model that captures cohort effects with a dummy variable for each entry cohort.8
Because the earnings profiles of immigrants have changed markedly over time, accurately projecting the earnings of recent immigrants requires modelers to use earnings regressions estimated on recent immigrant cohorts. The regressions that inform the earnings projections of recent immigrants could be done, for instance, on a sample limited to immigrants that entered the United States after 1979.
There are also important differences in earnings profiles among immigrants divided by country of origin that are associated with source countries' level of economic development. Immigrants from economically developed countries have earnings profiles that resemble those of U.S. natives with similar years of education and experience. Initially, the earnings of immigrants from economically developing countries are much lower than their U.S.-born statistical twins, but rise more steeply.
Ideally, separate earnings regressions for recent immigrants from each source country would be estimated to capture these differences. Because sample size constraints make this impractical, a more feasible approach would be to estimate separately the immigrant earnings regressions for eight source-region categories: (1) Eastern Europe; (2) Western Europe, Oceania, and Japan; (3) Asia (except Japan); (4) Africa; (5) Canada; (6) Mexico, (7) Caribbean; and (8) Central and South America (except Mexico). Immigrants within these categories share similar earnings profiles, controlling for age at immigration and education. If the eight categories are still too many, then modelers could use the following four categories: (1) Economically developed countries (except Canada), (2) Canada, (3) Economically less-developed countries (except Mexico), and (4) Mexico. This division captures the economically developed versus less developed divide and, with the separate treatment of Canada and Mexico, the added dimension of proximity to the United States. If four categories were still too many, then a broad, but informative division simply would be to divide the world into two groups consisting of (1) the economically developed countries, and (2) the less-developed countries.
The "Past-Is-Prologue" Approach
In a nod to Shakespeare, a second approach for forecasting individual earnings in microsimulation models is the "past-is-prologue" approach (Iams and Sandell 1997): Earnings in earlier years predict earnings in later years. Underlying this approach is the idea that an individual's past earnings behavior captures both measured and unmeasureable factors that affect earnings (Nakamura, Nakamura, and Duleep 1990; Duleep and Sanders 1994). Iams and Sandell find that once past behavior is included, human capital variables contribute little further predictive power. The past-is-prologue approach requires a data source that follows the earnings of individuals over time or a survey with retrospective questions about past earnings.
In estimating the relationship between past and current earnings, it is important (as with the human capital approach) to separate the foreign born from the native born. Projecting future earnings using the estimated relationship between past and present earnings based on a sample of U.S. natives (or a sample in which natives dominate) would understate the future earnings of most recent immigrants, because recent immigrants tend to have higher earnings growth than natives. Moreover, to forecast accurately the earnings of recent immigrants, the past/present earnings relationship needs to be estimated on a sample of recent immigrants, such as immigrants who came to the United States after 1979, as opposed to a sample that represents earlier immigrants, or a sample that represents all immigrants regardless of year of entry. As shown in the preceding article (Duleep and Dowhan 2008), the relationship between past and present earnings is flatter for earlier immigrants than it is for more recent immigrants.
Finally, it is important to estimate the past/present earnings relationship on samples that divide immigrants by region of origin. The relationship between past and present U.S. earnings for immigrants coming from economically developing countries is much steeper than that for immigrants coming from economically developed countries resembling the United States, and this is particularly true for recent immigrant cohorts (Duleep and Dowhan 2008).
The "Donor" Approach
A third approach for projecting earnings in microsimulation models might be labeled "the donor approach" or, more exotically, the "clone" approach. To project the future earnings of individuals, similar, but older, individuals are chosen to provide their earnings as forecasts for the individuals with incomplete earnings histories (Burtless, Sahn, and Berk 2002).
The donor approach may combine insights of both the human capital approach and the past-is-prologue approach. As in the human capital approach, evidence from "like" individuals is used to project the earnings of individuals, where the pool of potential donors is sometimes determined by characteristics, such as age and education, commonly included in earnings estimations. As in the past-is-prologue approach, one of the characteristics that may be used to define the pool of potential donors is the past earnings of individuals.
As applied in Social Security microsimulation efforts, donors who are 5 years older are chosen to provide their earnings in 5-year intervals as forecasts for the individuals with incomplete earnings histories. The donors are randomly chosen from a potential pool of individuals, determined by a set of variables for which a match must occur between the worker with the incomplete earnings history and the 5-year-senior potential donor. This requires that the donor's age during the matching period be identical to that of the target worker and that his or her earnings in the years up to and including the matching period be similar to those of the target worker.9
The immigrant earnings trajectories highlighted in the preceding article (Duleep and Dowhan 2008) suggest three lessons for the donor approach: (1) donors for immigrants should be immigrants, (2) donors chosen to project the earnings of recent immigrants should be recent immigrants, such as immigrants who came to the United States after 1979, and (3) donors for immigrants from economically developed (developing) countries should be immigrants from economically developed (developing) countries.
When Dividing by Nativity and Year of Entry is Less Critical
Regardless of whether modelers use the human capital, past-is-prologue, or donor approach to forecast earnings, the preceding article's review of research (Duleep and Dowhan 2008) provides guidance as to when dividing by foreign-born/native-born status is less critical in microsimulation models. Because the earnings trajectories of immigrants who entered the United States before 1970, for men, and before 1980, for women, resemble those of U.S. natives (Duleep and Dowhan 2008, Chart 4), it is less critical to divide by nativity for models focused on the earlier entrants. It is also less critical to divide by nativity for models focused on immigrants coming from economically developed countries than it is for models focused on immigrants from the economically developing world, or models focused on all recent immigrants, because immigrants from economically developed countries have earnings profiles that resemble those of U.S. natives of similar age and years of schooling. The inverse relationship between immigrant entry earnings and earnings growth means that, holding years of schooling and experience constant, the longer immigrants have been in the United States, the more their earnings approach those of similarly educated and experienced natives, regardless of their country of origin (Duleep and Regets 1994a, 1994b, 1997a, 2002; Duleep and Dowhan 2002, 2000). For microsimulation models, this finding implies that the importance of separately treating immigrants and natives, or groups of immigrants, wanes the longer immigrants have lived in the United States.
Depicting Immigrant Earnings Variation
In applying the research results on immigrant earnings to projecting immigrant earnings trajectories, one should be mindful of an important caveat. Research suggests that immigrants who come from economically developed countries have earnings profiles that resemble those of similarly schooled and experienced U.S. natives, whereas the earnings profiles of immigrants from economically developing countries are quite different. Yet not all immigrants from economically developed countries have earnings profiles resembling those of their U.S.-born statistical twins, and not all immigrants from economically developing countries have trajectories characterized by low initial earnings and high earnings growth. Rather, within any group of immigrants originating from the same source country, a range of earnings profiles exists, with the percent of immigrants with low initial earnings and high earnings growth being higher among immigrants from economically developing versus developed countries. How successfully a model captures variations in earnings profiles will affect how accurately it can illuminate distributional issues. Depicting the range of immigrant earnings profiles is a challenge for microsimulation modelers.
The extent to which the past-is-prologue approach to modeling earnings captures variability in immigrant earnings profiles depends on the degree to which the measures of past earnings behavior that are used for prediction capture this variability. The success of the human capital approach in this regard depends on the degree of detail embedded in the parameterized equation used to relate the explanatory variables to earnings. Given sufficient sample size in the model's base population sample, the donor approach to modeling earnings in microsimulation models will, by design, be the most successful in representing variation in immigrant earnings profiles. This is because the individuals who "donate" the projected earnings profiles come from the existing population of immigrants and thus represent, in a completely nonparametric fashion, the extant variation in earnings profiles present within any demographic/human capital subgroup.
Immigration Research as a Catalyst for New Methods of Forecasting Earnings
The review of immigrant earnings research in the preceding article (Duleep and Dowhan 2008) also suggests new methods (or at least nuances in existing methods) for projecting earnings in microsimulation models.
The Predictive Power of Earnings Growth
A theme of the immigrant earnings research discussed in Duleep and Dowhan (2008) is the predictive power of earnings growth. Higher earnings growth distinguishes the earnings trajectories of immigrants from those of natives. It also distinguishes the earnings trajectories of immigrants who come from economically developing versus economically developed countries. Indeed, conditional on age and education, a few years of earnings growth may suffice to successfully identify in microsimulation models the earnings trajectories of the foreign born versus the native born as well as earnings variability within the foreign born.
An empirical test of this idea was a by-product of recent efforts to include immigration in Social Security's Modeling Income in the Near Term (MINT) microsimulation model. In one version of this model, donors are used to project earnings of workers up to age 55. Worker and donor must match on a set of variables that includes sex, education, disability status, race, ethnicity, and several earnings variables measured over a 5-year matching period (initially 1994–1998). The earnings variables include the number of years in which there are earnings in the 5-year matching period, average earnings for the 5-year matching period, earnings in the matching period's fifth year, earnings in the matching period's fourth year, and average earnings before the 5-year matching period.
The earnings variables are particularly important for the topic at hand because, combined, they provide information on earnings growth. If earnings growth (along with the standard demographic and human capital variables) suffices to accurately project immigrant earnings, then it should not be necessary to separately treat the foreign and native born in models that incorporate these variables in the projection methodology—a prediction that has proved correct.
When the MINT modelers included immigrant status as a matching constraint to ensure that immigrants from later cohorts received earnings from immigrants from earlier cohorts, little change occurred in the model's projected distribution of earnings.10 The result suggests that a matching algorithm that includes earnings growth, along with the usual demographic/socioeconomic variables, may accurately project immigrant earnings. It also suggests that modelers may not need to divide immigrants by region of origin, or by their year of U.S. entry, or even to separate the foreign born from the native born as long as earnings growth is in the predictive model—a finding of particular import when the size of the microsimulation base population sample is constrained.
The Predictive Power of Immigrant Entry Earnings
When incorporating immigrant earnings into microsimulation models, a challenge is how to project earnings trajectories for recently arrived immigrants who lack a U.S. earnings history. The immigrant earnings research in Duleep and Dowhan (2008) provides a potential solution.
The initial earnings of immigrants, relative to the earnings of U.S. natives of similar age and education, impart information about immigrants' future earnings—the lower (higher) the relative entry earnings of immigrants, controlling for age and education, the higher (lower) the subsequent earnings growth. This finding suggests that modelers could use the distance between the initial earnings of immigrants and that of similarly educated and experienced natives as a matching variable for selecting immigrant donors from earlier cohorts for the recently arrived immigrants. New immigrants would be assigned the earnings trajectories of earlier immigrants who had the same relative earnings starting point in the United States.
Alternatively, the relationship between immigrants' initial earnings, relative to similar U.S. natives, and subsequent earnings growth could be estimated and used to project the earnings trajectories of recently arrived immigrants. Modelers could amend the human capital approach for forecasting future immigrant earnings by adding as an explanatory variable the gap between immigrants' initial earnings and the earnings of U.S. natives with similar human capital attributes.
Not All Immigrants Stay: Predicting Immigrant Emigration
An often-ignored reality of immigration is that not all immigrants stay. When modeling immigrant earnings profiles (and benefit receipt) in microsimulation models, immigrant emigration requires special attention. It means that the U.S. earnings trajectories of some immigrants will be truncated. It is also likely that the propensity to emigrate and the "shape" of immigrant earnings trajectories (how high initial earnings are, how high earnings growth is) are related. The relationship between immigrant emigration and earnings profiles will affect how immigrants contribute to and benefit from Social Security.
A theme in the research review of Duleep and Dowhan (2008) is that immigrants who lack skills that transfer quickly to the U.S. labor market are more likely to invest in human capital. It follows that immigrants who lack transferable skills would be more likely to stay permanently in the U.S. than immigrants with highly transferable skills. Why invest if the rewards of the investment cannot be reaped? Indeed, it seems likely that immigrants who decide to come to the United States with the idea of investing in human capital would, from the outset, be more likely to see the country as their permanent home than would immigrants with highly transferable skills who do not intend to invest in U.S.-specific human capital.
In the absence of programs that recruit workers to fill specific labor market needs, immigrants from economically developing countries would tend to have lower U.S. skill transferability than immigrants from regions of the world with levels of economic development comparable to the United States. Following immigrant cohorts across decennial censuses, Duleep and Regets (2002) show that immigrants originating from economically developing countries have lower initial earnings, but higher earnings growth than those originating from economically developed countries. The inverse relationship between skill transferability and immigrants' propensity to invest in human capital suggests that immigrants from less economically developed countries would be more permanent than immigrants from countries similar to the U.S.
To test this hypothesis, we used 1980 and 1990 census data—the 5 percent public-use microdata sample (PUMS) files —to estimate 10-year attrition rates for immigrant cohorts who entered the United States during the 1975–1980 period, divided by age, sex, and economic-development status of the source country. Specifically, the number of immigrants who reported immigration to the United States from 1975 through 1980 was counted in the 1980 census and in the 1990 census. The 10-year attrition rates were then adjusted by the estimated 1980–1990 mortality of the cohorts. Of the proportion that was missing in the 1990 census, the percent that was estimated to have died was subtracted by applying the 1998 U.S. life tables by sex and single year of age to the age/sex/economic-development cohorts.11 The remaining attrition is attributed to emigration.
In estimating the mortality of immigrants, one could argue that race/ethnicity-specific mortality information should be applied to the attrition rates. Recent studies, however, hint that immigrants face lower mortality rates than their U.S.-born racial/ethnic counterparts.12 For this reason, and in the absence of actual information on immigrant mortality, the U.S. sex- and age-specific national statistics were used to adjust our immigrant attrition rates for mortality.
The resulting mortality-adjusted, 10-year attrition rates represent estimates of immigrant emigration, as shown in Table 1. As theoretically anticipated, the emigration rates of immigrants from less economically developed countries are lower than those of immigrants from more economically developed countries, particularly at younger ages when the propensity to invest in human capital is greatest.
Age group | 1980 to 1990 raw attrition rate a |
10-year mortality rate from sex- and age-specific mortality applied to individual 1980 data |
Residual emigration rate | |||
---|---|---|---|---|---|---|
Men | Women | Men | Women | Men | Women | |
Developed countries | ||||||
15–39 | 0.3507 | 0.3292 | 0.0185 | 0.0089 | 0.3322 | 0.3203 |
40–56 | 0.3518 | 0.2590 | 0.0650 | 0.0403 | 0.2868 | 0.2186 |
57–69 | 0.4704 | 0.4592 | 0.2308 | 0.1568 | 0.2396 | 0.3023 |
Developing countries | ||||||
15–39 | 0.0937 | 0.0609 | 0.0170 | 0.0080 | 0.0767 | 0.0529 |
40–56 | 0.1677 | 0.1062 | 0.0658 | 0.0415 | 0.1019 | 0.0646 |
57–69 | 0.3565 | 0.2803 | 0.2325 | 0.1534 | 0.1241 | 0.1269 |
SOURCE: The mortality data are from Table 2. Life table for males: United States, and Table 3. Life table for females: United States, Public Health Service, National Vital Statistics Report, Vol. 48, No. 18 (February 7, 2001). | ||||||
NOTE: PUMS = public-use microdata sample. | ||||||
a. The raw attrition rate is defined as [(the number of immigrants in the 1980 5 percent PUMS who entered the United States during the 1975–1980 period) - (the number of immigrants in the 1990 5 percent PUMS who entered the United States during the 1975–1980 period] / [(the number of immigrants in the 1980 5 percent PUMS who entered the United States during the 1975–1980 period)]. This statistic is computed for age, sex, and economic development categories, wherein the age category is aged 10 years when counting the number of immigrants in the 1990 PUMS. |
The emigration estimates shown in Table 1, based on two points in time separated by 10 years, do not convey the year-to-year pattern of emigration in the years before 10 years, or the emigration that occurs after 10 years. This information may be needed to model immigrant emigration in microsimulation models.
Research based on 1908–1950 U.S. emigration data (Warren and Kraly 1985) and research using 1971–1976 Social Security administrative data on retired immigrant emigrants (Duleep 1994) coupled with theoretical considerations suggest that the propensity of immigrants to emigrate declines the more time they spend in the United States. This pattern can be expressed as the exponential decay function y = d + ae-bx
where:
y = the number of immigrants in the United States after x years,
d = the number of immigrants who will never emigrate,
a = the total number of people who will emigrate, and
x = the number of years.
Furthermore, various pieces of empirical research, when tied together, suggest that about 87 percent of all emigration occurs within the first 10 years following immigration (Duleep 1994). Combining this information with the estimated 10-year emigration rates and the exponential decay model, estimates are generated of the percent of each age/sex/source-country cohort that emigrates for each year following immigration (Chart 1).13
Immigrants remaining in the United States, by years since immigration, entry age group, and source-country development status
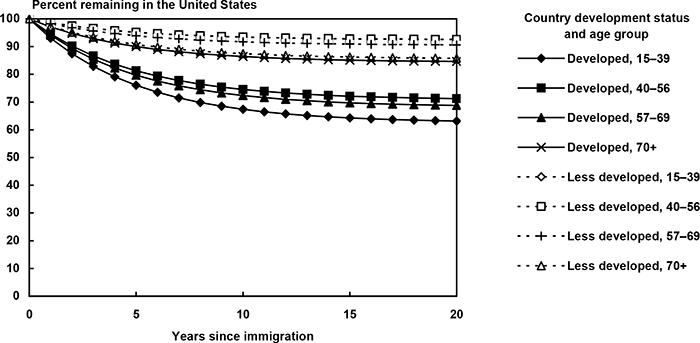
Years since immigration |
Developed countries, 15–39 |
Developed countries, 40–56 |
Developed countries, 57–69 |
Developed countries, 70+ |
Less developed countries, 15–39 |
Less developed countries, 40–56 |
Less developed countries, 57–69 |
Less developed countries, 70+ |
---|---|---|---|---|---|---|---|---|
1 | 93.08133415 | 94.6019857 | 94.1380563 | 97.11810836 | 98.60864423 | 98.60864423 | 98.24216433 | 97.33258573 |
2 | 87.43954707 | 90.20020537 | 89.35796735 | 94.76808606 | 97.47407102 | 97.47407102 | 96.80874714 | 95.1574578 |
3 | 82.8389836 | 86.61079841 | 85.46007076 | 92.85177362 | 96.54888968 | 96.54888968 | 95.63987517 | 93.38376198 |
4 | 79.08748005 | 83.68383673 | 82.28155295 | 91.28912744 | 95.79445578 | 95.79445578 | 94.68672512 | 91.93741173 |
5 | 76.02833762 | 81.29706233 | 79.68964852 | 90.01487643 | 95.1792569 | 95.1792569 | 93.90948427 | 90.75799357 |
6 | 73.53377717 | 79.3507806 | 77.57609466 | 88.97579562 | 94.67759644 | 94.67759644 | 93.27568761 | 89.79624367 |
7 | 71.49960192 | 77.76369613 | 75.85260908 | 88.12848303 | 94.26852025 | 94.26852025 | 92.75886169 | 89.01199014 |
8 | 69.84084517 | 76.46951704 | 74.44720248 | 87.43754675 | 93.93494138 | 93.93494138 | 92.33741891 | 88.37247502 |
9 | 68.48822132 | 75.414186 | 73.30117159 | 86.87412666 | 93.66292637 | 93.66292637 | 91.99375577 | 87.85098602 |
10 | 67.38523184 | 74.55362225 | 72.36664715 | 86.41468892 | 93.44111326 | 93.44111326 | 91.7135176 | 87.42574076 |
11 | 66.48580528 | 73.85188039 | 71.60459447 | 86.04004301 | 93.26023701 | 93.26023701 | 91.48499896 | 87.0789769 |
12 | 65.75237296 | 73.27964895 | 70.98318291 | 85.73454011 | 93.11274249 | 93.11274249 | 91.29865473 | 86.79621027 |
13 | 65.1542997 | 72.81302604 | 70.47645635 | 85.4854195 | 92.99246891 | 92.99246891 | 91.14670137 | 86.56562981 |
14 | 64.66660424 | 72.43252103 | 70.06324904 | 85.28227549 | 92.89439251 | 92.89439251 | 91.02279186 | 86.37760428 |
15 | 64.26891573 | 72.12224035 | 69.72630146 | 85.11662286 | 92.81441665 | 92.81441665 | 90.92175056 | 86.22427992 |
16 | 63.94462287 | 71.86922373 | 69.45153946 | 84.98154236 | 92.74920079 | 92.74920079 | 90.83935699 | 86.09925243 |
17 | 63.68018009 | 71.66290275 | 69.22748634 | 84.87139172 | 92.69602089 | 92.69602089 | 90.77216962 | 85.99729948 |
18 | 63.46454171 | 71.49465947 | 69.04478348 | 84.78157 | 92.65265564 | 92.65265564 | 90.71738206 | 85.91416251 |
19 | 63.28870061 | 71.35746643 | 68.89579946 | 84.70832539 | 92.61729368 | 92.61729368 | 90.67270585 | 85.84636894 |
20 | 63.14531198 | 71.24559314 | 68.77431128 | 84.64859848 | 92.58845798 | 92.58845798 | 90.63627489 | 85.79108705 |
These estimates can be applied to each year-of-immigration cohort of immigrants in the base sample of microsimulation models. From the base sample of immigrants in the model, emigrants can be chosen according to the probabilities of leaving, defined by sex, age, years in the United States, and country of origin (whether economically developed or not).14
Giving Undocumented Immigrants Earnings Profiles in Microsimulation Models
Although many data sources include to an unknown degree illegal immigrants, the discussion of projection methodology thus far has not explicitly treated illegal immigration. A challenge with incorporating the undocumented into microsimulation models is how to impute their earnings trajectories.
One approach would be to identify undocumented immigrants within the model's base population.15 An imputation process originally developed at the Urban Institute by Jeff Passel and Rebecca Clark uses a two-part process to code survey respondents as undocumented aliens versus legal immigrants.16
Individuals are first identified as legal immigrants if they have characteristics that would make it very unlikely for them to be undocumented. For instance, individuals are classified as legal immigrants if they are in certain occupations that rarely are pursued by the undocumented, or if they receive benefits for which the undocumented are ineligible, or if they are veterans. Then, using the occupational structure of former illegal aliens who legalized under the Immigration Reform and Control Act of 1986 (IRCA), the percentage of aliens in each major occupation category in the Current Population Survey (CPS) that is undocumented is estimated.17 Within each state/sex/occupation group, individuals are then randomly assigned to be undocumented or legal aliens, in line with the estimates of what percent in each of these cells should be undocumented. Equipped with these imputations, the earnings information of the assigned illegal aliens could be used to project the earnings trajectories of undocumented immigrants.
A potential problem with this approach is that the undocumented who are in national surveys such as the CPS may not represent most undocumented individuals. In particular, to the extent that undocumented people in the CPS and other national surveys are more permanent than those who are not in these surveys, their earnings patterns will be different as well. It seems unlikely that national surveys would "capture" undocumented immigrants who transit back and forth between the United Sates and their home country, which, for many, is Mexico. The intermittent U.S. attachment of these individuals makes it difficult to follow them through the Social Security record system or with any longitudinal survey data. Learning about the U.S. earnings profiles of these individuals requires an entirely different approach.
Moreover, assignment of illegal status in the Clark/Passel method is random. This is not a problem when projecting the numbers of illegal immigrants who, for instance, live in a particular region of the United States. It is however, a problem if the earnings profiles of the tagged individuals are used to represent the earnings profiles of illegal aliens. The earnings patterns of some of the respondents who are tagged undocumented will in fact be legal immigrants. Yet, we would anticipate very different earnings profiles for legal immigrants versus the undocumented, particularly undocumented people who transit back and forth and do not intend to stay in the United States permanently.
The imputation approach also fails to recognize that within the undocumented immigrant population there are two types of immigrants—those who transit back and forth from their country of origin and those who plan to stay. Because the "stayers" will be more likely to invest in human capital than the transient, their earnings profiles will be characterized by lower initial earnings but higher earnings growth than the earnings profiles of the more transient population. A more sophisticated approach would also recognize a transition for some of the undocumented immigrants from being transient to more permanent. The earnings profiles of those who become legal or plan to become legal will differ from the earnings profiles of the undocumented who traverse back and forth. An alternative strategy would be to estimate the percent of the undocumented who are transient versus stayers and then impute earnings trajectories according to research that focuses specifically on those two "types" of undocumented immigrants.18
The Mexican Migration Project (MMP) is designed to capture the experiences of the more elusive group, those who transit back and forth. Created in 1982, the MMP attempts to garner social as well as economic information on Mexican-U.S. migration. Employing comprehensive intensive studies of Mexican communities, data are gathered in the winter months, when many migrants return home to join their families. Out-migrant samples are also taken, matching communities with migrants residing in the United States. The collected data have been compiled in a comprehensive database that has formed the foundation of numerous studies such as Massey (1987), Massey and Singer (1995), Orrenius and Zavodny (2001), Phillips and Massey (1999), Singer and Massey (1998), Donato and Massey (1992), and White, Bean, and Espenshade (1990).
The second "type" of undocumented immigrant falls between the permanent visa holders documented in the Office of Immigration Statistics (OIS) of the Department of Homeland Security (formerly known as the Immigration and Naturalization Service) and the temporary sojourners described in the Mexican Migration Project. They are illegal entrants (either by virtue of entering the United States illegally or by overstaying their visa) who end up staying permanently. Some insight about these foreign-born individuals comes from the Legalized Population Surveys (LPS) of 1989 and 1992. Under the Immigrant Reform and Control Act of 1986, 3 million previously unauthorized foreign-born individuals residing in the United States were legalized. The IRCA amnesty restrictions applied only to persons who exhibited some measure of U.S. permanence. Beginning in 1987, those who had resided continuously in the United States since January of 1982 could apply for permanent resident status under the amnesty provisions of IRCA. The Legalized Population Surveys of 1989 and 1992 are longitudinal data that follow formerly illegal immigrants who were legalized under the 1986 Immigrant Reform and Control Act. Studies that have employed these data include Cobb-Clark and Kossoudji (1999), Kossoudji and Cobb-Clark (2000, 2002), Powers and Seltzer (1998), Powers, Seltzer, and Shi (1998), and Rivera-Batiz (1999).
In deciding how to represent the earnings trajectories of the undocumented, modelers need to think about whether both types of illegal aliens are relevant to the issues they are pursuing. It may be that only the transient type or the stayer type are relevant for their purposes. What currently matters for Social Security purposes is not an accurate representation of the earnings trajectories of the undocumented, or even how many undocumented enter the United States each year, but rather an accurate representation of the Social Security earnings contributions from the undocumented sector. For this purpose, Social Security's Earnings Suspense File, adjusted for employer reporting error or individuals' name changes, might be the best source of information. On the other hand, if one wants to estimate the effect on the Social Security system of legalizing the undocumented, then an accurate representation of the earnings trajectories of the undocumented is important, keeping in mind that by affecting employment opportunities and permanence, legalization would affect the earning trajectories of those who were legalized.
As with the legal population, the emigration behavior of the undocumented population would need to be incorporated into the model. The evidence to date suggests an emigration pattern that is distinct from that of the legal population. Based on an analysis of the 1995 CPS, Passel (1999) finds that only 25 percent of the undocumented immigrant population had been in the United States for 10 years. Representing the emigration of the undocumented population with the exponential decay function introduced in the preceding section and imposing Passel's estimate that 75 percent of any given cohort of undocumented immigrants emigrate before 10 years yields a much higher emigration for the undocumented population than the documented, and a much steeper decline in the probability of emigrating with time spent in the United States (Chart 2).
Undocumented immigrants remaining in the United States, by years since immigration
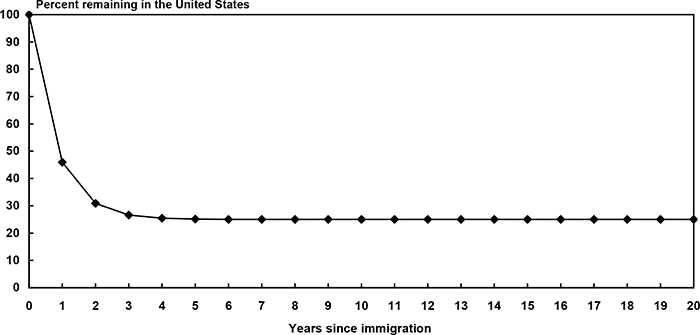
Years since immigration |
Percent remaining in the United States |
---|---|
0 | 100 |
1 | 45.96153846 |
2 | 30.85848126 |
3 | 26.6373704 |
4 | 25.45762404 |
5 | 25.12790005 |
6 | 25.03574642 |
7 | 25.00999067 |
8 | 25.00279226 |
9 | 25.0007804 |
10 | 25.00021811 |
11 | 25.00006096 |
12 | 25.00001704 |
13 | 25.00000476 |
14 | 25.00000133 |
15 | 25.00000037 |
16 | 25.0000001 |
17 | 25.00000003 |
18 | 25.00000001 |
19 | 25 |
20 | 25 |
Before deciding how to represent the undocumented immigrant population in a microsimulation model, modelers should carefully consider whether the issues being addressed require the inclusion of the undocumented at all. The youthfulness of the undocumented (described in the next article) and their generally short U.S. stays may make their inclusion irrelevant for some of the policy issues microsimulation models address.
A Concluding Word about the Choice of Predictor Variables
An important advance since microsimulation was conceived and the first model built (Orcutt 1957; Orcutt, Greenberg, Korbel, and Rivlin 1961) is the creation and use of longitudinal earnings data for research. This made possible the development of the less restrictive donor and past-is-prologue approaches for projecting individual earnings in microsimulation models. Yet, surveys that follow individuals or surveys matched to longitudinal administrative data are typically small. Because immigrants are a subsample of the population, sample size will often dictate a parsimonious choice of variables for projecting immigrant earnings trajectories in microsimulation models.
Ideally, an ongoing process would be established that would predict contributions to and benefits from the Social Security system for the immigrant population based on immigrant characteristics that capture distinctive features of immigrant earnings trajectories. Data on the predictor variables should be readily available on a continual basis so that projections can be updated annually.
Age at migration, sex, source-country level of economic development, and entry-level education determine distinct immigrant earnings trajectories. Other variables such as entry-level English language proficiency could be considered also. However, as described in the article preceding this one (Duleep and Dowhan 2008), there are complex interactions that modelers would then need to consider to insure a robust projection of immigrant earnings trajectories.
Building on research that links the characteristics of immigrants measured during their initial years in the United States to subsequent earnings growth, this article has emphasized the use of entry-level characteristics (for example, entry-level education) to predict immigrant earnings trajectories. The reason for this emphasis is twofold. One, it obviates what would otherwise be a need to model human capital investment processes.19 Two, an approach that relies on entry-level predictors lends itself to projections of future immigration—the topic of the next and final article in this series.
Notes
1. Refer to U.S. Immigration and Naturalization Service (1996, 2001). INS statistics on permanent visas show particularly dramatic increases in immigration in the 1980s. However, a large component of this increase represents newly legalized immigrants under the Immigration Reform and Control Act of 1986, as well as increases in adjustments from temporary to permanent visa status.
2. Immigrants entering the United States between 1900–1910 totaled 8.8 million, representing nearly 12 percent of the total U.S. population in 1900 (U.S. Immigration and Naturalization Statistics 1990, p. 22). The 1990 INS Statistical Yearbook presents an interesting synopsis of historical immigration trends. Also, see Reimers (1996). Note that the INS is now called the Office of Immigration Statistics, Department of Homeland Security.
3. An exception to this generalization is that a different labor force participation rate is assumed for undocumented immigrants than for the general population.
4. The seminal publications that introduced microsimulation are Orcutt (1957, 1960). Other publications that present this modeling methodology include Orcutt, Caldwell, and Wertheimer (1976), and Citro and Hanushek (1991).
5. These results hold controlling for past health problems that could affect the level of income being related to mortality (Duleep 1986a, 1986c).
6. For an illustration of the aggregation problem applied to the income-mortality relationship, refer to Duleep (1986b, 1986c).
7. Although the discussion presents these methods separately, modelers often use various combinations of them.
8. Because cohorts that vary in their entry-level earnings will also systematically vary in their earnings growth, the popular approach of controlling for cohort effects by including a dummy variable for each cohort in analyses that pool more than one cross-section is invalid: Earnings growth will be overestimated for cohorts starting at relatively high levels and underestimated for cohorts starting at relatively low levels. Predictions of immigrant earnings growth must either directly take into account the inverse relationship between entry earnings and earnings growth or include variables such as immigrant admission criteria, that may capture the effect of cohort characteristics on entry earnings and earnings growth, and allow the interaction between the added variables and the entry earnings and earnings growth (Duleep and Regets 1992, 1996a, 1996b). If modelers require information that summarizes the experiences of multiple year-of-entry cohorts, they can do this by averaging the estimates or modeling the relationship between entry earnings and earnings growth.
9. In the Social Security application, an iterative earnings splicing procedure was pursued instead of using the entire completed earnings record of a single donor: Successive imputations in 5-year time segments were used to forecast earnings until retirement. Different donors, from different older cohorts, provided the earnings information that was successively spliced to the end of each incomplete earnings record. After each 5-year imputation, another donor is chosen. The iterative splicing process continues until the worker reaches age 67 or is predicted to die.
10. In doing this sensitivity test, modelers should remember to use only post-1980 immigrants as donors for recent immigrants. If pre-1980 immigrant cohorts supply the donors, then "the test" will spuriously suggest no difference between using immigrants versus natives as donors; this would be because the earnings patterns of pre-1980 immigrants resembled the earnings patterns of U.S. natives. It could still be the case that using post-1980 immigrant cohorts as opposed to natives would make a difference.
11. The mortality data are from the Public Health Service (2001, Table 2. Life table for males, United States, and Table 3. Life table for females, United States). Refer to Ahmed and Robinson (1994) for a more sophisticated example of a census-based estimation of emigration.
12. Refer to Abraido-Lanza and others (1999); Fang, Madhavan, and Alderman (1997); Hummer and others (2000); Leclere (1997); Pandey and others (2001); Rogers and others (1996); Sorlie and others (1993); and Stellman and Wang (1994).
13. We ignore complexities that prior research hints at. For instance, we ignore spikes in emigration when eligibility for Social Security insurance occurs, and at retirement age (Duleep 1994).
14. Proximity of the United States to the country of origin should also be considered, particularly the neighboring status of Canada and Mexico.
15. Note that survey data matched by identifying characteristics to Social Security earnings data will more likely represent legal immigrants only.
16. This imputation of legal status for immigrants was first introduced in Passel and Clark (1998). It has subsequently been updated and expanded in many applications. See, for instance, Clark and others (2000) and Passel, Van Hook, and Bean (2006).
17. The Current Population Survey is a survey of approximately 50,000 households per month. It is used to calculate the monthly national unemployment rate and is one of the mainstays of demographic and labor market research. In January 1994, the CPS regularly began to collect information on nativity that permits the identification of immigrants.
18. Note that embedded in this approach could be a model of transformation from transient to stayer status for some undocumented immigrants.
19. As shown in Duleep and Regets (1999), immigrants are more likely to invest in education than natives are, and this tendency persists at older ages.
References
Abraido-Lanza, Ana F., Bruce P. Dohrenwend, Daisy Ng-Mak, and J. B. Turner. 1999. The Latino mortality paradox: A test of the salmon bias and health migrant hypotheses. American Journal of Public Health 89(10): 1543–1548.
Ahmed, Bashir, and Gregory Robinson. 1994. Estimates of emigration of the foreign-born population: 1980–1990. Population Estimates and Projections Technical Working Paper Series, No. 9. U.S. Census Bureau.
Burtless, Gary A. 1994. Framework for analyzing future retirement income security. Paper prepared for the Retirement Income Modeling conference sponsored by the National Research Council (September).
Burtless, Gary, Claudia Sahn, and Jillian Berk. 2002. Chapter 2: Projections of earnings and prevalence of disability entitlement. In Modeling in the near term. The Urban Institute (June).
Citro, Constance, and Eric Hanushek, eds. 1991. Improving information for social policy decisions: The uses of microsimulation modeling. Washington, DC: National Academy Press.
Clark, Rebecca L., Linda Giannarelli, Sandi Nelson, Jeffrey Passel, and Laura Wheaton. 2000. TRIM3 estimates of immigrant participation in public assistance programs: 1995 and 1997. Report to the Assistance Secretary for Planning and Evaluation, Department of Health and Human Services. Washington, DC: Urban Institute (December).
Cobb-Clark, Deborah, and Sherri Kossoudji. 1999. Did legalization matter for women? Amnesty and the wage determinants of formerly unauthorized Latina workers. Gender Issues (Fall): 3–14.
Congressional Budget Office. 2005. The role of immigrants in the U.S. labor market. Washington, DC: CBO (November). http://digitalcommons.ilr.cornell.edu/key_workplace/269/.
Donato, Katharine M., Jorge Durand, and Douglas S. Massey. 1992. Changing conditions in the U.S. labor market: Effects of the Immigrant Reform and Control Act of 1986. Population Research and Policy Review 11(2): 93–115.
Duleep, Harriet Orcutt. 1986a. Measuring the effect of income on adult mortality using longitudinal administrative record data. The Journal of Human Resources 21(2, Spring): 238–251.
———. 1986b. Incorporating longitudinal aspects into mortality research. Journal of Economic and Social Measurement 14(2): 121–134.
———. 1986c. Poverty and inequality in mortality. Doctoral dissertation, Department of Economics. Cambridge, MA: M.I.T.
———. 1994. Social Security and the emigration of immigrants. Social Security Bulletin 57(1): 37–52.
Duleep, Harriet, and Daniel J. Dowhan. 2000. Revisiting the family investment model with longitudinal data: The earnings growth of immigrant and U.S.-Born women. Paper presented at the 2000 Annual meeting of the Population Association of America.
———. 2002. Insights from longitudinal data on the earnings growth of U.S. foreign-born men. Demography 39(3, August): 485–506.
———. 2008. Research on immigrant earnings. Social Security Bulletin 68(1): 31–50.
Duleep, Harriet, and Mark Regets. 1992. Some evidence on the effect of admission criteria on immigrant assimilation. In Immigration, language and ethnic issues: Canada and the United States, Barry Chiswick, ed. 410–439. Washington, DC: American Enterprise Institute.
———. 1994a. The elusive concept of immigrant quality. Program for Research on Immigration Policy, Discussion Paper PRIP-UI-28. Washington, DC: The Urban Institute.
———. 1994b. Country of origin and immigrant earnings. Program for Research on Immigration Policy, Discussion Paper PRIP-UI-31. Washington, DC: The Urban Institute.
———. 1996a. Family unification, siblings, and skills. In Immigrants and Immigration Policy: Individual Skills, Family Ties, and Group Identities, Harriet Orcutt Duleep and Phanindra V. Wunnava, eds., 219–244. Greenwich, CT: JAI Press.
———. 1996b. Admission criteria and immigrant earnings profiles. International Migration Review 30(2, Summer): 571–590.
———. 1997a. Measuring immigrant wage growth using matched CPS files. Demography 34(2): 239–249.
———. 1997b. The decline in immigrant entry earnings: Less transferable skills or lower ability? Quarterly Review of Economics and Finance 37(Part A—special issue on immigration): 189–208.
———.1999. Immigrants and human capital investment. American Economic Review 89(2, May): 186–191.
———. 2002. The elusive concept of immigrant quality: Evidence from 1970–1990. IZA Discussion Paper, No. 631. Institute for the Study of Labor (November).
Duleep, Harriet, and Seth Sanders. 1994. Empirical regularities across cultures: The effect of children on women's work. Journal of Human Resources 29 (2, Spring—special issue on women's work, wages, and well-being): 328–347.
Fang, Jing, Shantha Madhavan, and Michael Alderman. 1997. Nativity, race, and mortality: Favorable impact of birth outside the United States on mortality in New York City. Human Biology 69(5): 689–701.
Hummer, Robert A., Richard Rogers, Sarit H. Amir, Douglas Forbes, and W. Parker Frisbie. 2000. Adult mortality differentials among Hispanic subgroups and non-Hispanic whites. Social Science Quarterly 81(1): 459–476.
Iams, Howard M., and Steven H. Sandell. 1997. Projecting Social Security earnings: Past is prologue. Social Security Bulletin 60(2): 3–16.
Kossoudji, Sherrie, and Deborah Cobb-Clark. 2000. IRCA's impact on the occupational concentration and mobility of newly-legalized Mexican men. Journal of Population Economics 13(1): 81–98.
———. 2002. Coming out of the shadows: Learning about the legal status and wages from the legalized population. Journal of Labor Economics 20(3, July): 598–628.
Leclere, Felicia B. 1997. Ethnicity and mortality in the United States: Individual and community correlates. Social Forces 76(1): 169–198.
Massey, Douglas S. 1987. Do undocumented migrants earn lower wages than legal immigrants? New evidence from Mexico. International Migration Review 21(2): 236–274.
Massey, Douglas S., and Audrey Singer. 1995. New estimates of undocumented Mexican migration and the probability of apprehension. Demography 32(2): 203–213.
Nakamura, Alice, Masao Nakamura, and Harriet Orcutt Duleep. 1990. Alternative approaches to model choice. Journal of Economic Behavior and Organization 14(1, September): 97–125.
Orcutt, Guy. 1957. A new type of socio-economic system. Review of Economics and Statistics 58(2), 773–797.
———. 1960. Simulation of economic systems. American Economic Review 50 (December).
Orcutt, Guy, Steven Caldwell, and Richard Wertheimer III. 1976. Policy exploration through microanalytic simulation. Washington, DC: The Urban Institute.
Orcutt, Guy, Martin Greenberg, John Korbel, and Alice Rivlin. 1961. Microanalysis of socioeconomic systems: A simulation study. New York, NY: Harper and Row.
Orrenius, Pia, and Madeline Zavodny. 2001. Self-selection among undocumented immigrants from Mexico. Research Department Working Paper 00005. Federal Reserve Bank of Dallas.
Pandey, Dilip K., Darwin R Labarthe, David C. Goff, W. Chan, and Milton Z. Nichaman. 2001. Community-wide coronary heart disease mortality in Mexican Americans equals or exceeds that in non-Hispanic whites: The Corpus Christi heart project. American Journal of Medicine 110(2): 81–87.
Passel, Jeffrey S. 1999. Undocumented immigration to the United States: Numbers, trends, and characteristics. In Illegal immigration in America, David W. Haines and Karen E. Rosenblum, eds., 27–111. Westport, CT: Greenwood Press.
Passel, Jeffrey S., and Rebecca L. Clark. 1998. Immigrants in New York: Their legal status, incomes, and taxes. Washington, DC: The Urban Institute.
Passel, Jeffrey, Jennifer Van Hook, and Frank D. Bean. 2006. Narrative profile with adjoining tables of unauthorized migrants and other immigrants, based on Census 2000: Characteristics and methods. Unpublished paper (February 15).
Phillips, Julie, and Douglas Massey. 1999. The new labor market: Immigrants and wages after IRCA. Demography 36(2): 233–246.
Powers, Mary G., and William Seltzer. 1998. Occupational status and mobility among undocumented immigrants by gender. International Migration Review 32(1): 21–56.
Powers, Mary G., William Seltzer, and Jing Shi. 1998. Gender differences in the occupational status of undocumented immigrants in the United States: Experience before and after legalization. International Migration Review 32(4): 1015–1041.
Public Health Service. 2001. National vital statistics report 48(18, February 7).
Reimers, David M. 1996. Third world immigration to the United States. In Immigrants and immigration policy: Individual skills, family ties, and group identities, H. Duleep and P. V. Wunnava, eds. Greenwich, CT: JAI Press.
Rivera-Batiz, Francisco L. 1999. Earnings of undocumented workers in the United States. Journal of Population Economics 12(1): 91–116.
Rogers, Richard, Robert A. Hummer, Charles B. Nam, and Kimberley Peters. 1996. Demographic, socioeconomic, and behavioral factors affecting ethnic mortality by cause. Social Forces 74(4): 1419–1438.
Singer, Audrey, and Douglas S. Massey. 1998. The social process of undocumented border crossing among Mexican migrants. International Migration Review 32(3): 561–593.
Social Security Administration. 1995. Technical panel on assumptions and methods report, 1994– 1995. Advisory Council on Social Security. Washington, DC: Social Security Administration.
Sorlie, Paul D., Eric Backlund, Norman Johnson, and Eugene Rogot. 1993. Mortality by Hispanic status in the U.S. Journal of the American Medical Association 270(20): 2464–2468.
Smith, Ken R., and Cathleen D. Zick. 1994. Linked lives, dependent demise? Survival analysis of husbands and wives. Demography 31(1, February): 81–94.
Stellman, Steven, and Qiao Wang. 1994. Cancer mortality in Chinese immigrants to New York City comparison with Chinese in Tianjin and with United States-born whites. Cancer 73(4): 1270–1275.
U.S. Immigration and Naturalization Service. 1990. Statistical Yearbook of the Immigration and Naturalization Service, 1990. Washington, DC: U.S. Government Printing Office.
———. 1996. Statistical Yearbook of the Immigration and Naturalization Service, 1996. Washington, DC: U.S. Government Printing Office.
———. 1998. Statistical Yearbook of the Immigration and Naturalization Service, 1998. Washington, DC: U.S. Government Printing Office.
———. 2001. Statistical Yearbook of the Immigration and Naturalization Service, 2001. Washington, DC: U.S. Government Printing Office.
Warren, Robert, and Ellen Percy Kraly. 1985. The elusive exodus: Emigration from the United States. Occasional Paper No. 8. Population Reference Bureau.
White, Michael J., Frank Bean, and Thomas Espenshade. 1990. The U.S. IRCA and undocumented migration to the United States. Population Research and Policy Review 9: 93–116.
Zick, Cathleen D., and Ken R. Smith. 1991. Marital transitions, poverty, and gender differences in mortality. Journal of Marriage and the Family 53: 327–336.