The Comprehensive Wealth of Older Immigrants and Natives
Social Security Bulletin, Vol. 79 No. 4, 2019 (released November 2019)
Research has pointed to notable differences between immigrants and the native-born in individual components of retirement resources. To compare the retirement preparation of older immigrants and natives, we calculate measures of comprehensive available resources and an annualized equivalent of comprehensive resources. We document large immigrant-native differences in median profiles of annualized wealth. We also examine how annualized wealth varies by immigrant arrival cohort and find that recent waves of immigrants are poorly situated. Finally, we use regression analysis to estimate the extent to which immigrant-native gaps in retirement resources are attributable either to differences in observable characteristics or to differences in returns to those characteristics.
David Love and Lucie Schmidt are professors of economics at Williams College.
Acknowledgments: We thank the Michigan Retirement Research Center (MRRC) for funding. We benefited from the excellent research assistance of Jesse Freeman and Rebecca Lewis. We are grateful to Purvi Sevak for helpful conversations; to Maria Enchautegui, Delia Furtado, and Tara Watson for detailed comments; and to participants at the 2014 MRRC Workshop, the 17th Annual Retirement Research Consortium Conference, and seminars held at Washington University–St. Louis, Wellesley College, Williams College, and Goethe University. We also thank Michael Leonesio and three anonymous referees for their insightful comments and suggestions.
This research was supported by a grant (no. RRC08098401-07) from the Social Security Administration to the Michigan Retirement Research Center as part of the Retirement Research Consortium.
Contents of this publication are not copyrighted; any items may be reprinted, but citation of the Social Security Bulletin as the source is requested. The findings and conclusions presented in the Bulletin are those of the authors and do not necessarily represent the views of the Social Security Administration.
Introduction
COLA | cost-of-living adjustment |
HRS | Health and Retirement Study |
OLS | ordinary least square |
SSA | Social Security Administration |
The 1965 Immigration and Nationality Act replaced a national-origins quota system with one based on family ties and skilled labor demand. The law had a profound effect on the provenance of immigrants arriving in the United States.1 The foreign-born share of the population changed, as did the demographic and skill composition of immigrants across arrival cohorts. Numerous studies2 have examined the relative earnings of immigrants and natives.3 However, wealth accumulation and retirement preparation among immigrants have not been widely examined. Understanding more about the retirement resources of immigrants is important for at least two reasons. First, immigrants are projected to become a much larger share of the aged population in the near future, doubling from 10 percent to 20 percent between 2005 and 2050 (Passel and Cohn 2008), which underscores their increasing influence on populationwide retirement wealth. Second, the initial waves of post-1965 immigrants are now reaching retirement age, and they differ substantially from previous waves in terms of countries of origin, earnings histories, and wealth.
The retirement resources of immigrants and natives differ in notable ways. Immigrants tend to have lower net worth (Cobb-Clark and Hildebrand 2006; Favreault and Nichols 2011), lower Social Security benefits (Cohen and Iams 2007; Favreault and Nichols 2011; Sevak and Schmidt 2014), and lower rates of private pension coverage (Osili and Paulson 2009; Heim, Lurie, and Ramnath 2012). Among homeowners, immigrants also tend to have higher home equity than natives (Chatterjee and Zahirovic-Herbert 2011; Sevak and Schmidt 2014).4 Taken together, these studies shed light on each of the major components—financial, nonfinancial, and annuitized—of retirement resources. However, analyses of immigrant wealth have not yet examined the contribution of all of the components to a comprehensive wealth balance sheet.
This article is the first to examine how retirement resources differ between natives and immigrants using a broad measure of wealth that includes the present value of expected pension and Social Security benefits, which for many households finance the bulk of retirement spending (Gustman and Steinmeier 1999; Gustman, Steinmeier, and Tabatabai 2010). The comprehensive balance sheet provides insights into immigrant-native differences in retirement preparation that are not available through standard measures of net worth alone. For example, the present-value measures of future pensions and Social Security are likely to differ substantially between recently arrived immigrants and natives of similar ages because pension formulas depend on years of service and Social Security benefits are a function of lifetime covered earnings. We calculate the measures of comprehensive wealth for immigrants and natives using data from the 1998–2012 waves of the Health and Retirement Study (HRS). We find that immigrants have significantly lower levels of comprehensive wealth, but that there is a great deal of heterogeneity within the immigrant population, particularly along the dimension of year of U.S. arrival. More recent waves of immigrants have substantially less wealth in all forms (financial, nonfinancial, and annuitized) than natives and earlier waves of immigrants alike.
We also calculate an annualized equivalent of comprehensive wealth and examine median profiles of annualized comprehensive wealth to simulate potential income streams over the retirement period. Our method for constructing both the comprehensive wealth measure and its annualized equivalent closely follows that of Love, Smith, and McNair (2008) and Love, Palumbo, and Smith (2009). For a household headed by an individual of a given age, the annualized measure is equivalent to the income derived from a real, joint-life annuity purchased with the full value of comprehensive wealth. The income provided by that annuity is our measure of annualized wealth, which serves as a rough measure of potential consumption in each remaining year of life. In contrast with comprehensive wealth, which does not account for the age or composition of households, annualized wealth provides trajectories that indicate whether households draw down resources faster or slower than a simple lifecycle model would predict. Previous research found that annualized comprehensive wealth rises with age, suggesting slower drawdown (Love, Palumbo, and Smith 2009). However, when we apply this approach to an analysis of immigrant-native differentials, we find that annualized comprehensive wealth rises faster for immigrants than for natives, which implies that immigrants spend down retirement resources more slowly than does the overall population.
Additionally, we estimate descriptive median regressions of annualized wealth, both to see whether observable characteristics can explain immigrant-native gaps and to examine the extent of convergence in annualized resources across different immigrant arrival cohorts. Working through regression specifications that include controls for demographic characteristics, lifecycle factors, transfers to and from family members, and immigrant country of origin and race/ethnicity, we find that recent immigrant cohorts show lower levels of annualized wealth, even after we control for an extensive set of observable characteristics. Finally, we decompose the immigrant-native differences in annualized wealth into the effects that can be explained by differences in observable characteristics and the effects that are attributable to differences in the “returns” to those observable characteristics.5 We find that the gap between the most recent wave of immigrants and natives is about three-fourths attributable to characteristics and about one-fourth attributable to returns.
Our results suggest that recent waves of immigrants tend to reach retirement with substantially lower resources than do immigrants who arrived before the 1965 immigration act. The HRS data now contain the first waves of post-1965 immigrants to reach retirement age, and our results may thus serve as a bellwether for the retirement preparation of future immigrants. From a public policy perspective, a widespread shortfall in retirement resources raises important questions for social insurance programs,6 including questions about Social Security rules that may disadvantage immigrants with fewer quarters of covered earnings (Sevak and Schmidt 2014). Understanding more about immigrant wealth is therefore important from the perspectives of both welfare economics and public policy.
Background
An extensive literature has investigated the relative earnings of immigrants and natives and the convergence of those groups' relative earnings over time. The literature points to the importance of arrival cohort and country of origin for retirement outcomes. In this section, we discuss possible reasons why the retirement resources of immigrants and natives might differ, even after controlling for lifetime earnings.
Immigrants and Social Security
Because Social Security rules link benefit levels to lifetime covered earnings, immigrants are likely to receive lower benefits than the native-born. Eligibility for retirement benefits requires a worker to accrue 40 quarters of coverage by meeting earnings thresholds over a minimum of 10 years. Many immigrants have insufficient quarters of covered earnings (or of reported earnings) to qualify. Empirical evidence largely confirms that projected and actual Social Security benefits are lower for immigrants than for natives, even after controlling for an extensive array of health and socioeconomic characteristics. Cohen and Iams (2007) used a microsimulation model to predict that the foreign-born will be significantly less likely to receive Social Security benefits. Favreault and Nichols (2011) linked Survey of Income and Program Participation data to administrative Social Security records and found that immigrants have lower Social Security benefits than natives. They also found that immigrants are much more likely to have made Social Security payroll-tax contributions but not be eligible for benefits. Sevak and Schmidt (2014) used HRS data linked to Social Security earnings records to show that immigrants have significantly lower predicted Social Security benefits, but that this gap is strongly related to years in the United States and is entirely explained by differences in quarters of covered earnings.
Immigrants and Private Wealth
Despite lower Social Security benefits, immigrants may adequately prepare for retirement if they amass sufficient private wealth to compensate. Immigrants are a heterogeneous population; but on average, that compensation does not appear to occur. Relative to natives, immigrants have lower saving rates (Carroll, Rhee, and Rhee 1994; 1999), significant differences in portfolio allocations (Cobb-Clark and Hildebrand 2006; Osili and Paulson 2009), and lower levels of net worth and projected retirement well-being (Cobb-Clark and Hildebrand 2006; Favreault and Nichols 2011; Sevak and Schmidt 2014). In addition, immigrants have lower levels of private pension coverage than natives (Osili and Paulson 2009; Heim, Lurie, and Ramnath 2012; Sevak and Schmidt 2014). Heim, Lurie, and Ramnath found that the participation gap is primarily due to immigrants being less likely to work for firms that offer pension plans, rather than differential take-up rates.
Housing may be particularly important when considering immigrant-native differentials, given the significance of homeownership to immigrants as a symbol of assimilation (Anacker 2013). Research shows that immigrants are significantly less likely to own homes than natives (Borjas 2002; Cobb-Clark and Hildebrand 2006; Sevak and Schmidt 2014). However, among homeowners, immigrants have higher levels of home equity, even before controlling for observable characteristics (Chatterjee and Zahirovic-Herbert 2011; Sevak and Schmidt 2014). Drew (2002) found that the median value of first-time home purchases among the foreign-born was 50 percent higher than that of the native-born and that, as a result, immigrants made larger down payments and held more home equity. This is in part due to the concentration of immigrants in areas with high housing costs such as California and New York. Similarly, Borjas (2002) found that observable demographic characteristics do not explain much of the homeownership gap between immigrants and natives, but that geographic locations play a role.
Data
We examine immigrant and native retirement resources for households with respondents aged 51 or older using data from eight waves of the HRS, spanning 1998–2012.7 For studies of comprehensive wealth, the HRS has several advantages over other national surveys. As described in detail in Smith (1995), the HRS questionnaire was specifically designed to minimize bias in measures of wealth by using an unfolding-brackets methodology.8 Consequently, the HRS provides a more complete picture of private wealth than most other data sets do. The HRS results closely match the wealth distribution derived from the cross-sectional Survey of Consumer Finances (SCF) except for the top 1 percent, for which the HRS underreports wealth relative to the SCF (Sierminska, Michaud, and Rohwedder 2008). However, because we focus on the financial behavior of the median household, the discrepancy in the top 1 percent of the wealth distribution should not strongly affect our analysis.
In addition to the publicly available HRS data, we use restricted geocoded data for 1992–2012 from the HRS Cross-Wave Geographic Information (Detail) file and restricted Social Security Administration (SSA) covered earnings records for 1951–2013 from the Respondent Cross-Year Summary Earnings file. The geocoded data include country of origin as well as state and urbanicity of current residence. Because restrictions prohibit combining the geocoded data with the earnings data, we analyze the data from those modules separately.
Our HRS panel includes six birth-cohort subgroups. The original HRS wave, introduced in 1992, surveyed respondents born during 1931–1941. In the 1993 wave, called the AHEAD survey, respondents were born in 1923 or earlier. Beginning in 2012, survey respondents included members of four additional cohorts (those born during 1924–1930, 1942–1947, 1948–1953, and 1954–1959). Approximately 11 percent of HRS respondents overall are foreign-born, with the rate varying from 8 percent of those born 1942–1947 to 14 percent of those born 1948–1953.9 The availability of longitudinal data on multiple birth cohorts allows us to examine wealth trajectories by age and by birth cohort simultaneously.10
In the following subsections, we summarize our methodologies for calculating comprehensive and annualized wealth. Appendix A provides detailed descriptions.
Estimating Comprehensive Wealth
We follow Gustman and Steinmeier (1999); Wolff (2007); Gustman, Steinmeier, and Tabatabai (2009); and Love, Palumbo, and Smith (2009) in constructing a comprehensive measure of the household balance sheet. This measure includes both conventional sources of net worth and the actuarial present value of expected future streams of income derived from pensions, annuities, future earnings (to age 65), Social Security, and other social insurance programs. Apart from the usual concerns about measurement error in survey data on wealth (Gustman and others 1997), the calculation of the financial and nonfinancial components of comprehensive wealth is straightforward. The financial component includes stocks, bonds, checking accounts, certificates of deposit, defined contribution pensions, individual retirement accounts, Keogh accounts, and other savings, minus nonvehicle and nonhousing debt. Nonfinancial comprehensive wealth includes the net value of primary and secondary housing, the net value of vehicles, and any investment and business real estate minus associated debt.11
Estimating Annualized Wealth
To measure the annual contribution of each source of comprehensive wealth, we need to know not only the amounts of each future income stream but also when the payments will start, how long they will continue, and whether cost-of-living adjustments (COLAs) or survivor's benefits apply. HRS questions address all future streams of income and use unfolding brackets to narrow the responses. Thus, for a given annualized stream of payments, computing the present value is relatively straightforward. We discount each of these cash flows using payment details, interest rate assumptions, and survival probabilities. To discount the benefit streams, we experimented with various interest rates (including the full yield curve on Treasury debt) and chose a 4.5 percent nominal rate of return for future nominal payments that will not receive COLAs. For all other future payments, such as Social Security benefits or pensions with COLAs, we assumed that the payments will keep pace with an expected inflation rate of 2 percent, which approximates the Federal Reserve Board's target for the annual rate of change in the price index for personal consumption expenditures.
We allow for survival-rate differentials by education, race/ethnicity, and sex. We start with a baseline set of mortality rates by sex and age from the 2010 Social Security Period Life Table (SSA 2015, Table 4.C6). We then adjust those probabilities using ratios of subgroup mortality rates to aggregate rates estimated in Brown, Liebman, and Pollet (2001, Tables A.1 and A.2). Those estimated ratios were based on data from the National Longitudinal Mortality Survey for three educational-attainment groups (less than high school, high school diploma but less than 4-year college degree, and 4-year college degree) and by sex, race (non-Hispanic white and black), and Hispanic origin. We then compute our respondent-specific mortality rates by applying a linear approximation of Brown, Liebman, and Pollet's ratios to the mortality rates in the Social Security life table.12 In this way, we generate separate mortality rates by race (white or black), education (less than high school, high school diploma, or college degree), Hispanic origin, and sex.13,14
By far the most important source of future income for most U.S. households is Social Security. The HRS asks respondents to report their current and expected future Social Security benefits—both for themselves and for their spouses (if married). Not surprisingly, self-reported current benefit values tend to be more accurate than predictions of future benefits.15 As shown in Appendix A, our measure of the present value of the Social Security income stream discounts future benefits by the relevant differential survival probabilities and adjusts for survivor benefits.16 The present-value calculation for defined-benefit pensions, veterans' benefits, future earnings to age 65, annuities, and other sources of future nonlabor income follows a similar procedure. However, we compute the present values separately for the respondent and the spouse, and we include a COLA and spousal benefits only if respondents report them in the survey (see Appendix A).17
Results
This section presents our estimates of comprehensive and annualized wealth for immigrants and natives. It also presents median wealth profiles.
Comprehensive Wealth
In this section, we focus on comprehensive wealth of married couples.18 Although the patterns of comprehensive wealth for single men and women are similar to those of married couples, their levels of wealth are much lower and the statistical relationships are much noisier.19
Table 1 shows the weighted means, medians, and 25th and 75th percentiles of comprehensive wealth by selected component, immigrant status, and age. Immigrants have significantly lower levels of wealth than natives at the mean and throughout the distribution. The median comprehensive wealth of married immigrants, for example, ranges from 62 percent to 70 percent that of their native counterparts, depending on the age bracket. At the 25th percentile, these differences are even more pronounced, with married immigrants holding about 54 percent as much comprehensive wealth as their native counterparts. Most of the overall difference stems from differences in financial wealth and Social Security wealth. Immigrants are much more similar to natives in nonfinancial wealth, an important pattern that we will revisit.
Origin | Financial | Nonfinancial | Social Security | Comprehensive | ||||
---|---|---|---|---|---|---|---|---|
65–74 | 75–84 | 65–74 | 75–84 | 65–74 | 75–84 | 65–74 | 75–84 | |
Mean | ||||||||
Native | 390 | 348 | 417 | 384 | 427 | 268 | 1,809 | 1,242 |
Immigrant | 379 | 270 | 475 | 342 | 368 | 246 | 1,447 | 1,022 |
t-statistic of difference | 0.2 | 1.7 | -0.8 | 1.2 | 7.0 | 3.2 | 1.7 | 2.9 |
Median | ||||||||
Native | 113 | 108 | 197 | 188 | 414 | 248 | 1,174 | 837 |
Immigrant | 11 | 16 | 163 | 160 | 354 | 237 | 733 | 589 |
t-statistic of difference | 21.5 | 13.8 | 3.4 | 1.6 | 7.5 | 1.5 | 16.1 | 5.8 |
25th percentile | ||||||||
Native | 13 | 17 | 96 | 95 | 303 | 182 | 709 | 498 |
Immigrant | 0 | 0 | 36 | 15 | 229 | 155 | 386 | 271 |
t-statistic of difference | 14.6 | 16.4 | 8.7 | 8.5 | 6.9 | 4.0 | 18.5 | 11.8 |
75th percentile | ||||||||
Native | 401 | 358 | 395 | 374 | 532 | 323 | 1,926 | 1,451 |
Immigrant | 195 | 199 | 405 | 382 | 483 | 332 | 1,465 | 1,273 |
t-statistic of difference | 9.1 | 5.0 | -0.4 | -0.3 | 4.0 | -1.1 | 5.4 | 2.4 |
SOURCE: Authors' calculations based on HRS (1998–2012 waves). | ||||||||
NOTES: Values are weighted.
Married couples are those in which both spouses are immigrants or natives and the couple was married when the respondent participated in his or her first HRS wave.
The age of the older spouse determines the household's age group.
Comprehensive wealth includes non–Social Security annuitized wealth.
|
In Chart 1, we compare the distributions of comprehensive wealth for married natives and immigrants by plotting the kernel densities of comprehensive wealth for married households. The lines plot the fractions of immigrants and natives holding the wealth levels shown on the horizontal axis. Comparing the two densities, we can see that a larger share of immigrants holds lower levels of wealth, while a larger share of natives holds higher levels of wealth.
Kernel density of comprehensive wealth of married immigrant and native households aged 60–89
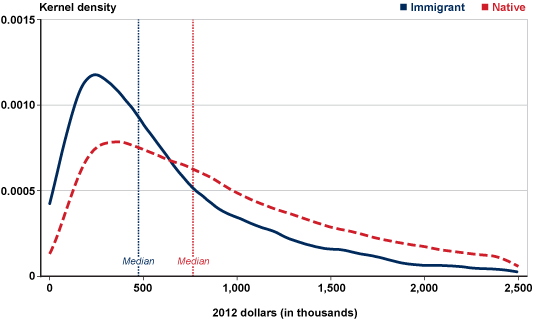
Annualized Wealth
To complement the comprehensive measure of wealth, we calculate an annualized equivalent to show how differences in total wealth translate into different consumption and welfare possibilities. For example, a given amount of comprehensive wealth will imply more consumption possibilities for older single individuals than for younger married households. Although it is difficult to calculate an exact welfare measure without making strong assumptions about the structure of preferences, we can compute an approximate measure that relates total resources to an annual equivalent per household member, as in Love, Palumbo, and Smith (2009).
In particular, we imagine that a household uses its entire comprehensive wealth to purchase an actuarially fair, real, joint-life annuity whose price is computed using the differential survival probabilities discussed above. The income level delivered by that annuity is our measure of annualized wealth (Appendix A describes the calculation). The primary advantage of the annualized measure is that it automatically adjusts for household size, expected remaining years of consumption, and survival probabilities. It also reflects the familiar notion of permanent income and, therefore, provides an approximate measure of the consumption possibilities available in each remaining year of life. By contrast, the stock value of total household resources, for example, tells us less about the consumption and welfare implications of a given amount of savings.20
Both the levels and the growth rate of annualized wealth can yield important insights into the adequacy of household resources. Annualized wealth levels at or below the poverty line for a demographic subset of households would be concerning. Conversely, an increase in annualized wealth for most households over time would imply that households could afford increased spending (or bequests) with age; yet if the levels instead tended to decline with age, there might be concern that households were spending down their resources at an unsustainable rate.
However, the annualized equivalent of wealth might not correspond directly with actual consumption possibilities, particularly if not all forms of wealth are equally fungible. For example, if the majority of a family's comprehensive wealth is the present value of its future Social Security benefits, the household may not have access to an annual equivalent of this wealth until its members are eligible to start receiving their benefits. A similar concern about fungibility would apply to housing, in that reverse mortgages remain uncommon and tapping into equity could entail substantial transaction costs.
Chart 2 plots the kernel densities of annualized wealth for immigrant and native married households, with vertical lines marking the median values for the two groups. As with comprehensive wealth, a larger share of immigrants holds lower levels of wealth, while a larger share of natives holds higher levels.
Kernel density of annualized comprehensive wealth of married immigrant and native households aged 60–89
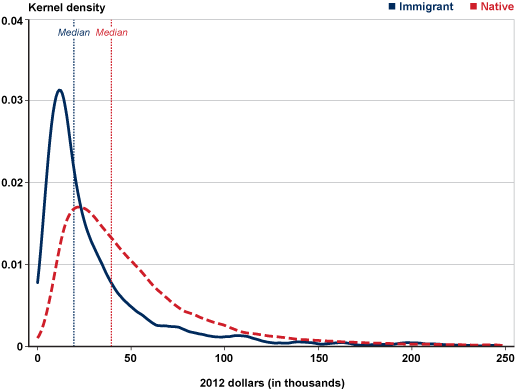
Wealth Profiles
To see how retirement wealth changes for immigrants and natives as they age, we begin by examining regression-based age profiles of comprehensive wealth using a technique developed in Love, Palumbo, and Smith (2009). We use the median growth rates within households to trace the typical trajectory of wealth over time for a given population. The premise is straightforward: If we knew a household's initial amount of wealth and the projected growth rates of wealth across future ages, we would be able to construct the full age-path of wealth.21
The advantage of the technique is that it helps mitigate cohort effects, nonrandom attrition, and survivorship bias that may induce differences in the observed levels of wealth for different ages at a given time. The cohort effects are largely absorbed by the survey-year and age dummies. Nonrandom attrition is eliminated because the growth rates are necessarily calculated for survivors. Finally, because the growth rates of wealth tend to differ much less than the levels for survivors versus nonsurvivors (Love, Palumbo, and Smith 2009), the regression-based approach tends to reduce survivorship bias as well.
Chart 3 displays the age trajectories of median comprehensive wealth for married immigrants and natives aged 60 to 90 (specifically, for the median age within each of the 5-year age brackets). The gap between immigrants and natives in comprehensive wealth is substantial at all ages. Natives begin this phase with almost $1.2 million in comprehensive wealth, while immigrants start with only about $800,000. Despite the initial difference, however, the wealth gap between the two groups converges over the retirement years.22
Comprehensive wealth profiles of married immigrants and natives, by age of older spouse (in 2012 dollars)
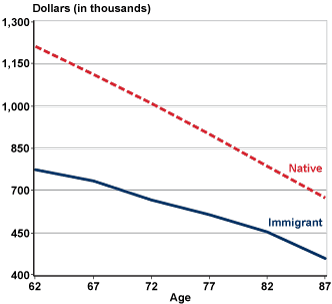
The decline with age in comprehensive wealth for both natives and immigrants does not necessarily imply the same pattern for annualized wealth. Chart 4 displays trajectories of annualized wealth for immigrant and native married couples using the same median regression-based technique. The annualized profiles for both groups slope upward (though only slightly in the case of natives), which is consistent with findings in Love, Palumbo, and Smith (2009). Declines in comprehensive wealth combined with rising annualized wealth imply that households draw down their total resources more slowly than a simple lifecycle model would suggest. As with comprehensive wealth, the annualized wealth profiles for immigrants and natives converge with age. At the youngest potential retirement ages, immigrants have annualized wealth about $15,000 lower than that held by natives, but the difference narrows slightly as households approach age 80. Thus, although native and immigrant married couples both appear to be drawing down resources more slowly than a simple lifecycle framework would predict, we find some evidence that immigrants are especially slow to spend down retirement wealth.23 Patterns for unmarried men and unmarried women (available from the authors on request; see note 19) show a similar rise in annualized wealth, albeit with more noise.
Annualized comprehensive wealth profiles of married immigrants and natives, by age of older spouse (in 2012 dollars)
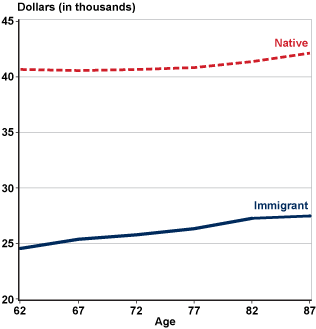
One drawback to our growth-based method of tracing median annualized wealth is that median growth rates need not correspond with the median levels of annualized growth to which we anchor the trajectories in Chart 4.24 Ideally, we would estimate growth-based profiles for households with annualized wealth near the median for each age bracket, but we do not have enough observations to accurately estimate growth rates for the age cells used to construct Chart 4. However, we can align the median growth rates and levels if we consider much wider age brackets.
Table 2 shows the annualized wealth levels and growth rates by component for married households with annualized wealth within 25 percent (plus or minus) of the median level for each age and immigration-status group. Despite having substantially lower median annualized financial and annuitized wealth levels than natives, immigrants aged 65–74 and 75–85 have similar levels of housing wealth. This pattern is also reflected in the shares of total nonfinancial wealth, as immigrants aged 75–85 hold about 14 percentage points more of their portfolios in the form of nonfinancial wealth than do natives in that age group.
Age and origin | Financial | Nonfinancial | Annuitized | Comprehensive | |
---|---|---|---|---|---|
Housing | Total | ||||
Amount (2012 dollars) | |||||
55–64 | |||||
Native | 3,059 | 3,486 | 4,913 | 18,025 | 38,838 |
Immigrant | 99 | 2,143 | 3,000 | 9,971 | 19,314 |
t-statistic of difference | 29.9 | 4.0 | 4.0 | 14.4 | 43.7 |
65–74 | |||||
Native | 4,570 | 5,832 | 7,744 | 23,170 | 39,851 |
Immigrant | 416 | 5,780 | 6,607 | 14,971 | 24,543 |
t-statistic of difference | 20.7 | 0.1 | 2.0 | 17.3 | 24.2 |
75–85 | |||||
Native | 6,380 | 9,191 | 11,805 | 21,212 | 44,294 |
Immigrant | 1,031 | 10,107 | 12,199 | 14,011 | 29,603 |
t-statistic of difference | 9.2 | -1.0 | -0.4 | 8.9 | 19.3 |
Annual growth rate a (%) | |||||
55–64 | |||||
Native | 6.4 | 4.3 | 3.9 | 2.8 | 0.4 |
Immigrant | 4.8 | 8.2 | 6.1 | 1.6 | -0.9 |
t-statistic of difference | 0.3 | -2.6 | -1.6 | 2.1 | 3.0 |
65–74 | |||||
Native | 3.1 | 5.4 | 4.2 | -0.3 | 0.3 |
Immigrant | -1.6 | 7.3 | 6.2 | -0.2 | 0.6 |
t-statistic of difference | 3.4 | -2.6 | -3.0 | -0.6 | -0.5 |
75–85 | |||||
Native | 2.8 | 6.3 | 5.0 | -0.9 | 0.9 |
Immigrant | 1.3 | 12.9 | 10.2 | -0.3 | 1.1 |
t-statistic of difference | 0.4 | -2.7 | -3.8 | -2.3 | -0.2 |
SOURCE: Authors' calculations based on HRS (1998–2012 waves). | |||||
NOTES: Values are medians for all households between the 25th and 75th percentiles for annualized wealth within their age and immigrant-status groups.
Married couples are those in which both spouses are immigrants or natives and the couple was married when the respondent participated in his or her first HRS wave.
The age of the older spouse determines the household's age group.
|
|||||
a. Among households with positive holdings. |
The table's lower panel shows that immigrant households near the median of annualized wealth experience faster growth in nonfinancial wealth than do natives. The growth-rate differences persist across all of the age groups, and are widest for the oldest group. Most of the differential growth in nonfinancial annualized wealth appears to be due to housing. For example, although natives aged 75–85 saw an annual increase in housing wealth of about 6.3 percent over the study period, immigrants in the same age bracket experienced an increase twice as large. Housing wealth is likely to be a particularly important indicator for immigrants, both because it is large relative to financial wealth and because it is relatively illiquid.
Along with understanding the importance of housing, we want to see how annualized wealth depends on factors that pertain particularly to immigrants, including country of origin, immigration cohort, race, and ethnicity. Therefore, the next section turns to a rich regression analysis of the covariates of annualized wealth for immigrants and natives.
Empirical Analysis of Native and Immigrant Wealth
Table 3 shows weighted mean and median levels of annualized wealth by age (65–74 and 75–85), education, race and ethnicity, and immigration cohort (pre-1955, 1955–1964, 1965–1974, 1975–1984, and 1985 or later) for households headed by a married couple at the time of the respondent's first survey wave. For both age groups, immigrants have lower mean and median annualized wealth than their native counterparts at all education levels (except for mean wealth of those aged 75–85 who did not complete high school). Patterns are similar for unmarried men and unmarried women, but wealth levels are much lower for those groups (not shown). Race affects the wealth differentials, as immigrants fare better than natives in both mean and median wealth for both age groups and non-Hispanic racial categories (except for median wealth of nonwhites aged 75–85). This result is consistent with work by Sevak and Schmidt (2014), who found higher levels of total net worth for immigrants after controlling for demographic characteristics including race and ethnicity. However, the pattern does not hold for Hispanics, as native Hispanics hold almost twice as much annualized wealth as Hispanic immigrants at both the mean and the median.
Characteristic | Age 65–74 | Age 75–85 | ||||
---|---|---|---|---|---|---|
Immigrant | Native | t-statistic of difference | Immigrant | Native | t-statistic of difference | |
Mean | ||||||
Education | ||||||
Less than high school | 27.5 | 33.8 | 2.5 | 46.0 | 40.0 | -1.1 |
High school diploma | 40.2 | 49.4 | 4.1 | 48.0 | 61.8 | 3.1 |
College degree | 92.3 | 107.7 | 0.7 | 75.3 | 111.2 | 3.7 |
Race/ethnicity | ||||||
White non-Hispanic | 81.5 | 64.7 | -1.9 | 83.8 | 71.3 | -1.9 |
Nonwhite non-Hispanic | 59.3 | 34.8 | -3.1 | 36.1 | 35.5 | -0.1 |
Hispanic | 18.1 | 33.8 | 6.7 | 20.3 | 40.9 | 5.5 |
Immigration cohort | ||||||
Pre-1955 | 46.3 | . . . | . . . | 57.6 | . . . | . . . |
1955–1964 | 78.9 | . . . | . . . | 77.1 | . . . | . . . |
1965–1974 | 39.0 | . . . | . . . | 46.8 | . . . | . . . |
1975–1984 | 26.3 | . . . | . . . | 34.0 | . . . | . . . |
1985 or later | 14.3 | . . . | . . . | 9.1 | . . . | . . . |
Median | ||||||
Education | ||||||
Less than high school | 15.1 | 22.9 | 10.0 | 19.5 | 27.9 | 3.3 |
High school diploma | 29.5 | 38.8 | 7.3 | 30.0 | 45.0 | 5.1 |
College degree | 50.6 | 66.5 | 4.5 | 49.2 | 76.3 | 6.6 |
Race/ethnicity | ||||||
White non-Hispanic | 48.8 | 42.9 | -1.9 | 56.6 | 47.3 | -2.0 |
Nonwhite non-Hispanic | 31.4 | 24.6 | -2.9 | 17.9 | 24.0 | 1.8 |
Hispanic | 14.7 | 25.1 | 7.7 | 13.9 | 26.4 | 5.2 |
Immigration cohort | ||||||
Pre-1955 | 30.5 | . . . | . . . | 33.9 | . . . | . . . |
1955–1964 | 37.0 | . . . | . . . | 49.7 | . . . | . . . |
1965–1974 | 23.3 | . . . | . . . | 28.6 | . . . | . . . |
1975–1984 | 13.5 | . . . | . . . | 17.3 | . . . | . . . |
1985 or later | 8.0 | . . . | . . . | 7.0 | . . . | . . . |
SOURCE: Authors' calculations based on HRS (1998–2012 waves). | ||||||
NOTES: Married couples are those in which both spouses are immigrants or natives and the couple was married when the respondent participated in his or her first HRS wave.
The age of the older spouse determines the household's age group.
Total annualized wealth includes future earnings and other components not shown separately.
. . . = not applicable.
|
One possible explanation of the wealth differences among Hispanics may involve differences in wealth by immigration cohort. The table shows large differences in annualized wealth by year of arrival. The two earliest immigration cohorts (corresponding loosely to those arriving before the 1965 act) have several times the mean annualized wealth of immigrants arriving after 1984, and the differences at the median are almost as large. Thus, although the annualized resources of the most recent immigrants fall below the poverty line, the earliest immigrants appear to be much better off.
Differences in annualized wealth by immigrant cohort might reflect differences in the age distribution of the earlier and more recent arrivals, with younger people—who tend to have lower annualized wealth levels—being more predominant among the latter groups. The differences in annualized wealth levels by immigration cohort, however, are large even within age brackets. With the 65–74 age group as an example, Chart 5 illustrates. It shows the median annualized wealth by component for native households and for immigrant households by year of arrival. All are married households whose oldest member is aged 65–74. The chart indicates that annualized wealth for each successive arrival cohort of immigrants is dramatically lower than that of its predecessor. In addition, the most recent arrivals hold very little financial wealth and have much less nonfinancial wealth than earlier immigrants and natives. Because annualized wealth differs dramatically within its single age group, Chart 5 suggests that differences in the age distributions of successive immigrant arrival cohorts do not account for all the cross-cohort variance in wealth.
Median annualized comprehensive wealth for married households aged 65–74, by component: Immigrants by arrival cohort and natives (in 2012 dollars)
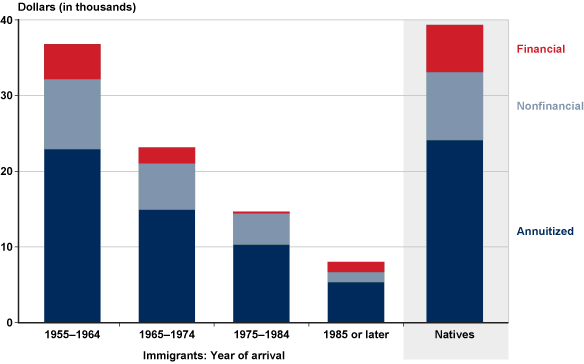
Median Regressions of Annualized Wealth
Immigrants and natives may differ along a number of potentially important observable characteristics, including health, education, earnings, wealth, and expectations about longevity and bequests. To see whether levels of annualized wealth differ between the groups after controlling for observables, we estimate median regressions of the logarithm of annualized wealth on five categories of key demographic and financial covariates. We focus on median regressions because wealth is unevenly distributed and we are interested in the experience of households near the middle of the distribution. These regressions are meant to be descriptive and should not be interpreted as implying causality. However, they will allow us to infer whether observable characteristics can fully account for the immigrant-native gap in annualized financial wealth. We have also estimated ordinary least square (OLS) regressions with qualitatively similar results (see Appendix B).
Empirical Approach
We assume that the conditional median of the logarithm of annualized wealth, y, is a linear function of a vector of observable characteristics. We are therefore interested in estimating the following median regression:
where the vector of the β regression coefficients can be interpreted as rates of return to the different characteristics at the median of the conditional distribution, and where i indexes households and t indexes the time period. Because errors are likely to be correlated within households over time, we cluster standard errors following the method in Parente and Santos Silva (2013).
We estimate the median regression to understand how much of the gap in annualized wealth between immigrant arrival cohorts and between immigrants and natives can be explained by several categories of observable characteristics, including demographics, lifecycle variables, intergenerational transfers, and immigrant origins and racial/ethnic backgrounds. We first estimate a set of median regressions, controlling for an increasing number of observable characteristics, and then examine the relative importance of characteristics versus returns to characteristics by applying the quantile decomposition described in Melly (2005).
Covariates and Summary Statistics
Table 4 shows the weighted means for the variables included in the regressions. Although our tables and charts have thus far focused on married couples (who have the highest levels of wealth), we now examine the potential role of marital status in determining immigrant-native wealth differentials. As such, we include all respondents in this sample, regardless of marital status.
Variable | Weighted mean | Standard deviation | Minimum | Maximum |
---|---|---|---|---|
Dependent variable | ||||
Natural logarithm of annualized wealth a | 10.42 | 0.88 | 1.68 | 15.66 |
Baseline covariates | ||||
Immigration cohort | ||||
Pre-1955 | 0.02 | 0.13 | 0.00 | 1.00 |
1955–1964 | 0.02 | 0.13 | 0.00 | 1.00 |
1965–1974 | 0.02 | 0.14 | 0.00 | 1.00 |
1975–1984 | 0.02 | 0.13 | 0.00 | 1.00 |
1985 or later | 0.01 | 0.10 | 0.00 | 1.00 |
Age | ||||
65–74 | 0.25 | 0.43 | 0.00 | 1.00 |
75 or older | 0.23 | 0.42 | 0.00 | 1.00 |
Demographic covariates | ||||
Married couple | 0.50 | 0.50 | 0.00 | 1.00 |
Immigrant and immigrant | 0.03 | 0.17 | 0.00 | 1.00 |
Immigrant and native | 0.03 | 0.18 | 0.00 | 1.00 |
Naturalized citizen | 0.06 | 0.23 | 0.00 | 1.00 |
Non-English speaker | 0.03 | 0.17 | 0.00 | 1.00 |
Education | ||||
High school diploma | 0.54 | 0.50 | 0.00 | 1.00 |
College degree | 0.26 | 0.44 | 0.00 | 1.00 |
Race/ethnicity | ||||
Hispanic— | ||||
White | 0.05 | 0.21 | 0.00 | 1.00 |
Nonwhite | 0.02 | 0.15 | 0.00 | 1.00 |
Nonwhite non-Hispanic | 0.13 | 0.34 | 0.00 | 1.00 |
Family size | 2.13 | 1.22 | 1.00 | 19.00 |
Number of children | 2.91 | 2.03 | 0.00 | 22.00 |
Lifecycle covariates | ||||
Share of wealth a from— | ||||
Financial assets | 0.13 | 0.34 | -23.60 | 31.01 |
Nonfinancial assets | 0.22 | 0.29 | -13.24 | 22.34 |
Logarithm of household earnings b | 11.06 | 5.74 | 0.00 | 15.91 |
Standard deviation of logarithm of household earnings b | 0.92 | 0.33 | 0.01 | 3.01 |
Urban residence c | 0.48 | 0.50 | 0.00 | 1.00 |
Homeowner | 0.79 | 0.41 | 0.00 | 1.00 |
Business owner | 0.10 | 0.29 | 0.00 | 1.00 |
Health status | ||||
Good | 0.34 | 0.47 | 0.00 | 1.00 |
Fair or poor | 0.34 | 0.48 | 0.00 | 1.00 |
Medical out-of-pocket costs | ||||
Middle tercile | 0.34 | 0.46 | 0.00 | 1.00 |
Highest tercile | 0.30 | 0.46 | 0.00 | 1.00 |
Transfer covariates | ||||
Expect to leave bequest | ||||
Deemed likely, greater than $10,000 | 0.70 | 0.46 | 0.00 | 1.00 |
Deemed likely, greater than $100,000 | 0.47 | 0.50 | 0.00 | 1.00 |
Child(ren) within 10 miles | 0.56 | 0.50 | 0.00 | 1.00 |
Transfers | ||||
To child(ren) | 0.40 | 0.49 | 0.00 | 1.00 |
From child(ren) | 0.05 | 0.22 | 0.00 | 1.00 |
To relative(s) | 0.10 | 0.30 | 0.00 | 1.00 |
From relative(s) | 0.03 | 0.18 | 0.00 | 1.00 |
Immigrant origin and race/ethnicity covariates | ||||
Immigrated from Mexico c | 0.01 | 0.12 | 0.00 | 1.00 |
Per capita income of country of origin c | ||||
High-middle | 0.03 | 0.18 | 0.00 | 1.00 |
Low-middle | 0.01 | 0.12 | 0.00 | 1.00 |
Low | 0.01 | 0.08 | 0.00 | 1.00 |
Race/ethnicity | ||||
Hispanic— | ||||
White | 0.01 | 0.11 | 0.00 | 1.00 |
Nonwhite | 0.02 | 0.16 | 0.00 | 1.00 |
Nonwhite non-Hispanic | 0.02 | 0.13 | 0.00 | 1.00 |
SOURCE: Authors' calculations based on HRS (1998–2012 waves), SSA earnings records, and geocoded data from HRS. | ||||
NOTE: Study sample consists of HRS households with a respondent or spouse aged 51 or older. | ||||
a. Reflects the value first reported by each household in its initial HRS wave. | ||||
b. Restricted earnings data sample only. | ||||
c. Restricted geocoded data sample only. |
The dependent variable in the regressions is the natural logarithm of annualized wealth. We restrict our sample to positive values of annualized wealth, which eliminates only about 0.3 percent of the respondents. The table organizes the covariates into five categories that correspond with the types of controls that we will use in the regression analysis: baseline, demographic, lifecycle, transfer payment, and immigrant origin and race/ethnicity. The baseline category is relatively sparse and includes only the immigration dummies, year dummies, and a set of age dummies (with ages 51–64 as the omitted category).
The demographic controls comprise dummies for marital status, citizenship status, whether English is spoken at home, education, race, and ethnicity. In addition, we include variables for family size and the total number of children (the latter including children not living in the respondent's household). Because the importance of marriage likely depends on whether the couple consists of two natives, two immigrants, or one immigrant and one native, we include dummies and interactions that control for each configuration.
The lifecycle covariates consist of a set of variables that are theoretically important in lifecycle models of saving. We control for the portfolio shares of financial and nonfinancial wealth (the share of annuitized wealth is a linear combination of the other two shares) because different liquidity characteristics across wealth categories could influence rates of drawdown in retirement (for example, a slow withdrawal of housing wealth).25 The restricted SSA earnings data allow us to construct a variable for average household Social Security–covered earnings (in 2012 dollars) from 1951 to 2013. We include covariates for both the logarithm of household earnings (plus one, to handle zeros) and the standard deviation of the logarithm of household earnings. The geocoded data allow us to control for whether respondents live in an urban area, defined as a county within a metro area that has a population of 1 million or more. As noted earlier, we cannot include both the restricted geocoded variables and the restricted earnings variables in the same regressions. We include dummies for whether individuals own houses and businesses because these assets may be harder to liquidate in retirement. Finally, we include dummy variables for health status and out-of-pocket medical costs as a way to control for the effects of these factors on retirement resources. “Excellent/very good” is the omitted health-status category, and we assign a household to the less favorable of the respondent's and spouse's reported health statuses. The medical out-of-pocket cost dummies indicate whether the household's costs are in the highest tercile or the middle tercile of the expense distribution.
The transfer covariates include bequest variables that take a value of 1 if respondents report a 50 percent or higher probability that they will bequeath a given amount to their child(ren). About half of the sample reports greater than even odds of leaving a bequest. We include a dummy variable for whether children live within 10 miles of the household to proxy for unobserved service transfers between children and parents.26 The transfer variables are indicators for “yes” answers to questions of the following form: “Including help with education but not shared housing or shared food, have you given [received] financial help totaling $500 or more to [from] any of your children [relatives]?” The transfer variables are especially important in the context of immigrant resources because they may capture part of the effect of unobserved remittances on annualized wealth.
The immigrant origin, race, and ethnicity category includes variables that may capture differences in initial opportunity (such as schooling), culture, and (along with the non-English speaker control) language barriers. We classify countries of origin based on per capita gross national income using the World Bank's fiscal year 2014 income categories: low ($1,035 or less), low-middle ($1,036 to $4,085), high-middle ($4,086 to $12,615), and high ($12,616 or more, the omitted category). We control separately for whether respondents migrated from Mexico given its border status and large migrant flows to and from the United States.
Annualized Wealth Regressions: Immigrants and Natives
Table 5 shows the coefficient estimates and standard errors for the equation described above under “Empirical Analysis of Native and Immigrant Wealth.” Because we consider both the association of immigration status with annualized wealth and the channels through which that association might emerge, we present cumulating estimates for five categorical specifications that control for the household characteristics that Table 4 lists individually.27 Because we cannot merge the restricted SSA earnings data with the restricted HRS geocoded data, we report results for the two samples separately.
Variable | Baseline covariates | With demographic covariates added | With lifecycle covariates added | With transfer-payment covariates added | With immigrant origin and race/ethnicity covariates added | |||||
---|---|---|---|---|---|---|---|---|---|---|
Coefficient | Standard error | Coefficient | Standard error | Coefficient | Standard error | Coefficient | Standard error | Coefficient | Standard error | |
Earnings data sample | ||||||||||
Immigration cohort | ||||||||||
Pre-1955 | -0.222* | 0.124 | -0.053 | 0.073 | 0.051 | 0.040 | 0.050 | 0.056 | 0.105** | 0.041 |
1955–1964 | -0.147** | 0.066 | 0.025 | 0.058 | 0.092** | 0.042 | 0.063 | 0.039 | 0.125*** | 0.045 |
1965–1974 | -0.626*** | 0.071 | -0.147*** | 0.047 | -0.029 | 0.036 | -0.034 | 0.040 | 0.045 | 0.039 |
1975–1984 | -0.932*** | 0.061 | -0.311*** | 0.045 | -0.145*** | 0.040 | -0.162*** | 0.039 | -0.080 | 0.049 |
1985 or later | -1.246*** | 0.070 | -0.662*** | 0.073 | -0.186*** | 0.052 | -0.237*** | 0.078 | -0.169** | 0.074 |
Constant | 10.342*** | 0.010 | 9.917*** | 0.020 | 8.137*** | 0.072 | 8.427*** | 0.071 | 8.431*** | 0.071 |
R-squared | 0.066 | 0.348 | 0.381 | 0.526 | 0.526 | |||||
Observations | 105,268 | 103,289 | 81,138 | 69,055 | 69,055 | |||||
Geocoded data sample | ||||||||||
Immigration cohort | ||||||||||
Pre-1955 | -0.222* | 0.124 | -0.053 | 0.073 | 0.000 | 0.031 | 0.017 | 0.034 | 0.061 | 0.040 |
1955–1964 | -0.147** | 0.066 | 0.025 | 0.058 | 0.065 | 0.041 | 0.053 | 0.037 | 0.109*** | 0.042 |
1965–1974 | -0.626*** | 0.071 | -0.147*** | 0.047 | -0.094** | 0.039 | -0.078* | 0.041 | -0.001 | 0.045 |
1975–1984 | -0.932*** | 0.061 | -0.311*** | 0.045 | -0.204*** | 0.035 | -0.216*** | 0.043 | -0.126** | 0.050 |
1985 or later | -1.246*** | 0.070 | -0.662*** | 0.073 | -0.414*** | 0.053 | -0.464*** | 0.060 | -0.355*** | 0.094 |
Constant | 10.342*** | 0.010 | 9.917*** | 0.020 | 9.389*** | 0.017 | 9.378*** | 0.019 | 9.377*** | 0.018 |
R-squared | 0.066 | 0.348 | 0.285 | 0.389 | 0.389 | |||||
Observations | 105,268 | 103,289 | 103,289 | 86,382 | 86,382 | |||||
SOURCE: Authors' calculations based on HRS (1998–2012 waves), SSA earnings records, and geocoded data from HRS. | ||||||||||
NOTES: All covariate category specifications include a full set of year dummies.
Standard errors are clustered at the household level.
* = statistically significant at the p < 0.10 level; ** = statistically significant at the p < 0.05 level; *** = statistically significant at the p < 0.01 level.
|
The baseline specification includes only the immigrant cohort dummies and, to represent the first and second halves of retirement, a pair of age dummies. The baseline covariates examine the relationship between immigration status and annualized wealth without controlling for demographics, financial variables, or immigrant origins. The coefficient estimates on the cohort dummies indicate that immigrants hold less annualized wealth than natives and that their annualized wealth increases with years in the United States. Although the coefficient estimates imply that the first two cohorts (pre-1955 and 1955–1964) respectively have 20 percent and 13 percent less wealth than natives , the wealth levels of the final three immigrant cohorts (1965–1974, 1975–1984, and 1985 or later) are lower by 47 percent, 61 percent, and 71 percent, respectively.
With the addition of the demographic controls, the coefficient estimates on the immigrant cohorts fall substantially, and those on the three most recent cohorts remain statistically significant at the 1 percent level. The estimates indicate that these cohorts respectively have 14 percent, 27 percent, and 48 percent less median annualized wealth than native households have.
The lifecycle variables further shape the wealth trajectories. The prohibition of merging restricted data on earnings and geography does not affect the estimates using baseline and demographic covariates—both panels of Table 5 contain the same values in those columns—but it does produce different estimates for the lifecycle covariates. The top panel includes measures of the level and variation in lifetime earnings based on the restricted SSA data, while the bottom panel introduces a control for whether households live in a highly populated urban area. In both specifications, the introduction of the lifecycle variables absorbs some of the association between immigration cohort and annualized wealth, particularly in the case of the regression controlling for lifetime Social Security–covered earnings. Although the addition of lifecycle covariates lowers the absolute value of the estimated coefficient from 0.662 to 0.414 for the post-1985 immigration cohort in the regression using the geocoded data, the estimate (again, in absolute value) declines further still—from 0.662 to 0.186—when we control for lifetime earnings. These estimates suggest that much, but not all, of the differences in annualized wealth between the more recent cohorts and their native counterparts can be explained by lifecycle factors and by differences in their earnings histories.
The patterns of the coefficient estimates on the individual lifecycle variables, detailed in Appendix B, are consistent with the predictions of a lifecycle model that includes housing wealth. In particular, owning a house is strongly associated with higher annualized wealth. The high transaction costs associated with housing may cause households to withdraw housing wealth slowly in retirement.
With the addition of controls for bequests and transfers, the estimated coefficients all indicate a statistically strong relationship with annualized wealth, as wealth is positively associated with bequests and transfers to family members and negatively associated with transfers from family members. However, transfers do not substantially change the coefficient estimates on the immigration cohort dummies.
Controlling for immigrant origins and race and ethnicity further reduces the estimated coefficient for the 1965–1974 cohort dummy, but the estimates for the most recent immigrant cohort remain strongly negative and statistically significant. Thus, although controlling for a rich set of observables including lifetime earnings, geographic origins, and other lifecycle variables substantially reduces the measured immigrant-native gap in annualized wealth, a portion remains unexplained for the most recent cohorts. Shortfalls range from 8 percent to 11 percent for the 1975–1984 cohort and from 16 percent to 30 percent for the most recent cohort, depending on whether we control for restricted earnings or geocoded information.
Although we cannot separately identify the importance of the immigration cohort and the age of immigrants at arrival, we can explore their relative importance by considering the role of observable characteristics in explaining the annualized wealth differences between natives and immigrants. The regression estimates indicate that recent immigrants accumulate less wealth than do those in earlier cohorts. As we add covariates for demographics, lifecycle variables, transfers, and origin and race/ethnicity, however, the gaps begin to close, suggesting that age at arrival is unlikely to be the sole (or perhaps even the major) driver of annualized wealth.28 If that were the case, then we would expect most of these differences to survive the layering of covariates. Thus, although we do not have enough information to answer the question definitively, it seems fair to suggest that both factors—who came, and when they came—likely play an important role.
Convergence of Immigrant and Native Wealth
A central question in the labor literature is whether immigrant earnings tend to converge with those of natives having similar characteristics. If saving rates and asset allocation were held constant, convergence in earnings would imply convergence in retirement resources. Saving, however, involves a complex relationship between earnings, financial investments, and homeownership. Therefore, convergence in earnings does not necessarily imply convergence in annualized wealth if saving and investment behavior differ widely between immigrants and natives and across immigrant arrival cohorts.
The results in Table 5 suggest that the annualized resources of earlier immigrant waves are statistically indistinguishable from those of natives with similar characteristics. More recent immigrant cohorts, by contrast, appear to accumulate substantially less wealth heading into retirement. However, that conclusion implicitly assumes that immigrants and natives experience similar returns to household characteristics such as family composition, financial variables, and health events.
It is possible that the negative coefficient estimates for the more recent immigrant cohorts partially reflect differences in the returns to characteristics between immigrants and natives. If so, we would expect some of the wealth gradient by immigration wave to disappear if we regressed annualized wealth on the covariates of immigrants alone. Like Table 5, Table 6 shows estimates for cumulating sets of covariates, but the sample is limited to immigrant households (either single respondents or married couples in which both spouses are immigrants). Table 6 omits the pre-1955 immigration cohort.
Variable | Baseline covariates | With demographic covariates added | With lifecycle covariates added | With transfer-payment covariates added | With immigrant origin and race/ethnicity covariates added | |||||
---|---|---|---|---|---|---|---|---|---|---|
Coefficient | Standard error | Coefficient | Standard error | Coefficient | Standard error | Coefficient | Standard error | Coefficient | Standard error | |
Earnings data sample | ||||||||||
Immigration cohort | ||||||||||
1955–1964 | 0.200* | 0.111 | 0.052 | 0.091 | 0.003 | 0.059 | -0.033 | 0.055 | -0.030 | 0.049 |
1965–1974 | -0.202** | 0.096 | -0.222*** | 0.070 | -0.098** | 0.048 | -0.116** | 0.048 | -0.076* | 0.041 |
1975–1984 | -0.534*** | 0.088 | -0.399*** | 0.070 | -0.208*** | 0.053 | -0.217*** | 0.050 | -0.186*** | 0.046 |
1985 or later | -0.819*** | 0.090 | -0.767*** | 0.087 | -0.205*** | 0.077 | -0.247*** | 0.077 | -0.225*** | 0.070 |
Constant | 9.909*** | 0.068 | 9.544*** | 0.074 | 7.396*** | 0.276 | 7.784*** | 0.278 | 7.946*** | 0.314 |
R-squared | 0.105 | 0.323 | 0.490 | 0.562 | 0.606 | |||||
Observations | 9,135 | 8,985 | 6,206 | 5,087 | 5,087 | |||||
Geocoded data sample | ||||||||||
Immigration cohort | ||||||||||
1955–1964 | 0.200* | 0.111 | 0.052 | 0.091 | 0.070 | 0.052 | 0.022 | 0.043 | 0.054 | 0.046 |
1965–1974 | -0.202** | 0.096 | -0.222*** | 0.070 | -0.129*** | 0.042 | -0.138*** | 0.043 | -0.069 | 0.050 |
1975–1984 | -0.534*** | 0.088 | -0.399*** | 0.070 | -0.250*** | 0.042 | -0.256*** | 0.043 | -0.196*** | 0.054 |
1985 or later | -0.819*** | 0.090 | -0.767*** | 0.087 | -0.440*** | 0.062 | -0.496*** | 0.068 | -0.447*** | 0.082 |
Constant | 9.909*** | 0.068 | 9.544*** | 0.074 | 9.166*** | 0.053 | 9.176*** | 0.054 | 9.396*** | 0.071 |
R-squared | 0.105 | 0.323 | 0.430 | 0.513 | 0.550 | |||||
Observations | 9,135 | 8,985 | 8,985 | 7,175 | 7,175 | |||||
SOURCE: Authors' calculations based on HRS (1998–2012 waves), SSA earnings records, and geocoded data from HRS. | ||||||||||
NOTES: An immigrant household comprises either a single immigrant or a married immigrant couple.
All covariate category specifications include a full set of year dummies.
Standard errors are clustered at the household level.
* = statistically significant at the p < 0.10 level; ** = statistically significant at the p < 0.05 level; *** = statistically significant at the p < 0.01 level.
|
The coefficient estimates show a similar pattern of decreasing annualized wealth across increasingly recent cohorts.29 The one exception to the pattern is the 1955–1964 cohort, which appears to have statistically more annualized wealth than the previous cohort. This pattern of declining annualized wealth across the three most recent immigration cohorts holds in all specifications and both restricted data samples with one slight exception (lifecycle variables in the earnings-data sample for the 1985 or later cohort). The 1975–1984 cohort holds about 17 percent less annualized wealth than the earliest cohort, while the resources of those in the most recent wave are between 20 percent and 36 percent lower, depending on the restricted sample used.30
Characteristics Versus Returns
The results in Tables 5 and 6 indicate that much of the raw gap in annualized wealth between different immigrant cohorts and natives is due to differences either in observables or in the returns to those observables. In this section, we explore some key differences in characteristics that a standard lifecycle model would suggest should matter for total wealth accumulation, and we analyze the raw gap in annualized wealth using Melly's (2005) quantile version of the standard Oaxaca-Blinder decomposition.
Housing wealth, health shocks, and bequests/transfers may affect observed patterns of annualized wealth. Tables 7, 8, and 9 highlight some systemic differences in these characteristics between natives and different waves of immigrants. The tables show both the unconditional summary statistics for each variable and the coefficient estimates that result from regressing each of those variables on immigrant-cohort dummies, controlling for a large number of individual characteristics.31
Table 7 shows that the earliest and the most recent immigration cohorts have the lowest homeownership rates after controlling for observable characteristics. That result makes sense for immigrants in the most recent cohort, given that they have had less time to accumulate money for a down payment or face credit constraints because of lower incomes; but for the earliest cohort, that result is more of a mystery. It may reflect members of the older cohort moving in with their children or into institutionalized care.
Variable | Homeownership rate | Home equity/net worth a ratio | Mortgage-holding rate b | Mortgage/home value ratio c | ||||||||
---|---|---|---|---|---|---|---|---|---|---|---|---|
Mean | Probit regressions | Median | Quantile regressions | Mean | Probit regressions | Mean | Quantile regressions | |||||
Coefficient | Standard error | Coefficient | Standard error | Coefficient | Standard error | Coefficient | Standard error | |||||
Native | 0.76 | . . . | . . . | 0.51 | . . . | . . . | 0.42 | . . . | . . . | 0.39 | . . . | . . . |
Immigration cohort | ||||||||||||
Pre-1955 | 0.64 | -0.255*** | 0.069 | 0.68 | 0.032*** | 0.011 | 0.28 | -0.042 | 0.084 | 0.36 | -0.019 | 0.033 |
1955–1964 | 0.72 | -0.119* | 0.068 | 0.64 | 0.042*** | 0.016 | 0.45 | 0.143* | 0.074 | 0.36 | -0.024 | 0.026 |
1965–1974 | 0.64 | -0.149** | 0.065 | 0.83 | 0.031* | 0.016 | 0.53 | 0.186** | 0.075 | 0.40 | -0.053*** | 0.020 |
1975–1984 | 0.60 | -0.089 | 0.070 | 0.82 | -0.020 | 0.018 | 0.59 | 0.274*** | 0.080 | 0.50 | -0.025 | 0.032 |
1985 or later | 0.46 | -0.223** | 0.091 | 0.79 | -0.026 | 0.027 | 0.54 | -0.053 | 0.095 | 0.60 | 0.042 | 0.037 |
SOURCE: Authors' calculations based on HRS (1998–2012 waves). | ||||||||||||
NOTES: Regressions include controls for age, marital status, separate indicators of whether an immigrant is married to an immigrant or a native, non-English speaking status, education, Hispanic origin, nonwhite race, family size, number of children, the natural logarithm of annualized comprehensive wealth, and a full set of year dummies.
Standard errors are clustered at the household level.
. . . = not applicable.
* = statistically significant at the p < 0.10 level; ** = statistically significant at the p < 0.05 level; *** = statistically significant at the p < 0.01 level.
|
||||||||||||
a. Net worth of combined financial and nonfinancial wealth (annuitized wealth omitted). | ||||||||||||
b. Among homeowners. | ||||||||||||
c. Among mortgage-holders. |
Table 7 also confirms that immigrants are less likely to own a house than natives are, yet median home equity among immigrants who are homeowners constitutes a greater share of their net worth—at least among the earliest three waves. The two most recent immigrant waves do not hold higher portions of wealth in housing after controlling for other characteristics. This could partly explain their lower levels of annualized wealth in retirement, because drawdowns of housing wealth tend to be lower than those of other wealth holdings.
Table 8 shows some significant differences between natives and immigrants in medical costs, business ownership, bequest intentions, and expected longevity. Immigrants are less likely than natives to incur out-of-pocket medical costs, and conditional on having positive costs, only the pre-1955 immigration cohort has a significant difference in the cost level. Immigrants are less likely than natives to own their own businesses, although the differences in the ratios of business valuation to net worth are insignificant for all but the most recent wave of immigrants. Interestingly, there are strong differences in bequest intentions. With the exception of the earliest wave, immigrants report considerably higher probabilities of bequeathing large amounts than do natives with similar characteristics. There is no consistent pattern of immigrant-native differences in expected longevity.
Variable | Presence of any out-of-pocket medical costs | Amount of out-of-pocket medical costs a | Business ownership rate | Business valuation/ net worth b ratio | Probability of bequeathing more than $10,000 | Probability of bequeathing more than $100,000 | Ratio of own survival expectancy to SSA life table projection | ||||||||||||||
---|---|---|---|---|---|---|---|---|---|---|---|---|---|---|---|---|---|---|---|---|---|
Mean | Probit regressions | Median ($) | Quantile regressions | Mean | Probit regressions | Median | Quantile regressions | Mean (%) | OLS regressions | Mean (%) | OLS regressions | Median | OLS regressions | ||||||||
Coefficient | Standard error | Coefficient | Standard error | Coefficient | Standard error | Coefficient | Standard error | Coefficient | Standard error | Coefficient | Standard error | Coefficient | Standard error | ||||||||
Native | 0.909 | . . . | . . . | 2,400 | . . . | . . . | 0.086 | . . . | . . . | 0.289 | . . . | . . . | 66.61 | . . . | . . . | 42.52 | . . . | . . . | 1.086 | . . . | . . . |
Immigration cohort | |||||||||||||||||||||
Pre-1955 | 0.824 | -0.181*** | 0.058 | 2,500 | 272.013* | 144.134 | 0.027 | -0.364*** | 0.118 | 0.284 | -0.009 | 0.034 | 59.63 | -2.424* | 1.347 | 37.31 | 0.166 | 1.306 | 1.185 | 0.176* | 0.096 |
1955–1964 | 0.844 | -0.141** | 0.058 | 2,160 | 125.526 | 119.174 | 0.050 | -0.214** | 0.092 | 0.219 | -0.020 | 0.035 | 62.39 | -2.288 | 1.479 | 43.39 | 2.841** | 1.446 | 1.074 | -0.114** | 0.049 |
1965–1974 | 0.795 | -0.155*** | 0.057 | 2,160 | 122.403 | 121.022 | 0.021 | -0.362*** | 0.109 | 0.265 | -0.037 | 0.050 | 51.15 | -2.077 | 1.434 | 31.74 | 3.460** | 1.378 | 0.962 | -0.127*** | 0.039 |
1975–1984 | 0.773 | -0.150*** | 0.058 | 1,790 | -39.083 | 122.120 | 0.047 | 0.098 | 0.103 | 0.313 | 0.109 | 0.075 | 45.97 | -2.103 | 1.545 | 27.39 | 4.645*** | 1.401 | 0.996 | -0.055 | 0.052 |
1985 or later | 0.799 | 0.089 | 0.073 | 1,660 | -73.137 | 154.442 | 0.039 | 0.089 | 0.147 | 0.376 | 0.213** | 0.092 | 42.77 | 3.991* | 2.076 | 25.51 | 14.180*** | 1.999 | 0.985 | -0.002 | 0.057 |
SOURCE: Authors' calculations based on HRS (1998–2012 waves). | |||||||||||||||||||||
NOTES: Regressions include controls for age, marital status, separate indicators of whether an immigrant is married to an immigrant or a native, non-English speaking status, education, Hispanic origin, nonwhite race, family size, number of children, the natural logarithm of annualized comprehensive wealth, and a full set of year dummies.
Standard errors are clustered at the household level.
. . . = not applicable.
* = statistically significant at the p < 0.10 level; ** = statistically significant at the p < 0.05 level; *** = statistically significant at the p < 0.01 level.
|
|||||||||||||||||||||
a. Among respondents with costs. | |||||||||||||||||||||
b. Net worth of combined financial and nonfinancial wealth (annuitized wealth omitted). |
Family transfers likely play an important role in the saving decisions of immigrants (Table 9). Although the HRS does not collect information on specific remittance amounts, it does provide information on the presence of transfers totaling $500 or more to and from family members. Immigrants are more likely to report transfers to their children and other relatives. The fact that they are also much less likely to live near their children suggests that some of these transfers may be flowing abroad. Among immigrants, the most recent wave differs most widely from natives with similar characteristics in terms of reported transfers, suggesting both a reason for their lower annualized wealth (outbound transfers) and a cause for concern about the adequacy of their resources (if current practice predicts future transfers).
Variable | Number of people in household beyond respondent (and spouse, if any) | Whether any child(ren) living outside the household but within 10 miles | Whether providing financial assistance a to child(ren) | Whether receiving financial assistance a from child(ren) | Whether providing financial assistance a to relatives other than child(ren) | Whether receiving financial assistance a from relatives other than child(ren) | ||||||||||||
---|---|---|---|---|---|---|---|---|---|---|---|---|---|---|---|---|---|---|
Mean | OLS regressions | Mean | Probit regressions | Mean | Probit regressions | Mean | Probit regressions | Mean | Probit regressions | Mean | Probit regressions | |||||||
Coefficient | Standard error | Coefficient | Standard error | Coefficient | Standard error | Coefficient | Standard error | Coefficient | Standard error | Coefficient | Standard error | |||||||
Native | 0.547 | . . . | . . . | 0.589 | . . . | . . . | 0.361 | . . . | . . . | 0.058 | . . . | . . . | 0.083 | . . . | . . . | 0.029 | . . . | . . . |
Immigration cohort | ||||||||||||||||||
Pre-1955 | 0.627 | 0.015** | 0.007 | 0.551 | -0.142** | 0.059 | 0.309 | 0.137*** | 0.051 | 0.077 | 0.074 | 0.068 | 0.074 | 0.091 | 0.067 | 0.024 | 0.114 | 0.102 |
1955–1964 | 0.891 | 0.019** | 0.009 | 0.561 | -0.066 | 0.063 | 0.336 | 0.089 | 0.056 | 0.087 | 0.158** | 0.072 | 0.103 | 0.075 | 0.066 | 0.017 | -0.143* | 0.083 |
1965–1974 | 1.288 | 0.043*** | 0.009 | 0.562 | -0.144** | 0.059 | 0.315 | 0.168*** | 0.051 | 0.107 | 0.154** | 0.062 | 0.122 | 0.265*** | 0.060 | 0.022 | -0.060 | 0.085 |
1975–1984 | 1.531 | 0.052*** | 0.008 | 0.579 | -0.173*** | 0.064 | 0.265 | 0.076 | 0.054 | 0.116 | 0.143** | 0.066 | 0.146 | 0.409*** | 0.064 | 0.028 | -0.026 | 0.086 |
1985 or later | 1.791 | 0.041*** | 0.011 | 0.424 | -0.486*** | 0.077 | 0.330 | 0.357*** | 0.065 | 0.113 | 0.013 | 0.074 | 0.146 | 0.464*** | 0.075 | 0.023 | -0.331*** | 0.107 |
SOURCE: Authors' calculations based on HRS (1998–2012 waves). | ||||||||||||||||||
NOTES: Regressions include controls for age, marital status, separate indicators of whether an immigrant is married to an immigrant or a native, non-English speaking status, education, Hispanic origin, nonwhite race, family size, number of children, the natural logarithm of annualized comprehensive wealth, and a full set of year dummies.
Standard errors are clustered at the household level.
. . . = not applicable.
* = statistically significant at the p < 0.10 level; ** = statistically significant at the p < 0.05 level; *** = statistically significant at the p < 0.01 level.
|
||||||||||||||||||
a. $500 or more. |
Taken together, the results in Tables 7, 8, and 9 indicate that there are large differences in financial characteristics and behaviors between natives and successive waves of immigrants. If the returns to these characteristics were the same across groups, these differences would account for the decrease in the annualized wealth gaps that appear with each additional layer of controls in the regressions in Tables 5 and 6. Another possibility, however, is that groups have experienced different returns to these characteristics, so that education (for example) may be more or less important for some groups than it is for others.
We examine this possibility by decomposing the raw annualized wealth differences using the approach described in Melly (2005). Broadly, the procedure first approximates the conditional distribution by estimating a set of n quantile regressions. With the conditional distribution in hand, we can obtain the unconditional distribution by integrating the conditional distribution over the independent variables. Importantly, the procedure can provide counterfactual unconditional distributions. In our case, we are interested in counterfactual distributions of median annualized wealth that would arise if natives had the same quantile function (that is, the same coefficients) as different waves of immigrants. With these counterfactual distributions, we can decompose the raw differences in annualized wealth into parts explained either by different distributions of observables or by different returns to those observables.
Table 10 shows the results of the decompositions for each immigration cohort, as estimated using the baseline, demographic, lifecycle, and transfer covariates listed in Table 4. We omit the geographic and immigrant origin and race/ethnicity covariates because they pertain only to immigrants. Across cohorts, most of the raw differences in annualized wealth are attributable to differences in characteristics. This is not too surprising in light of the large differences in financial characteristics and behaviors between immigrants and natives shown in Tables 7–9. The full regression results in Appendix B make clear that the characteristics that most explain the gaps in annualized wealth are financial (relative shares of financial and nonfinancial wealth, home value, and business valuation), medical (out-of-pocket costs and self-reported health), and demographic (race, ethnicity, and per capita income of country of origin).
Immigration cohort | Raw difference | Standard error | Effect of observable characteristics | Standard error | Effect of returns to characteristics | Standard error |
---|---|---|---|---|---|---|
Pre-1955 | -0.167 | 0.036 | -0.328 | 0.045 | 0.161 | 0.003 |
1955–1964 | -0.248 | 0.029 | -0.368 | 0.037 | 0.120 | 0.005 |
1965–1974 | -0.597 | 0.031 | -0.647 | 0.036 | 0.050 | 0.003 |
1975–1984 | -0.973 | 0.027 | -0.827 | 0.044 | -0.145 | 0.004 |
1985 or later | -1.085 | 0.042 | -0.786 | 0.042 | -0.299 | 0.004 |
SOURCE: Authors' calculations based on HRS (1998–2012 waves) using the method described in Melly (2005). | ||||||
NOTE: Values are quantile regressions estimated using the restricted earnings sample with controls for demographic, lifecycle, and transfer-payment covariates and a full set of year dummies. Geographic and immigrant origin and race/ethnicity covariates are omitted because they pertain only to immigrants. |
The pattern for returns to characteristics, however, is more interesting. Among the earliest waves of immigrants, the returns to characteristics close some of the raw gap in annualized wealth. With the most recent waves, however, this pattern reverses, and the returns to characteristics are negative. The most recent immigrant waves therefore appear to be falling behind in retirement preparation both because of characteristics such as education and lifetime earnings and because of the returns to those characteristics. For the most recent immigration cohort, 72 percent (−0.786 ⁄ −1.085) of the raw difference can be explained by characteristics, and 28 percent (−0.299 ⁄ −1.085) can be explained by returns.
Conclusion and Directions for Future Research
This article compares the total resources available to immigrants and natives in retirement. Although we find that immigrants have less wealth overall than natives, they appear to decumulate resources more slowly in retirement. Consistent with the literature on lifecycle wealth accumulation, we find some evidence that these patterns may be due to differential concentrations of illiquid wealth and use of bequests and transfers. Compared with natives, immigrant homeowners have higher shares of net worth in home equity and report being more likely to leave a bequest and make transfers to children and relatives. Each of these tendencies is likely to slow wealth decumulation. The concentration of wealth in housing is a factor if households are reluctant to tap into housing wealth, and bequests provide a stronger incentive to preserve wealth in retirement.
Our findings suggest that immigrants in general are relatively well situated in retirement, but that recent immigrants have low levels of total resources and are likely to have difficulty maintaining adequate levels of spending in retirement. In this sense, our sample of households may signify an important transition for the retirement well-being of immigrants. The patterns of immigrant retirement wealth will soon reflect the effects of the dramatic change in the composition of immigrants following the 1965 immigration reform. Our findings suggest that some of the newer immigrants, who may be better off than if they had remained in their countries of origin, are nonetheless likely to be particularly vulnerable, facing retirement with a combination of low Social Security benefits, low private pension coverage, and insufficient financial and nonfinancial wealth. Improving financial literacy and access to banking services could help narrow these gaps.
With these findings come caveats that also suggest topics for future research. First, because Social Security benefits are among the most important sources of retirement wealth for both immigrants and natives, a better understanding of the accumulation patterns by years of covered earnings would be useful. Although recent immigrants have fewer years of covered earnings, the progressive benefit calculation formula provides low lifetime earners with a higher replacement rate than high earners receive (Gustman and Steinmeier 2000). The extent to which this issue mitigates the disadvantage we find for recent immigrants is worth exploring. In addition, immigrants may be more likely than natives to work at older ages; Borjas (2011), for example, linked the retirement behavior of immigrants to their insured status for Social Security retirement benefits. Moreover, if the lower initial earnings of recent versus earlier immigrant cohorts reflect lower skill transferability (Duleep and Regets 1997), then one would expect recent immigrants to work longer than natives (or earlier immigrant entrants) to maximize the return on their greater human capital investment.
Immigrants might also have access to other resources that our measure of comprehensive wealth does not capture. Relative to natives, aged immigrants may receive more transfers from their children and other family members, which could reduce their financial vulnerability at retirement. Foreign assets could also reduce their vulnerability. Although HRS wealth questions aim to capture all components of total wealth, future research might assess whether HRS respondents underreport foreign assets. Finally, the possibility of return migration may mitigate the disadvantage in retirement resources faced by the most recent cohorts. Estimates of return migration range from about 15 percent to 30 percent (Borjas and Bratsberg 1996; Mayr and Peri 2008), with recent immigrants and those who immigrated at older ages tending to be more likely to return (Duleep 1994).
Future research could help to identify the extent to which low wealth, as measured in the HRS, corresponds with lower living standards in retirement. Differences by years in the United States also bear further examination. Although we could not isolate the effect of years in the United States from the cohort effect associated with the year of arrival, year of U.S. entry can be proxied with administrative records on first reported earnings (Duleep and Dowhan 2002). Using such information, a researcher could explore how much of the wealth gap between recent immigrants and natives is explained by years in the United States rather than a straight cohort effect linked to year of arrival.
Appendix A
Described below are our methodologies for constructing the comprehensive and annualized wealth measures.
Comprehensive Wealth
Comprehensive wealth combines current-market valuations for some components, such as 401(k) plans, with the actuarial present value of future cash flows, such Social Security benefits. We convert the current-wealth component values into 2012 dollars and add them together. To calculate future cash flows, we convert each reported income stream—Social Security, Supplemental Security Income, and any of the scores of annuity and pension types listed in the HRS—to present values for each respondent. Below we present the formulas for Social Security benefits and for other streams of payments.
Social Security. Let denote the respondent's probability of surviving until age t (conditional on being alive in period t−1), and denote the corresponding survival probability for the respondent's spouse. The formula for computing the present value of Social Security benefits is given by
where i is the nominal interest rate and and denote the benefit amounts at age t for the respondent and spouse, respectively. The first term in brackets accounts for the household's receipt of both the respondent's and the spouse's benefits when both are alive, and the second term reflects the surviving spouse's widow(er) benefits. If either partner dies, the survivor will receive the larger of the two benefit amounts.
Pension benefits and other payments. The present-value calculation for defined-benefit pensions, veteran's benefits, earnings to age 65, annuities, and other nonlabor income follows a similar procedure, except that we compute the present values separately for the respondent and the spouse, and we include a COLA and spousal benefits only if respondents report them in the survey. The formula for computing the present value of these annualized payments is given by
where is the fraction of the payment remaining as a survivor's benefit. Note that the survivor's term in this equation differs from that of the Social Security formula because we compute the respondent's payments and not combined household payments.
Annualized Wealth
After computing the values of comprehensive wealth and its components, we convert those amounts to an annualized equivalent by determining how much one would have to pay for an actuarially fair, inflation-adjusted joint-life annuity that pays an equivalence of $α when both members of the household are living and $1 otherwise. The price of such an annuity is given by
where the survival probabilities again reflect differential mortality by education, race, ethnicity, and sex, as described in the article's “Data” section under “Estimating Annualized Wealth.” The annualizing factors, , are household- and age-specific and equal to the reciprocal of the annuity price: .
The final step in computing annualized comprehensive wealth is simply to multiply the annualizing factors, , by the value of comprehensive wealth for each household. The result enables an approximate translation of total resources into an amount that households could spend each year, as if they were able to fully annuitize their current and future wealth.
Appendix B
Variable | Baseline covariates | With demographic covariates added | With lifecycle covariates added | With transfer-payment covariates added | With immigrant origin and race/ethnicity covariates added | |||||
---|---|---|---|---|---|---|---|---|---|---|
Coefficient | Standard error | Coefficient | Standard error | Coefficient | Standard error | Coefficient | Standard error | Coefficient | Standard error | |
Immigration cohort | ||||||||||
Pre-1955 | -0.222* | 0.124 | -0.053 | 0.073 | 0.051 | 0.040 | 0.050 | 0.056 | 0.105** | 0.041 |
1955–1964 | -0.147** | 0.066 | 0.025 | 0.058 | 0.092** | 0.042 | 0.063 | 0.039 | 0.125*** | 0.045 |
1965–1974 | -0.626*** | 0.071 | -0.147*** | 0.047 | -0.029 | 0.036 | -0.034 | 0.040 | 0.045 | 0.039 |
1975–1984 | -0.932*** | 0.061 | -0.311*** | 0.045 | -0.145*** | 0.040 | -0.162*** | 0.039 | -0.080 | 0.049 |
1985 or later | -1.246*** | 0.070 | -0.662*** | 0.073 | -0.186*** | 0.052 | -0.237*** | 0.078 | -0.169** | 0.074 |
Age | ||||||||||
65–74 | 0.084*** | 0.013 | 0.147*** | 0.010 | 0.023** | 0.009 | 0.051*** | 0.008 | 0.051*** | 0.008 |
75 or older | 0.189*** | 0.018 | 0.326*** | 0.014 | 0.131*** | 0.015 | 0.176*** | 0.012 | 0.178*** | 0.012 |
Married couple | . . . | . . . | 0.288*** | 0.013 | 0.029** | 0.012 | 0.012 | 0.011 | 0.012 | 0.011 |
Immigrant and immigrant | . . . | . . . | 0.038 | 0.038 | -0.069** | 0.031 | -0.063** | 0.032 | -0.055* | 0.031 |
Immigrant and native | . . . | . . . | 0.021 | 0.035 | 0.010 | 0.033 | -0.005 | 0.029 | -0.017 | 0.027 |
Naturalized citizen | . . . | . . . | 0.077* | 0.043 | 0.011 | 0.030 | 0.004 | 0.033 | 0.000 | 0.030 |
Non-English speaker | . . . | . . . | -0.178*** | 0.041 | -0.098*** | 0.030 | -0.100** | 0.043 | -0.075* | 0.039 |
Education | ||||||||||
High school diploma | . . . | . . . | 0.460*** | 0.016 | 0.188*** | 0.014 | 0.146*** | 0.011 | 0.146*** | 0.012 |
College degree | . . . | . . . | 0.987*** | 0.019 | 0.492*** | 0.021 | 0.392*** | 0.018 | 0.391*** | 0.017 |
Race/ethnicity | ||||||||||
Hispanic— | ||||||||||
White | . . . | . . . | -0.502*** | 0.031 | -0.239*** | 0.023 | -0.224*** | 0.021 | -0.201*** | 0.026 |
Nonwhite | . . . | . . . | -0.486*** | 0.038 | -0.191*** | 0.026 | -0.169*** | 0.030 | -0.126*** | 0.032 |
Nonwhite non-Hispanic | . . . | . . . | -0.360*** | 0.016 | -0.081*** | 0.016 | -0.075*** | 0.013 | -0.074*** | 0.014 |
Family size | . . . | . . . | -0.031*** | 0.004 | -0.001 | 0.004 | -0.004 | 0.003 | -0.004 | 0.003 |
Number of children | . . . | . . . | -0.023*** | 0.003 | -0.009*** | 0.002 | -0.007*** | 0.002 | -0.007*** | 0.002 |
Share of wealth from— | ||||||||||
Financial assets | . . . | . . . | . . . | . . . | 0.019*** | 0.001 | 0.015*** | 0.001 | 0.015*** | 0.001 |
Nonfinancial assets | . . . | . . . | . . . | . . . | 0.008*** | 0.001 | 0.007*** | 0.001 | 0.007*** | 0.001 |
Logarithm of average household earnings | . . . | . . . | . . . | . . . | 0.099*** | 0.005 | 0.077*** | 0.005 | 0.076*** | 0.005 |
Standard deviation of logarithm of average household earnings | . . . | . . . | . . . | . . . | 0.038** | 0.016 | 0.014 | 0.014 | 0.014 | 0.014 |
Homeowner | . . . | . . . | . . . | . . . | 0.297*** | 0.017 | 0.213*** | 0.014 | 0.213*** | 0.014 |
Business owner | . . . | . . . | . . . | . . . | 0.130*** | 0.018 | 0.076*** | 0.017 | 0.077*** | 0.017 |
Health status | ||||||||||
Good | . . . | . . . | . . . | . . . | -0.058*** | 0.009 | -0.037*** | 0.008 | -0.037*** | 0.008 |
Fair or poor | . . . | . . . | . . . | . . . | -0.154*** | 0.011 | -0.117*** | 0.009 | -0.117*** | 0.009 |
Medical out-of-pocket costs | ||||||||||
Middle tercile | . . . | . . . | . . . | . . . | 0.069*** | 0.007 | 0.061*** | 0.007 | 0.062*** | 0.007 |
Highest tercile | . . . | . . . | . . . | . . . | 0.068*** | 0.009 | 0.063*** | 0.009 | 0.064*** | 0.009 |
Expect to leave bequest | ||||||||||
Deemed likely, greater than $10,000 | . . . | . . . | . . . | . . . | . . . | . . . | 0.088*** | 0.008 | 0.087*** | 0.008 |
Deemed likely, greater than $100,000 | . . . | . . . | . . . | . . . | . . . | . . . | 0.250*** | 0.011 | 0.250*** | 0.011 |
Child(ren) within 10 miles | . . . | . . . | . . . | . . . | . . . | . . . | -0.013* | 0.008 | -0.012 | 0.008 |
Transfers | ||||||||||
To child(ren) | . . . | . . . | . . . | . . . | . . . | . . . | 0.152*** | 0.007 | 0.150*** | 0.007 |
From child(ren) | . . . | . . . | . . . | . . . | . . . | . . . | -0.067*** | 0.012 | -0.070*** | 0.012 |
To relative(s) | . . . | . . . | . . . | . . . | . . . | . . . | 0.122*** | 0.013 | 0.124*** | 0.013 |
From relative(s) | . . . | . . . | . . . | . . . | . . . | . . . | -0.091*** | 0.020 | -0.091*** | 0.020 |
Immigrant race/ethnicity | ||||||||||
Hispanic— | ||||||||||
White | . . . | . . . | . . . | . . . | . . . | . . . | . . . | . . . | -0.161*** | 0.050 |
Nonwhite | . . . | . . . | . . . | . . . | . . . | . . . | . . . | . . . | -0.112** | 0.045 |
Nonwhite non-Hispanic | . . . | . . . | . . . | . . . | . . . | . . . | . . . | . . . | -0.075 | 0.047 |
Constant | 10.342*** | 0.010 | 9.917*** | 0.020 | 8.137*** | 0.072 | 8.427*** | 0.071 | 8.431* | 0.071 |
R-squared | 0.066 | 0.348 | 0.381 | 0.526 | 0.526 | |||||
Observations | 105,268 | 103,289 | 81,138 | 69,055 | 69,055 | |||||
SOURCE: Authors' calculations based on HRS (1998–2012 waves) and SSA earnings records. | ||||||||||
NOTES: . . . = not applicable.
* = statistically significant at the p < 0.10 level; ** = statistically significant at the p < 0.05 level; *** = statistically significant at the p < 0.01 level.
|
Variable | Baseline covariates | With demographic covariates added | With lifecycle covariates added | With transfer-payment covariates added | With immigrant origin and race/ethnicity covariates added | |||||
---|---|---|---|---|---|---|---|---|---|---|
Coefficient | Standard error | Coefficient | Standard error | Coefficient | Standard error | Coefficient | Standard error | Coefficient | Standard error | |
Immigration cohort | ||||||||||
Pre-1955 | -0.222* | 0.124 | -0.053 | 0.073 | 0.000 | 0.031 | 0.017 | 0.034 | 0.061 | 0.040 |
1955–1964 | -0.147** | 0.066 | 0.025 | 0.058 | 0.065 | 0.041 | 0.053 | 0.037 | 0.109*** | 0.042 |
1965–1974 | -0.626*** | 0.071 | -0.147*** | 0.047 | -0.094** | 0.039 | -0.078* | 0.041 | -0.001 | 0.045 |
1975–1984 | -0.932*** | 0.061 | -0.311*** | 0.045 | -0.204*** | 0.035 | -0.216*** | 0.043 | -0.126** | 0.050 |
1985 or later | -1.246*** | 0.070 | -0.662*** | 0.073 | -0.414*** | 0.053 | -0.464*** | 0.060 | -0.355*** | 0.094 |
Age | ||||||||||
70–79 | 0.084*** | 0.013 | 0.147*** | 0.010 | 0.029*** | 0.008 | 0.058*** | 0.008 | 0.056* | 0.008 |
80 or older | 0.189*** | 0.018 | 0.326*** | 0.014 | 0.118*** | 0.012 | 0.178*** | 0.013 | 0.178*** | 0.013 |
Married couple | . . . | . . . | 0.288*** | 0.013 | 0.120*** | 0.010 | 0.084*** | 0.009 | 0.084*** | 0.009 |
Immigrant and immigrant | . . . | . . . | 0.038 | 0.038 | -0.066** | 0.031 | -0.056** | 0.029 | -0.047 | 0.032 |
Immigrant and native | . . . | . . . | 0.021 | 0.035 | -0.013 | 0.027 | -0.016 | 0.027 | -0.016 | 0.026 |
Naturalized citizen | . . . | . . . | 0.077* | 0.043 | 0.032 | 0.027 | 0.000 | 0.029 | 0.010 | 0.030 |
Non-English speaker | . . . | . . . | -0.178*** | 0.041 | -0.180*** | 0.029 | -0.149*** | 0.034 | -0.148*** | 0.027 |
Education | ||||||||||
High school diploma | . . . | . . . | 0.460*** | 0.016 | 0.227*** | 0.011 | 0.169*** | 0.010 | 0.168*** | 0.010 |
College degree | . . . | . . . | 0.987*** | 0.019 | 0.535*** | 0.018 | 0.418*** | 0.017 | 0.417*** | 0.017 |
Race/ethnicity | ||||||||||
Hispanic— | ||||||||||
White | . . . | . . . | -0.502*** | 0.031 | -0.262*** | 0.021 | -0.254*** | 0.020 | -0.226*** | 0.020 |
Nonwhite | . . . | . . . | -0.486*** | 0.038 | -0.223*** | 0.027 | -0.224*** | 0.025 | -0.208*** | 0.028 |
Nonwhite non-Hispanic | . . . | . . . | -0.360*** | 0.016 | -0.132*** | 0.013 | -0.111*** | 0.012 | -0.109*** | 0.012 |
Family size | . . . | . . . | -0.030*** | 0.004 | -0.007** | 0.003 | -0.009*** | 0.003 | -0.009*** | 0.003 |
Number of children | . . . | . . . | -0.023*** | 0.003 | -0.009*** | 0.002 | -0.007*** | 0.002 | -0.007*** | 0.002 |
Share of wealth from— | ||||||||||
Financial assets | . . . | . . . | . . . | . . . | 0.018*** | 0.001 | 0.014*** | 0.001 | 0.014*** | 0.001 |
Nonfinancial assets | . . . | . . . | . . . | . . . | 0.007*** | 0.001 | 0.006*** | 0.000 | 0.007*** | 0.000 |
Urban residence | . . . | . . . | . . . | . . . | 0.162*** | 0.008 | 0.124*** | 0.008 | 0.120*** | 0.008 |
Homeowner | . . . | . . . | . . . | . . . | 0.375*** | 0.015 | 0.277*** | 0.013 | 0.276*** | 0.013 |
Business owner | . . . | . . . | . . . | . . . | 0.182*** | 0.018 | 0.113*** | 0.017 | 0.112*** | 0.017 |
Health status | ||||||||||
Good | . . . | . . . | . . . | . . . | -0.069*** | 0.008 | -0.045*** | 0.007 | -0.045*** | 0.007 |
Fair or poor | . . . | . . . | . . . | . . . | -0.168*** | 0.010 | -0.121*** | 0.009 | -0.121*** | 0.009 |
Medical out-of-pocket costs | ||||||||||
Middle tercile | . . . | . . . | . . . | . . . | 0.099*** | 0.007 | 0.074*** | 0.006 | 0.074*** | 0.006 |
Highest tercile | . . . | . . . | . . . | . . . | 0.102*** | 0.008 | 0.089*** | 0.008 | 0.088*** | 0.008 |
Expect to leave bequest | ||||||||||
Deemed likely, greater than $10,000 | . . . | . . . | . . . | . . . | . . . | . . . | 0.093*** | 0.008 | 0.094*** | 0.008 |
Deemed likely, greater than $100,000 | . . . | . . . | . . . | . . . | . . . | . . . | 0.257*** | 0.012 | 0.256*** | 0.012 |
Child(ren) within 10 miles | . . . | . . . | . . . | . . . | . . . | . . . | -0.016** | 0.007 | -0.016** | 0.007 |
Transfers | ||||||||||
To child(ren) | . . . | . . . | . . . | . . . | . . . | . . . | 0.159*** | 0.007 | 0.158*** | 0.007 |
From child(ren) | . . . | . . . | . . . | . . . | . . . | . . . | -0.077*** | 0.010 | -0.080*** | 0.010 |
To relative(s) | . . . | . . . | . . . | . . . | . . . | . . . | 0.134*** | 0.011 | 0.133*** | 0.011 |
From relative(s) | . . . | . . . | . . . | . . . | . . . | . . . | -0.101*** | 0.017 | -0.101*** | 0.017 |
Immigrant origin | ||||||||||
Country per capita income | ||||||||||
High-middle | . . . | . . . | . . . | . . . | . . . | . . . | . . . | . . . | -0.086** | 0.040 |
Low-middle | . . . | . . . | . . . | . . . | . . . | . . . | . . . | . . . | -0.112** | 0.050 |
Low | . . . | . . . | . . . | . . . | . . . | . . . | . . . | . . . | -0.074 | 0.069 |
Mexico | . . . | . . . | . . . | . . . | . . . | . . . | . . . | . . . | -0.101*** | 0.036 |
Constant | 10.342*** | 0.010 | 9.917*** | 0.020 | 9.389*** | 0.017 | 9.378*** | 0.019 | 9.377*** | 0.018 |
R-squared | 0.066 | 0.348 | 0.285 | 0.389 | 0.389 | |||||
Observations | 105,268 | 103,289 | 103,289 | 86,382 | 86,382 | |||||
SOURCE: Authors' calculations based on HRS (1998–2012 waves) and geocoded data from HRS. | ||||||||||
NOTES: . . . = not applicable.
* = statistically significant at the p < 0.10 level; ** = statistically significant at the p < 0.05 level; *** = statistically significant at the p < 0.01 level.
|
Variable | Baseline covariates | With demographic covariates added | With lifecycle covariates added | With transfer-payment covariates added | With immigrant origin and race/ethnicity covariates added | |||||
---|---|---|---|---|---|---|---|---|---|---|
Coefficient | Standard error | Coefficient | Standard error | Coefficient | Standard error | Coefficient | Standard error | Coefficient | Standard error | |
Immigration cohort | ||||||||||
Pre-1955 | -0.183*** | 0.051 | -0.063 | 0.048 | 0.048 | 0.045 | 0.049 | 0.043 | 0.091** | 0.046 |
1955–1964 | -0.129** | 0.055 | 0.036 | 0.053 | 0.097* | 0.051 | 0.056 | 0.047 | 0.107** | 0.050 |
1965–1974 | -0.551*** | 0.044 | -0.125*** | 0.047 | -0.008 | 0.047 | -0.041 | 0.043 | 0.044 | 0.051 |
1975–1984 | -0.776*** | 0.045 | -0.298*** | 0.047 | -0.122** | 0.062 | -0.159*** | 0.045 | -0.070 | 0.054 |
1985 or later | -1.172*** | 0.065 | -0.686*** | 0.066 | -0.280*** | 0.076 | -0.354*** | 0.080 | -0.271*** | 0.083 |
Age | ||||||||||
65–74 | 0.132*** | 0.010 | 0.165*** | 0.008 | 0.126*** | 0.012 | 0.135*** | 0.009 | 0.135*** | 0.009 |
75 or older | 0.255*** | 0.013 | 0.366*** | 0.012 | 0.367*** | 0.027 | 0.364*** | 0.018 | 0.365*** | 0.018 |
Married couple | . . . | . . . | 0.295*** | 0.010 | 0.022* | 0.012 | -0.006 | 0.011 | -0.006 | 0.011 |
Immigrant and immigrant | . . . | . . . | -0.015 | 0.037 | -0.028 | 0.034 | -0.044 | 0.032 | -0.035 | 0.032 |
Immigrant and native | . . . | . . . | -0.020 | 0.028 | 0.026 | 0.029 | 0.004 | 0.025 | -0.006 | 0.025 |
Naturalized citizen | . . . | . . . | 0.133*** | 0.036 | 0.028 | 0.037 | 0.020 | 0.035 | 0.029 | 0.035 |
Non-English speaker | . . . | . . . | -0.246*** | 0.040 | -0.144*** | 0.039 | -0.113*** | 0.038 | -0.089** | 0.039 |
Education | ||||||||||
High school diploma | . . . | . . . | 0.427*** | 0.013 | 0.260*** | 0.015 | 0.180*** | 0.012 | 0.180*** | 0.012 |
College degree | . . . | . . . | 0.936*** | 0.017 | 0.635*** | 0.026 | 0.464*** | 0.018 | 0.464*** | 0.018 |
Race/ethnicity | ||||||||||
Hispanic— | ||||||||||
White | . . . | . . . | -0.515*** | 0.023 | -0.350*** | 0.028 | -0.295*** | 0.021 | -0.265*** | 0.024 |
Nonwhite | . . . | . . . | -0.516*** | 0.032 | -0.295*** | 0.035 | -0.245*** | 0.033 | -0.166*** | 0.035 |
Nonwhite non-Hispanic | . . . | . . . | -0.360*** | 0.013 | -0.170*** | 0.021 | -0.122*** | 0.014 | -0.118*** | 0.014 |
Family size | . . . | . . . | -0.035*** | 0.004 | -0.016*** | 0.004 | -0.015*** | 0.003 | -0.015*** | 0.003 |
Number of children | . . . | . . . | -0.020*** | 0.002 | -0.016*** | 0.003 | -0.011*** | 0.002 | -0.011*** | 0.002 |
Share of wealth from— | ||||||||||
Financial assets | . . . | . . . | . . . | . . . | 0.005** | 0.002 | 0.005*** | 0.001 | 0.005*** | 0.001 |
Nonfinancial assets | . . . | . . . | . . . | . . . | 0.002** | 0.001 | 0.003*** | 0.001 | 0.003*** | 0.001 |
Logarithm of average household earnings | . . . | . . . | . . . | . . . | 0.098*** | 0.005 | 0.074*** | 0.005 | 0.074*** | 0.005 |
Standard deviation of logarithm of average household earnings | . . . | . . . | . . . | . . . | 0.005 | 0.015 | -0.010 | 0.015 | -0.011 | 0.015 |
Homeowner | . . . | . . . | . . . | . . . | 0.480*** | 0.027 | 0.302*** | 0.018 | 0.300*** | 0.018 |
Business owner | . . . | . . . | . . . | . . . | 0.258*** | 0.025 | 0.154*** | 0.020 | 0.155*** | 0.020 |
Health status | ||||||||||
Good | . . . | . . . | . . . | . . . | -0.097*** | 0.011 | -0.056*** | 0.008 | -0.056*** | 0.008 |
Fair or poor | . . . | . . . | . . . | . . . | -0.231*** | 0.016 | -0.147*** | 0.010 | -0.147*** | 0.010 |
Medical out-of-pocket costs | ||||||||||
Middle tercile | . . . | . . . | . . . | . . . | 0.100*** | 0.009 | 0.075*** | 0.007 | 0.074*** | 0.007 |
Highest tercile | . . . | . . . | . . . | . . . | 0.128*** | 0.011 | 0.106*** | 0.009 | 0.106*** | 0.009 |
Expect to leave bequest | ||||||||||
Deemed likely, greater than $10,000 | . . . | . . . | . . . | . . . | . . . | . . . | 0.102*** | 0.008 | 0.102*** | 0.008 |
Deemed likely, greater than $100,000 | . . . | . . . | . . . | . . . | . . . | . . . | 0.351*** | 0.013 | 0.351*** | 0.013 |
Child(ren) within 10 miles | . . . | . . . | . . . | . . . | . . . | . . . | -0.008 | 0.008 | -0.009 | 0.008 |
Transfers | ||||||||||
To child(ren) | . . . | . . . | . . . | . . . | . . . | . . . | 0.169*** | 0.007 | 0.168*** | 0.007 |
From child(ren) | . . . | . . . | . . . | . . . | . . . | . . . | -0.106*** | 0.012 | -0.105*** | 0.012 |
To relative(s) | . . . | . . . | . . . | . . . | . . . | . . . | 0.147*** | 0.012 | 0.146*** | 0.012 |
From relative(s) | . . . | . . . | . . . | . . . | . . . | . . . | -0.161*** | 0.018 | -0.161*** | 0.018 |
Immigrant race/ethnicity | ||||||||||
Hispanic— | ||||||||||
White | . . . | . . . | . . . | . . . | . . . | . . . | . . . | . . . | -0.238*** | 0.068 |
Nonwhite | . . . | . . . | . . . | . . . | . . . | . . . | . . . | . . . | -0.131*** | 0.045 |
Nonwhite non-Hispanic | . . . | . . . | . . . | . . . | . . . | . . . | . . . | . . . | -0.097** | 0.047 |
Constant | 10.292*** | 0.008 | 9.937*** | 0.017 | 8.347*** | 0.078 | 8.578*** | 0.070 | 8.578*** | 0.070 |
R-squared | 0.067 | 0.350 | 0.521 | 0.600 | 0.600 | |||||
Observations | 105,268 | 103,289 | 81,138 | 69,055 | 69,055 | |||||
SOURCE: Authors' calculations based on HRS (1998–2012 waves) and SSA earnings records. | ||||||||||
NOTES: . . . = not applicable.
* = statistically significant at the p < 0.10 level; ** = statistically significant at the p < 0.05 level; *** = statistically significant at the p < 0.01 level.
|
Variable | Baseline covariates | With demographic covariates added | With lifecycle covariates added | With transfer-payment covariates added | With immigrant origin and race/ethnicity covariates added | |||||
---|---|---|---|---|---|---|---|---|---|---|
Coefficient | Standard error | Coefficient | Standard error | Coefficient | Standard error | Coefficient | Standard error | Coefficient | Standard error | |
Immigration cohort | ||||||||||
Pre-1955 | -0.183*** | 0.051 | -0.063 | 0.048 | -0.016 | 0.040 | -0.006 | 0.041 | 0.047 | 0.042 |
1955–1964 | -0.120** | 0.055 | 0.036 | 0.053 | 0.037 | 0.044 | 0.022 | 0.043 | 0.096** | 0.046 |
1965–1974 | -0.551*** | 0.044 | -0.125*** | 0.047 | -0.095** | 0.040 | -0.116*** | 0.040 | -0.010 | 0.047 |
1975–1984 | -0.776*** | 0.045 | -0.298*** | 0.047 | -0.242*** | 0.048 | -0.250*** | 0.040 | -0.126*** | 0.048 |
1985 or later | -1.172*** | 0.065 | -0.686*** | 0.066 | -0.565*** | 0.060 | -0.596*** | 0.066 | -0.483*** | 0.069 |
Age | ||||||||||
70–79 | 0.132*** | 0.010 | 0.165*** | 0.008 | 0.132*** | 0.009 | 0.151*** | 0.009 | 0.151*** | 0.009 |
80 or older | 0.255*** | 0.013 | 0.366*** | 0.012 | 0.361*** | 0.019 | 0.391*** | 0.018 | 0.392*** | 0.018 |
Married couple | . . . | . . . | 0.295*** | 0.010 | 0.109*** | 0.010 | 0.051*** | 0.009 | 0.050*** | 0.009 |
Immigrant and immigrant | . . . | . . . | -0.015 | 0.037 | -0.025 | 0.032 | -0.027 | 0.032 | -0.016 | 0.031 |
Immigrant and native | . . . | . . . | -0.020 | 0.028 | -0.008 | 0.026 | -0.008 | 0.023 | -0.015 | 0.023 |
Naturalized citizen | . . . | . . . | 0.133*** | 0.036 | 0.090*** | 0.032 | 0.071** | 0.032 | 0.072** | 0.032 |
Non-English speaker | . . . | . . . | -0.246*** | 0.040 | -0.230*** | 0.034 | -0.175*** | 0.034 | -0.164*** | 0.034 |
Education | ||||||||||
High school diploma | . . . | . . . | 0.427*** | 0.013 | 0.285*** | 0.012 | 0.195*** | 0.010 | 0.194*** | 0.010 |
College degree | . . . | . . . | 0.936*** | 0.017 | 0.665*** | 0.020 | 0.487*** | 0.016 | 0.487*** | 0.016 |
Race/ethnicity | ||||||||||
Hispanic— | ||||||||||
White | . . . | . . . | -0.515*** | 0.023 | -0.405*** | 0.021 | -0.351*** | 0.020 | -0.320*** | 0.020 |
Nonwhite | . . . | . . . | -0.516*** | 0.032 | -0.370*** | 0.029 | -0.331*** | 0.028 | -0.315*** | 0.028 |
Nonwhite non-Hispanic | . . . | . . . | -0.360*** | 0.013 | -0.248*** | 0.017 | -0.189*** | 0.015 | -0.183*** | 0.015 |
Family size | . . . | . . . | -0.035*** | 0.004 | -0.025*** | 0.003 | -0.022*** | 0.003 | -0.021*** | 0.003 |
Number of children | . . . | . . . | -0.020*** | 0.002 | -0.015*** | 0.002 | -0.013*** | 0.002 | -0.012*** | 0.002 |
Share of wealth from— | ||||||||||
Financial assets | . . . | . . . | . . . | . . . | 0.003** | 0.001 | 0.002* | 0.001 | 0.002* | 0.001 |
Nonfinancial assets | . . . | . . . | . . . | . . . | 0.002** | 0.001 | 0.002*** | 0.001 | 0.002*** | 0.001 |
Urban residence | . . . | . . . | . . . | . . . | 0.195*** | 0.009 | 0.142*** | 0.008 | 0.141*** | 0.008 |
Homeowner | . . . | . . . | . . . | . . . | 0.556*** | 0.019 | 0.369*** | 0.015 | 0.370*** | 0.015 |
Business owner | . . . | . . . | . . . | . . . | 0.302*** | 0.020 | 0.194*** | 0.016 | 0.195*** | 0.016 |
Health status | ||||||||||
Good | . . . | . . . | . . . | . . . | -0.106*** | 0.008 | -0.064*** | 0.007 | -0.064*** | 0.007 |
Fair or poor | . . . | . . . | . . . | . . . | -0.248*** | 0.011 | -0.158*** | 0.009 | -0.158*** | 0.009 |
Medical out-of-pocket costs | ||||||||||
Middle tercile | . . . | . . . | . . . | . . . | 0.128*** | 0.007 | 0.093*** | 0.007 | 0.093*** | 0.007 |
Highest tercile | . . . | . . . | . . . | . . . | 0.166*** | 0.009 | 0.134*** | 0.009 | 0.134*** | 0.009 |
Expect to leave bequest | ||||||||||
Deemed likely, greater than $10,000 | . . . | . . . | . . . | . . . | . . . | . . . | 0.115*** | 0.008 | 0.115*** | 0.008 |
Deemed likely, greater than $100,000 | . . . | . . . | . . . | . . . | . . . | . . . | 0.372*** | 0.013 | 0.372*** | 0.013 |
Child(ren) within 10 miles | . . . | . . . | . . . | . . . | . . . | . . . | -0.014* | 0.007 | -0.014** | 0.007 |
Transfers | ||||||||||
To child(ren) | . . . | . . . | . . . | . . . | . . . | . . . | 0.185*** | 0.007 | 0.185*** | 0.007 |
From child(ren) | . . . | . . . | . . . | . . . | . . . | . . . | -0.125*** | 0.011 | -0.124*** | 0.011 |
To relative(s) | . . . | . . . | . . . | . . . | . . . | . . . | 0.153*** | 0.011 | 0.153*** | 0.011 |
From relative(s) | . . . | . . . | . . . | . . . | . . . | . . . | -0.173*** | 0.017 | -0.172*** | 0.017 |
Immigrant origin | ||||||||||
Country per capita income | ||||||||||
High-middle | . . . | . . . | . . . | . . . | . . . | . . . | . . . | . . . | -0.121*** | 0.042 |
Low-middle | . . . | . . . | . . . | . . . | . . . | . . . | . . . | . . . | -0.166*** | 0.050 |
Low | . . . | . . . | . . . | . . . | . . . | . . . | . . . | . . . | -0.171*** | 0.054 |
Mexico | . . . | . . . | . . . | . . . | . . . | . . . | . . . | . . . | -0.096** | 0.045 |
Constant | 10.292*** | 0.008 | 9.937*** | 0.017 | 9.539*** | 0.021 | 9.480*** | 0.020 | 9.475*** | 0.020 |
R-squared | 0.067 | 0.350 | 0.494 | 0.571 | 0.572 | |||||
Observations | 105,268 | 103,289 | 103,289 | 86,382 | 86,382 | |||||
SOURCE: Authors' calculations based on HRS (1998–2012 waves) and geocoded data from HRS. | ||||||||||
NOTES: . . . = not applicable.
* = statistically significant at the p < 0.10 level; ** = statistically significant at the p < 0.05 level; *** = statistically significant at the p < 0.01 level.
|
Variable | Baseline covariates | With demographic covariates added | With lifecycle covariates added | With transfer-payment covariates added | With immigrant origin and race/ethnicity covariates added | |||||
---|---|---|---|---|---|---|---|---|---|---|
Coefficient | Standard error | Coefficient | Standard error | Coefficient | Standard error | Coefficient | Standard error | Coefficient | Standard error | |
Immigration cohort | ||||||||||
1955–1964 | 0.200* | 0.111 | 0.052 | 0.091 | -0.012 | 0.058 | -0.019 | 0.053 | -0.029 | 0.048 |
1965–1974 | -0.202** | 0.096 | -0.222*** | 0.070 | -0.109** | 0.046 | -0.101** | 0.048 | -0.080* | 0.041 |
1975–1984 | -0.534*** | 0.088 | -0.399*** | 0.070 | -0.225*** | 0.050 | -0.208*** | 0.050 | -0.187*** | 0.045 |
1985 or later | -0.819*** | 0.090 | -0.767*** | 0.087 | -0.209*** | 0.071 | -0.233*** | 0.069 | -0.242*** | 0.078 |
Age | ||||||||||
65–74 | . . . | . . . | 0.068** | 0.032 | 0.051 | 0.025 | 0.089*** | 0.025 | 0.072*** | 0.023 |
75 or older | . . . | . . . | 0.157*** | 0.051 | 0.155*** | 0.038 | 0.225*** | 0.039 | 0.194*** | 0.037 |
Married | 0.257*** | 0.042 | -0.072** | 0.034 | -0.094*** | 0.033 | -0.058* | 0.033 | ||
Naturalized citizen | . . . | . . . | 0.111** | 0.047 | -0.003 | 0.032 | -0.009 | 0.031 | 0.022 | 0.032 |
Education | ||||||||||
High school diploma | . . . | . . . | 0.560*** | 0.050 | 0.219*** | 0.038 | 0.183*** | 0.036 | 0.148*** | 0.036 |
College degree | . . . | . . . | 1.177*** | 0.060 | 0.493*** | 0.059 | 0.385*** | 0.058 | 0.320*** | 0.061 |
Family size | . . . | . . . | -0.032*** | 0.011 | -0.011 | 0.007 | -0.002 | 0.007 | 0.003 | 0.007 |
Number of children | . . . | . . . | -0.026*** | 0.008 | -0.006 | 0.006 | -0.005 | 0.007 | -0.005 | 0.007 |
Share of wealth from— | ||||||||||
Financial assets | . . . | . . . | . . . | . . . | 0.023*** | 0.002 | 0.019*** | 0.002 | 0.015*** | 0.002 |
Nonfinancial assets | . . . | . . . | . . . | . . . | 0.008*** | 0.001 | 0.007*** | 0.001 | 0.006*** | 0.001 |
Logarithm of average household earnings | . . . | . . . | . . . | . . . | 0.162*** | 0.021 | 0.127*** | 0.020 | 0.129*** | 0.023 |
Standard deviation of logarithm of average household earnings | . . . | . . . | . . . | . . . | 0.036 | 0.047 | -0.007 | 0.050 | -0.008 | 0.054 |
Homeowner | . . . | . . . | . . . | . . . | 0.272*** | 0.041 | 0.196*** | 0.039 | 0.196*** | 0.033 |
Business owner | . . . | . . . | . . . | . . . | 0.195** | 0.092 | 0.054 | 0.086 | 0.045 | 0.099 |
Health status | ||||||||||
Good | . . . | . . . | . . . | . . . | -0.068* | 0.036 | -0.080** | 0.040 | -0.046 | 0.032 |
Fair or poor | . . . | . . . | . . . | . . . | -0.213*** | 0.041 | -0.194*** | 0.043 | -0.152*** | 0.037 |
Medical out-of-pocket costs | ||||||||||
Middle tercile | . . . | . . . | . . . | . . . | 0.213*** | 0.059 | 0.130*** | 0.045 | 0.080 | 0.054 |
Highest tercile | . . . | . . . | . . . | . . . | 0.132** | 0.052 | 0.090* | 0.049 | 0.059 | 0.045 |
Expect to leave bequest | ||||||||||
Deemed likely, greater than $10,000 | . . . | . . . | . . . | . . . | . . . | . . . | 0.063** | 0.027 | 0.057** | 0.026 |
Deemed likely, greater than $100,000 | . . . | . . . | . . . | . . . | . . . | . . . | 0.239*** | 0.041 | 0.250*** | 0.036 |
Child(ren) within 10 miles | . . . | . . . | . . . | . . . | . . . | . . . | 0.000 | 0.024 | 0.000 | 0.023 |
Transfers | ||||||||||
To child(ren) | . . . | . . . | . . . | . . . | . . . | . . . | 0.220*** | 0.029 | 0.186*** | 0.027 |
From child(ren) | . . . | . . . | . . . | . . . | . . . | . . . | -0.017 | 0.035 | -0.016 | 0.030 |
To relative(s) | . . . | . . . | . . . | . . . | . . . | . . . | 0.085** | 0.034 | 0.108*** | 0.036 |
From relative(s) | . . . | . . . | . . . | . . . | . . . | . . . | -0.110 | 0.088 | -0.027 | 0.085 |
Immigrant race/ethnicity | ||||||||||
Hispanic— | ||||||||||
White | . . . | . . . | . . . | . . . | . . . | . . . | . . . | . . . | -0.327*** | 0.052 |
Nonwhite | . . . | . . . | . . . | . . . | . . . | . . . | . . . | . . . | -0.337*** | 0.060 |
Nonwhite non-Hispanic | . . . | . . . | . . . | . . . | . . . | . . . | . . . | . . . | -0.200*** | 0.058 |
Constant | 9.909*** | 0.068 | 9.544*** | 0.074 | 7.302*** | 0.285 | 7.737*** | 0.270 | 7.937*** | 0.309 |
R-squared | 0.105 | 0.323 | 0.489 | 0.560 | 0.606 | |||||
Observations | 9,135 | 8,985 | 6,206 | 5,087 | 5,087 | |||||
SOURCE: Authors' calculations based on HRS (1998–2012 waves) and SSA earnings records. | ||||||||||
NOTES: . . . = not applicable.
* = statistically significant at the p < 0.10 level; ** = statistically significant at the p < 0.05 level; *** = statistically significant at the p < 0.01 level.
|
Variable | Baseline covariates | With demographic covariates added | With lifecycle covariates added | With transfer-payment covariates added | With immigrant origin and race/ethnicity covariates added | |||||
---|---|---|---|---|---|---|---|---|---|---|
Coefficient | Standard error | Coefficient | Standard error | Coefficient | Standard error | Coefficient | Standard error | Coefficient | Standard error | |
Immigration cohort | ||||||||||
1955–1964 | 0.200* | 0.111 | 0.052 | 0.091 | 0.070 | 0.052 | 0.022 | 0.043 | 0.054 | 0.046 |
1965–1974 | -0.202** | 0.096 | -0.222*** | 0.070 | -0.129*** | 0.042 | -0.138*** | 0.043 | -0.069 | 0.050 |
1975–1984 | -0.534*** | 0.088 | -0.399*** | 0.070 | -0.250*** | 0.042 | -0.256*** | 0.043 | -0.196*** | 0.054 |
1985 or later | -0.819*** | 0.090 | -0.767*** | 0.087 | -0.440*** | 0.062 | -0.496*** | 0.068 | -0.447*** | 0.082 |
Age | ||||||||||
70–79 | . . . | . . . | 0.068** | 0.032 | 0.080*** | 0.027 | 0.093*** | 0.023 | 0.056** | 0.025 |
80 or older | . . . | . . . | 0.157*** | 0.051 | 0.157*** | 0.032 | 0.195*** | 0.033 | 0.152*** | 0.035 |
Married | 0.257*** | 0.042 | 0.026 | 0.031 | 0.003 | 0.029 | 0.017 | 0.030 | ||
Naturalized citizen | . . . | . . . | 0.560*** | 0.050 | 0.286*** | 0.038 | 0.225*** | 0.035 | 0.147*** | 0.038 |
Education | ||||||||||
High school diploma | . . . | . . . | 1.177*** | 0.060 | 0.556*** | 0.057 | 0.391*** | 0.053 | 0.327*** | 0.052 |
College degree | . . . | . . . | 0.111** | 0.047 | 0.019 | 0.029 | 0.002 | 0.028 | 0.009 | 0.031 |
Family size | . . . | . . . | -0.032*** | 0.011 | -0.027*** | 0.007 | -0.018*** | 0.006 | -0.010 | 0.007 |
Number of children | . . . | . . . | -0.026*** | 0.008 | -0.002 | 0.005 | -0.003 | 0.005 | 0.004 | 0.006 |
Share of wealth from— | ||||||||||
Financial assets | . . . | . . . | . . . | . . . | 0.022*** | 0.002 | 0.020*** | 0.002 | 0.017*** | 0.002 |
Nonfinancial assets | . . . | . . . | . . . | . . . | 0.006*** | 0.001 | 0.005*** | 0.001 | 0.005*** | 0.001 |
Urban residence | . . . | . . . | . . . | . . . | 0.245*** | 0.032 | 0.214*** | 0.028 | 0.173*** | 0.033 |
Homeowner | . . . | . . . | . . . | . . . | 0.442*** | 0.045 | 0.351*** | 0.034 | 0.364*** | 0.034 |
Business owner | . . . | . . . | . . . | . . . | 0.207** | 0.086 | 0.077 | 0.071 | 0.033 | 0.076 |
Health status | ||||||||||
Good | . . . | . . . | . . . | . . . | -0.125*** | 0.033 | -0.111*** | 0.031 | -0.092*** | 0.032 |
Fair or poor | . . . | . . . | . . . | . . . | -0.283*** | 0.036 | -0.230*** | 0.035 | -0.183*** | 0.035 |
Medical out-of-pocket costs | ||||||||||
Middle tercile | . . . | . . . | . . . | . . . | 0.142*** | 0.023 | 0.095*** | 0.022 | 0.079*** | 0.022 |
Highest tercile | . . . | . . . | . . . | . . . | 0.149*** | 0.028 | 0.113*** | 0.026 | 0.090*** | 0.029 |
Expect to leave bequest | ||||||||||
Deemed likely, greater than $10,000 | . . . | . . . | . . . | . . . | . . . | . . . | 0.034 | 0.027 | 0.048** | 0.024 |
Deemed likely, greater than $100,000 | . . . | . . . | . . . | . . . | . . . | . . . | 0.277*** | 0.034 | 0.265*** | 0.034 |
Child(ren) within 10 miles | . . . | . . . | . . . | . . . | . . . | . . . | -0.006 | 0.021 | -0.014 | 0.022 |
Transfers | ||||||||||
To child(ren) | . . . | . . . | . . . | . . . | . . . | . . . | 0.244*** | 0.025 | 0.218*** | 0.024 |
From child(ren) | . . . | . . . | . . . | . . . | . . . | . . . | -0.051* | 0.031 | -0.051 | 0.033 |
To relative(s) | . . . | . . . | . . . | . . . | . . . | . . . | 0.117*** | 0.034 | 0.154*** | 0.036 |
From relative(s) | . . . | . . . | . . . | . . . | . . . | . . . | -0.155** | 0.079 | -0.159* | 0.094 |
Immigrant race/ethnicity | ||||||||||
Hispanic— | ||||||||||
White | . . . | . . . | . . . | . . . | . . . | . . . | . . . | . . . | -0.257*** | 0.051 |
Nonwhite | . . . | . . . | . . . | . . . | . . . | . . . | . . . | . . . | -0.284*** | 0.060 |
Nonwhite non-Hispanic | . . . | . . . | . . . | . . . | . . . | . . . | . . . | . . . | -0.069 | 0.058 |
Immigrant origin | ||||||||||
Country per capita income | ||||||||||
High-middle | . . . | . . . | . . . | . . . | . . . | . . . | . . . | . . . | -0.072* | 0.043 |
Low-middle | . . . | . . . | . . . | . . . | . . . | . . . | . . . | . . . | -0.138** | 0.054 |
Low | . . . | . . . | . . . | . . . | . . . | . . . | . . . | . . . | -0.084 | 0.056 |
Mexico | . . . | . . . | . . . | . . . | . . . | . . . | . . . | . . . | -0.067 | 0.046 |
Constant | 9.909*** | 0.068 | 9.544*** | 0.074 | 9.166*** | 0.053 | 9.176*** | 0.054 | 9.396*** | 0.071 |
R-squared | 0.105 | 0.323 | 0.430 | 0.513 | 0.550 | |||||
Observations | 9,135 | 8,985 | 8,985 | 7,175 | 7,175 | |||||
SOURCE: Authors' calculations based on HRS (1998–2012 waves) and geocoded data from HRS. | ||||||||||
NOTES: . . . = not applicable.
* = statistically significant at the p < 0.10 level; ** = statistically significant at the p < 0.05 level; *** = statistically significant at the p < 0.01 level.
|
Variable | Baseline covariates | With demographic covariates added | With lifecycle covariates added | With transfer-payment covariates added | With immigrant origin and race/ethnicity covariates added | |||||
---|---|---|---|---|---|---|---|---|---|---|
Coefficient | Standard error | Coefficient | Standard error | Coefficient | Standard error | Coefficient | Standard error | Coefficient | Standard error | |
Immigration cohort | ||||||||||
1955–1964 | 0.166** | 0.080 | 0.116 | 0.073 | 0.027 | 0.058 | 0.001 | 0.056 | 0.002 | 0.054 |
1965–1974 | -0.257*** | 0.068 | -0.216*** | 0.060 | -0.138*** | 0.052 | -0.140*** | 0.051 | -0.073 | 0.049 |
1975–1984 | -0.493*** | 0.068 | -0.413*** | 0.063 | -0.273*** | 0.059 | -0.242*** | 0.057 | -0.182*** | 0.054 |
1985 or later | -0.886*** | 0.086 | -0.799*** | 0.085 | -0.312*** | 0.092 | -0.369*** | 0.090 | -0.340*** | 0.089 |
Age | ||||||||||
65–74 | . . . | . . . | 0.141*** | 0.032 | 0.146*** | 0.033 | 0.171*** | 0.033 | 0.123*** | 0.031 |
75 or older | . . . | . . . | 0.315*** | 0.049 | 0.369*** | 0.053 | 0.415*** | 0.053 | 0.324*** | 0.047 |
Married | 0.288*** | 0.041 | -0.046 | 0.038 | -0.090** | 0.038 | -0.074** | 0.037 | ||
Naturalized citizen | . . . | . . . | 0.156*** | 0.043 | 0.040 | 0.039 | 0.022 | 0.037 | 0.040 | 0.036 |
Education | ||||||||||
High school diploma | . . . | . . . | 0.513*** | 0.047 | 0.289*** | 0.041 | 0.218*** | 0.038 | 0.146*** | 0.038 |
College degree | . . . | . . . | 1.105*** | 0.065 | 0.593*** | 0.075 | 0.432*** | 0.070 | 0.331*** | 0.069 |
Family size | . . . | . . . | -0.056*** | 0.010 | -0.022** | 0.009 | -0.007 | 0.009 | 0.000 | 0.009 |
Number of children | . . . | . . . | -0.032*** | 0.009 | -0.015** | 0.007 | -0.017** | 0.008 | -0.013* | 0.007 |
Share of wealth from— | ||||||||||
Financial assets | . . . | . . . | . . . | . . . | 0.009** | 0.004 | 0.007* | 0.004 | 0.006* | 0.003 |
Nonfinancial assets | . . . | . . . | . . . | . . . | 0.004*** | 0.001 | 0.003** | 0.002 | 0.003* | 0.001 |
Logarithm of average household earnings | . . . | . . . | . . . | . . . | 0.179 | 0.019 | 0.141*** | 0.019 | 0.134*** | 0.018 |
Standard deviation of logarithm of average household earnings | . . . | . . . | . . . | . . . | 0.007 | 0.052 | -0.006 | 0.049 | -0.006 | 0.048 |
Homeowner | . . . | . . . | . . . | . . . | 0.438*** | 0.051 | 0.306*** | 0.048 | 0.294*** | 0.045 |
Business owner | . . . | . . . | . . . | . . . | 0.315*** | 0.095 | 0.127 | 0.095 | 0.091 | 0.088 |
Health status | ||||||||||
Good | . . . | . . . | . . . | . . . | -0.104*** | 0.038 | -0.080** | 0.039 | -0.053 | 0.038 |
Fair or poor | . . . | . . . | . . . | . . . | -0.281*** | 0.042 | -0.217*** | 0.041 | -0.161*** | 0.041 |
Medical out-of-pocket costs | ||||||||||
Middle tercile | . . . | . . . | . . . | . . . | 0.159*** | 0.058 | 0.110* | 0.061 | 0.099* | 0.058 |
Highest tercile | . . . | . . . | . . . | . . . | 0.207*** | 0.058 | 0.146** | 0.060 | 0.130** | 0.056 |
Expect to leave bequest | ||||||||||
Deemed likely, greater than $10,000 | . . . | . . . | . . . | . . . | . . . | . . . | 0.082*** | 0.031 | 0.089*** | 0.030 |
Deemed likely, greater than $100,000 | . . . | . . . | . . . | . . . | . . . | . . . | 0.345*** | 0.044 | 0.309*** | 0.039 |
Child(ren) within 10 miles | . . . | . . . | . . . | . . . | . . . | . . . | 0.005 | 0.026 | 0.015 | 0.026 |
Transfers | ||||||||||
To child(ren) | . . . | . . . | . . . | . . . | . . . | . . . | 0.273*** | 0.031 | 0.227*** | 0.028 |
From child(ren) | . . . | . . . | . . . | . . . | . . . | . . . | -0.080** | 0.040 | -0.071* | 0.039 |
To relative(s) | . . . | . . . | . . . | . . . | . . . | . . . | 0.155*** | 0.043 | 0.161*** | 0.043 |
From relative(s) | . . . | . . . | . . . | . . . | . . . | . . . | -0.218 | 0.133 | -0.243* | 0.126 |
Immigrant race/ethnicity | ||||||||||
Hispanic— | ||||||||||
White | . . . | . . . | . . . | . . . | . . . | . . . | . . . | . . . | -0.453*** | 0.059 |
Nonwhite | . . . | . . . | . . . | . . . | . . . | . . . | . . . | . . . | -0.473*** | 0.072 |
Nonwhite non-Hispanic | . . . | . . . | . . . | . . . | . . . | . . . | . . . | . . . | -0.277*** | 0.066 |
Constant | 10.048*** | 0.049 | 9.623*** | 0.067 | 7.168*** | 0.259 | 7.593*** | 0.253 | 7.970*** | 0.247 |
R-squared | 0.108 | 0.334 | 0.568 | 0.626 | 0.653 | |||||
Observations | 9,135 | 8,985 | 6,206 | 5,087 | 5,087 | |||||
SOURCE: Authors' calculations based on HRS (1998–2012 waves) and SSA earnings records. | ||||||||||
NOTES: . . . = not applicable.
* = statistically significant at the p < 0.10 level; ** = statistically significant at the p < 0.05 level; *** = statistically significant at the p < 0.01 level.
|
Variable | Baseline covariates | With demographic covariates added | With lifecycle covariates added | With transfer-payment covariates added | With immigrant origin and race/ethnicity covariates added | |||||
---|---|---|---|---|---|---|---|---|---|---|
Coefficient | Standard error | Coefficient | Standard error | Coefficient | Standard error | Coefficient | Standard error | Coefficient | Standard error | |
Immigration cohort | ||||||||||
1955–1964 | 0.166** | 0.080 | 0.116 | 0.073 | 0.082 | 0.052 | 0.057 | 0.051 | 0.088* | 0.050 |
1965–1974 | -0.257*** | 0.068 | -0.216*** | 0.060 | -0.145*** | 0.046 | -0.161*** | 0.046 | -0.066 | 0.047 |
1975–1984 | -0.493*** | 0.068 | -0.413*** | 0.063 | -0.308*** | 0.051 | -0.286*** | 0.051 | -0.189*** | 0.053 |
1985 or later | -0.886*** | 0.086 | -0.799*** | 0.085 | -0.588*** | 0.074 | -0.623*** | 0.077 | -0.566*** | 0.076 |
Age | ||||||||||
70–79 | . . . | . . . | 0.141*** | 0.032 | 0.170*** | 0.029 | 0.206*** | 0.030 | 0.142*** | 0.029 |
80 or older | . . . | . . . | 0.315*** | 0.049 | 0.387*** | 0.043 | 0.433*** | 0.046 | 0.332*** | 0.042 |
Married | 0.288*** | 0.041 | 0.068* | 0.035 | 0.018 | 0.036 | 0.029 | 0.035 | ||
Naturalized citizen | . . . | . . . | 0.156*** | 0.043 | 0.112*** | 0.034 | 0.081** | 0.034 | 0.078** | 0.033 |
Education | ||||||||||
High school diploma | . . . | . . . | 0.513*** | 0.047 | 0.331*** | 0.038 | 0.254*** | 0.037 | 0.154*** | 0.039 |
College degree | . . . | . . . | 1.105*** | 0.065 | 0.657*** | 0.060 | 0.464*** | 0.060 | 0.347*** | 0.062 |
Family size | . . . | . . . | -0.056*** | 0.010 | -0.043*** | 0.008 | -0.031*** | 0.009 | -0.020** | 0.008 |
Number of children | . . . | . . . | -0.032*** | 0.009 | -0.014** | 0.007 | -0.014* | 0.007 | -0.004 | 0.007 |
Share of wealth from— | ||||||||||
Financial assets | . . . | . . . | . . . | . . . | 0.008*** | 0.002 | 0.007** | 0.003 | 0.006** | 0.003 |
Nonfinancial assets | . . . | . . . | . . . | . . . | 0.001 | 0.001 | 0.001 | 0.001 | 0.000 | 0.001 |
Urban residence | . . . | . . . | . . . | . . . | 0.260*** | 0.035 | 0.207*** | 0.034 | 0.200*** | 0.036 |
Homeowner | . . . | . . . | . . . | . . . | 0.656*** | 0.049 | 0.484*** | 0.052 | 0.490*** | 0.050 |
Business owner | . . . | . . . | . . . | . . . | 0.348*** | 0.082 | 0.148* | 0.084 | 0.134* | 0.078 |
Health status | ||||||||||
Good | . . . | . . . | . . . | . . . | -0.161*** | 0.034 | -0.134*** | 0.036 | -0.092*** | 0.035 |
Fair or poor | . . . | . . . | . . . | . . . | -0.379*** | 0.038 | -0.283*** | 0.039 | -0.206*** | 0.037 |
Medical out-of-pocket costs | ||||||||||
Middle tercile | . . . | . . . | . . . | . . . | 0.199*** | 0.025 | 0.126*** | 0.026 | 0.098*** | 0.025 |
Highest tercile | . . . | . . . | . . . | . . . | 0.217*** | 0.032 | 0.152*** | 0.033 | 0.118*** | 0.030 |
Expect to leave bequest | ||||||||||
Deemed likely, greater than $10,000 | . . . | . . . | . . . | . . . | . . . | . . . | 0.065** | 0.030 | 0.072** | 0.029 |
Deemed likely, greater than $100,000 | . . . | . . . | . . . | . . . | . . . | . . . | 0.393*** | 0.038 | 0.368*** | 0.035 |
Child(ren) within 10 miles | . . . | . . . | . . . | . . . | . . . | . . . | -0.017 | 0.026 | -0.011 | 0.025 |
Transfers | ||||||||||
To child(ren) | . . . | . . . | . . . | . . . | . . . | . . . | 0.282*** | 0.027 | 0.233*** | 0.025 |
From child(ren) | . . . | . . . | . . . | . . . | . . . | . . . | -0.119*** | 0.037 | -0.110*** | 0.036 |
To relative(s) | . . . | . . . | . . . | . . . | . . . | . . . | 0.198*** | 0.043 | 0.213*** | 0.042 |
From relative(s) | . . . | . . . | . . . | . . . | . . . | . . . | -0.216** | 0.104 | -0.225** | 0.099 |
Immigrant race/ethnicity | ||||||||||
Hispanic— | ||||||||||
White | . . . | . . . | . . . | . . . | . . . | . . . | . . . | . . . | -0.381*** | 0.057 |
Nonwhite | . . . | . . . | . . . | . . . | . . . | . . . | . . . | . . . | -0.439*** | 0.065 |
Nonwhite non-Hispanic | . . . | . . . | . . . | . . . | . . . | . . . | . . . | . . . | -0.116* | 0.066 |
Immigrant origin | ||||||||||
Country per capita income | ||||||||||
High-middle | . . . | . . . | . . . | . . . | . . . | . . . | . . . | . . . | -0.107** | 0.049 |
Low-middle | . . . | . . . | . . . | . . . | . . . | . . . | . . . | . . . | -0.182*** | 0.063 |
Low | . . . | . . . | . . . | . . . | . . . | . . . | . . . | . . . | -0.190*** | 0.062 |
Mexico | . . . | . . . | . . . | . . . | . . . | . . . | . . . | . . . | -0.081 | 0.053 |
Constant | 10.048*** | 0.049 | 9.623*** | 0.067 | 9.201*** | 0.068 | 9.172*** | 0.076 | 9.468*** | 0.084 |
R-squared | 0.108 | 0.334 | 0.514 | 0.577 | 0.606 | |||||
Observations | 9,135 | 8,985 | 8,985 | 7,175 | 7,175 | |||||
SOURCE: Authors' calculations based on HRS (1998–2012 waves) and geocoded data from HRS. | ||||||||||
NOTES: . . . = not applicable.
* = statistically significant at the p < 0.10 level; ** = statistically significant at the p < 0.05 level; *** = statistically significant at the p < 0.01 level.
|
Notes
1 Previous policy prioritized Western European immigrants and largely excluded immigrants from Asia, Africa, and Latin America.
2 Borjas (1999), Blau and others (2003), and Duleep and Dowhan (2008) include literature reviews of these studies.
3 For brevity, we refer to individuals born in the United States, regardless of race or ethnicity, as “natives.”
4 We discuss literature on immigrant resources more fully in the “Background” section.
5 Melly (2005) presents the decomposition methodology.
6 For a review of literature exploring the effects of immigration on social benefits, see Kerr and Kerr (2013).
7 The HRS is sponsored by the National Institute on Aging (grant number NIA U01AG009740) and is conducted by the University of Michigan. We use the RAND HRS Data File, version N, as well as the wave-specific RAND “fat files.” The RAND version of the HRS consists of an easy-to-use longitudinal file (the main file) and wave-specific enhanced fat files that can be merged at the respondent level. The RAND HRS was developed with funding from the National Institute on Aging and the Social Security Administration.
8 Respondents who report ownership of an asset are asked its value. Respondents who answer that they don't know are asked a series of questions to try to pinpoint a range for the value. For example, “Is it less than $25,000, more than $25,000, or about $25,000?” If the answer is more, a similar question with a higher range of values follows, and so forth.
9 We cannot distinguish between documented and undocumented immigrants in our data. The Immigration Reform and Control Act of 1986 offered amnesty to most undocumented immigrants who had entered the country before 1982. Nearly 3 million immigrants received amnesty as a result. The majority of the immigrants in our sample entered the country before 1982 and those who were undocumented were therefore eligible for the amnesty.
10 For immigrants, we cannot differentiate the effects of arrival cohort and of age at arrival because age at arrival is just a function of age, survey year, and year of arrival.
11 The wealth questions in the HRS are meant to capture total wealth, including foreign assets. However, if foreign assets are underreported, we might underestimate the retirement resources of immigrants.
12 The linear approximations capture the change in the ratios very closely and ease the implementation of the numerical calculations in this article.
13 The HRS reports whether a respondent is white, black, or “other.” For “other,” we apply the relevant adjustment for “white.” Brown, Liebman, and Pollet did not have enough data to estimate mortality rates for black college graduates. We estimate an adjustment factor by assuming that the mortality of black college graduates has the same proportion to black high school graduates as that of white college graduates to white high school graduates. The data in Brown, Liebman, and Pollet (2001) also do not support separate mortality rates for Hispanics by education. For Hispanic respondents, we therefore account for differential mortality only by sex.
14 Our measure does not allow for differences in survival probabilities by immigration status or country of origin. Sevak and Schmidt (2008) found that immigrants experience lower age-specific mortality rates, which is consistent with findings in the public health and demography literatures (see Singh and Siahpush 2001; Dupre, Gu, and Vaupel 2012; Lariscy, Hummer, and Hayward 2015; and Mehta and others 2016).
15 Some immigrants may be covered by a bilateral totalization agreement between the United States and a partner country that allows Social Security eligibility and benefit amounts to be based on earnings accrued in both countries (Barrick and Kestenbaum 2013). The expected Social Security benefits that those immigrants report may not account for that coverage. Because most U.S. totalization agreements are with industrialized countries in Europe and Asia, any discrepancies resulting from the omission of totalized benefits would lead us to underestimate retirement resources for immigrants more at the upper end of the wealth distribution than at the lower end (Sevak and Schmidt 2014).
16 Our measure does not account for the possibility that married couples might divorce during the retirement period.
17 Gustman, Steinmeier, and Tabatabai (2010) discussed substantial reporting error in the HRS pension wealth measures because of confusion among some respondents about pension plan type, despite detailed follow-up questions asked of respondents who provide inconsistent answers to initial queries about plan type and features. To the extent that reporting errors and overall levels of plan information vary randomly across respondents, the self-reported measures primarily increase the noisiness of our comprehensive wealth estimates. However, if information about plan type and plan characteristics depends systemically on demographics, resources, or (most importantly) immigration status, our measure of total household resources may be subject to an important additional source of bias.
18 We define households as married if the respondent reports being married in the first survey wave in which he or she enters our sample, regardless of subsequent changes. The sample therefore includes and defines as “married” some individuals who were divorced or widowed in later waves. We define a “married immigrant household” as one in which both the respondent and the spouse were born outside the United States. Using this definition results in the largest measured immigrant-native wealth gaps because couples comprising one immigrant and one native tend to have higher wealth than do couples comprising two immigrants. We consider a more flexible definition of married immigrant households, and single households, in the regression analysis that follows.
19 Results for single men and single women are available from authors on request (david.love@williams.edu; lschmidt@williams.edu).
20 Another measure could be based on replacement rates (annuitized value of converted assets as a percentage of preretirement income). Given lower preretirement income for immigrants, that measure would likely generate much smaller immigrant-native gaps.
21 The procedure involves four steps. First, compute the 2-year growth rate in wealth in the pooled HRS sample. Second, estimate a median regression of growth rates on 5-year age dummies, household characteristics, and a set of survey-year dummies. Third, construct predicted growth rates for each age dummy. Fourth, cumulate the predicted growth rates and “anchor” the profiles using the age-70 levels of median wealth.
22 To the extent that immigrant respondents may underreport foreign assets, the convergence of annualized wealth profiles in Chart 3 may partly reflect the fact that immigrants are able to preserve a larger share of comprehensive wealth by financing some retirement spending with the unreported foreign assets.
23 As with comprehensive wealth, the slope of the profiles may reflect other factors as well, such as cohort effects or capital gains in housing and financial assets that disproportionately benefit older households. Given the sharp differences in wealth between recent and earlier immigrants, cohort differences may drive some of the upward slope in annualized wealth. Note, however, that the cohort effect has to involve differences in the growth rate and not just levels of wealth, given that we base the profiles on predicted median growth rates of annualized wealth.
24 For example, suppose that our sample consists of only three households. Household A has an annual wealth level of $20,000 and a growth rate of 5 percent, household B has an annual wealth level of $50,000 and a growth rate of 2 percent, and household C has an annual wealth level of $70,000 and a growth rate of 7 percent. In this example, household B has the median level of annual wealth ($50,000), while household A has the median growth rate of wealth (5 percent). The median wealth trajectories in this case would reflect wealth information from two distinct households, showing a growth rate of 5 percent but a level of $50,000.
25 We take the within-household means to mitigate the contemporaneous correlation between the shares and annualized wealth that is due to slow portfolio rebalancing in the wake of asset price changes.
26 Parents whose children do not live close to them may have to pay for services that their children would otherwise provide. These parents may therefore be less financially prepared than their observed measures would indicate.
27 Appendix B contains tables showing the coefficients on the household characteristic variables, which are generally consistent with expectations.
28 OLS estimates in Appendix B show the same basic pattern at the mean, with somewhat larger differences in implied wealth accumulation for the most recent immigrant cohorts.
29 OLS estimates in Appendix B show a similar pattern, although the magnitudes differ.
30 Beyond the typical challenges faced by the most recent arrivals, members of the post-1984 cohort are much less likely than members of earlier cohorts to have benefited from the amnesty given in the 1986 Immigration Reform and Control Act.
31 Control variables include age, marital status, separate indicators for whether immigrants are married to an immigrant or to a native, whether the respondent speaks English, education, Hispanic origin, whether nonwhite, family size, presence of children, and the natural logarithm of annualized comprehensive wealth. The regressions also control for a full set of year dummies, and standard errors are clustered at the household level.
References
Anacker, Katrin B. 2013. “Immigrating, Assimilating, Cashing In? Analyzing Property Values in Suburbs of Immigrant Gateways.” Housing Studies 28(5): 720–745.
Barrick, Nettie, and Bertram Kestenbaum. 2013. “Totalization Agreements and Totalized Benefits.” Actuarial Note No. 152. Baltimore, MD: Social Security Administration, Office of the Chief Actuary.
Blau, Francine D., Lawrence M. Kahn, Joan Y. Moriarty, and Andre Portela Souza. 2003. “The Role of the Family in Immigrants' Labor-Market Activity: An Evaluation of Alternative Explanations: Comment.” American Economic Review 93(1): 429–447.
Borjas, George J. 1999. “The Economic Analysis of Immigration.” Handbook of Labor Economics 3(A): 1697–1760.
———. 2002. “Homeownership in the Immigrant Population.” Journal of Urban Economics 52(3): 448–476.
———. 2011. “Social Security Eligibility and the Labor Supply of Older Immigrants.” Industrial and Labor Relations Review 64(3): 485–501.
Borjas, George J., and Bernt Bratsberg. 1996. “Who Leaves? The Outmigration of the Foreign-Born.” The Review of Economics and Statistics 78(1): 165–176.
Brown, Jeffrey R., Jeffrey B. Liebman, and Joshua Pollet. 2001. “Estimating Life Tables That Reflect Socioeconomic Differences in Mortality.” In The Distributional Aspects of Social Security and Social Security Reform, edited by Martin Feldstein and Jeffrey B. Liebman. (447–457). Chicago, IL: University of Chicago Press.
Carroll, Christopher D., Byung-Kun Rhee, and Changyong Rhee. 1994. “Are There Cultural Effects on Saving? Some Cross-Sectional Evidence.” The Quarterly Journal of Economics 109(3): 685–699.
———. 1999. “Does Cultural Origin Affect Saving Behavior? Evidence from Immigrants.” Economic Development and Cultural Change 48(1): 33–50.
Chatterjee, Swarnankur, and Velma Zahirovic-Herbert. 2011. “Homeownership and Housing Equity: An Examination of Native-Immigrant Differences in Housing Wealth.” International Advances in Economic Research 17(2): 211–223.
Cobb-Clark, Deborah A., and Vincent A. Hildebrand. 2006. “The Wealth and Asset Holdings of U.S.-Born and Foreign-Born Households: Evidence from SIPP Data.” Review of Income and Wealth 52(1): 17–42.
Cohen, Lee, and Howard Iams. 2007. “Income Adequacy and Social Security Differences Between the Foreign-Born and U.S.-Born.” International Migration Review 41(3): 553–578.
Drew, Rachel Bogardus. 2002. “New Americans, New Homeowners: The Role and Relevance of Foreign Born First-Time Homebuyers in the U.S. Housing Market.” Research Note No. N02-2. Cambridge, MA: Harvard University Joint Center for Housing Studies.
Duleep, Harriet Orcutt. 1994. “Social Security and the Emigration of Immigrants.” Social Security Bulletin 57(1): 37–52.
Duleep, Harriet Orcutt, and Daniel J. Dowhan. 2002. “Insights from Longitudinal Data on the Earnings Growth of U.S. Foreign-Born Men.” Demography 39(3): 485–506.
———. 2008. “Research on Immigrant Earnings.” Social Security Bulletin 68(1): 31–50.
Duleep, Harriet Orcutt, and Mark C. Regets. 1997. “The Decline in Immigrant Entry Earnings: Less Transferable Skills or Lower Ability?” The Quarterly Review of Economics and Finance 37(1): 189–208.
Dupre, Matthew E., Danan Gu, and James W. Vaupel. 2012. “Survival Differences Among Native-Born and Foreign-Born Older Adults in the United States.” PLoS ONE 7(5).
Favreault, Melissa M., and Austin Nichols. 2011. Immigrant Diversity and Social Security: Recent Patterns and Future Prospects. Washington, DC: Urban Institute.
Gustman, Alan L., Olivia S. Mitchell, Andrew A. Samwick, and Thomas L. Steinmeier. 1997. “Pension and Social Security Wealth in the Health and Retirement Study.” NBER Working Paper No. W5912. Cambridge, MA: National Bureau of Economic Research.
Gustman, Alan L., and Thomas L. Steinmeier. 1999. “Effects of Pensions on Savings: Analysis with Data from the Health and Retirement Study.” Carnegie-Rochester Conference Series on Public Policy 50(1): 271–324.
———. 2000. “Social Security Benefits of Immigrants and U.S. Born.” In Issues in the Economics of Immigration, edited by George J. Borjas (309–350). Chicago, IL: University of Chicago Press.
Gustman, Alan L., Thomas L. Steinmeier, and Nahid Tabatabai. 2009. “What the Stock Market Decline Means for the Financial Security and Retirement Choices of the Near-Retirement Population.” NBER Working Paper No. 15435. Cambridge, MA: National Bureau of Economic Research.
———. 2010. Pensions in the Health and Retirement Study. Cambridge, MA: Harvard University Press.
Heim, Bradley T., Ithai Z. Lurie, and Shanthi P. Ramnath. 2012. “Immigrant-Native Differences in Employment-Based Retirement Plan Participation.” Journal of Pension Economics & Finance 11(3): 365–388.
Kerr, Sari Pekkala, and William R. Kerr. 2013. “Economic Impacts of Immigration: A Survey.” Finnish Economic Papers 24(1): 1–32.
Lariscy, Joseph T., Robert A. Hummer, and Mark D. Hayward. 2015. “Hispanic Older Adult Mortality in the United States: New Estimates and an Assessment of Factors Shaping the Hispanic Paradox.” Demography 52(1): 1–14.
Love, David A., Michael G. Palumbo, and Paul A. Smith. 2009. “The Trajectory of Wealth in Retirement.” Journal of Public Economics 93(1–2): 191–208.
Love, David A., Paul A. Smith, and Lucy C. McNair. 2008. “A New Look at the Wealth Adequacy of Older U.S. Households.” Review of Income and Wealth 54(4): 616–642.
Mayr, Karin, and Giovanni Peri. 2008. “Return Migration as a Channel of Brain Gain.” NBER Working Paper No. 14039. Cambridge, MA: National Bureau of Economic Research.
Mehta, Neil K., Irma T. Elo, Michael Engelman, Diane S. Lauderdale, and Bert M. Kestenbaum. 2016. “Life Expectancy Among U.S.-Born and Foreign-Born Older Adults in the United States: Estimates from Linked Social Security and Medicare Data.” Demography 53(4): 1109–1134.
Melly, Blaise. 2005. “Decomposition of Differences in Distribution Using Quantile Regression.” Labour Economics 12(4): 577–590.
Osili, Una Okonkwo, and Anna L. Paulson. 2009. “Immigrants' Access to Financial Services and Asset Accumulation.” In Insufficient Funds: Savings, Assets, Credit, and Banking Among Low-Income Households, edited by Rebecca M. Blank and Michael S. Barr (285–317). New York, NY: Russell Sage Foundation.
Parente, Paulo M. D. C., and João M. C. Santos Silva. 2013. “Quantile Regression with Clustered Data.” Journal of Econometric Methods 5(1): 1–15.
Passel, Jeffrey S., and D'Vera Cohn. 2008. “U.S. Population Projections: 2005–2050.” Washington, DC: Pew Research Center.
Sevak, Purvi, and Lucie Schmidt. 2008. “Immigrant-Native Fertility and Mortality Differentials in the United States.” MRRC Working Paper No. 2008-181. Ann Arbor, MI: University of Michigan Retirement Research Center.
———. 2014. “Immigrants and Retirement Resources.” Social Security Bulletin 74(1): 27–45.
Sierminska, Eva, Pierre-Carl Michaud, and Susann Rohwedder. 2008. “Measuring Wealth Holdings of Older Households in the U.S.: A Comparison Using the HRS, PSID and SCF.” https://pdfs.semanticscholar.org/8b9f/e35f6471498db0ddfe604eb06a9e2e2d7a82.pdf.
Singh, Girish K., and Mohammad Siahpush. 2001. “All-Cause and Cause-Specific Mortality of Immigrants and Native Born in the United States.” American Journal of Public Health 91(3): 392–399.
Smith, James P. 1995. “Racial and Ethnic Differences in Wealth in the Health and Retirement Study.” Journal of Human Resources 30(Suppl.): S158–S183.
[SSA] Social Security Administration. 2015. Annual Statistical Supplement to the Social Security Bulletin, 2014. Publication No. 11700. Washington, DC: SSA.
Wolff, Edward N. 2007. “The Retirement Wealth of the Baby Boom Generation.” Journal of Monetary Economics 54(1): 1–40.