Correlation Patterns between Primary and Secondary Diagnosis Codes in the Social Security Disability Programs
ORES Working Paper No. 113 (released June 2018)
This paper addresses impairment comorbidity among participants in programs that provide disability benefits. Comorbidity in this context is defined as the simultaneous presence of a primary and a secondary medical diagnosis. To this end, I fit a high-dimensional Bayesian multivariate probit model with a 10% random sample of 2009 initial claimants (disabled workers, including individuals concurrently applying for Disability Insurance and Supplemental Security Income). The resulting correlation estimates provide evidence of strong impairment comorbidity patterns at the initial-claim level. Many of the findings mirror the epidemiological evidence, such as associations of diabetes with chronic renal failure, open wounds of a lower limb, peripheral neuropathies, and blindness/low vision. Other results are surprising. For instance, the correlation estimates defy the presumption of high positive association between mental and musculoskeletal-system diagnoses. One interesting cluster of impairments involves diagnoses from five different diagnostic groups that exhibit high positive correlation with one another: (1) organic mental disorders; (2) intracranial (traumatic brain) injury; (3) malignant and benign neoplasms of the brain; (4) late effects of cerebrovascular disease (a circulatory impairment); and (5) multiple diagnoses involving the nervous system/sense organs, such as epilepsy, migraine, late effects of injuries to the nervous system, and other cerebral degenerations/visual disturbances. The findings in this paper suggest that the secondary diagnosis codes are a critical topic for disability research.
The author is with the Office of Economic Analysis and Comparative Studies, Office of Research, Evaluation, and Statistics, Office of Retirement and Disability Policy, Social Security Administration.
Acknowledgments: The author would like to thank Linda Cosme, Robyn Konkel, Judge Gerald Ray, Tim Miller, Matt Messel, and Bernard Wixon for their helpful comments and suggestions.
Working papers are unedited papers prepared by staff in the Office of Research, Evaluation, and Statistics and published on our website as a resource for future research initiatives and to encourage discussion among the wider research community. The findings and conclusions presented in this paper are those of the author and do not necessarily represent the views of the Social Security Administration.
Introduction
DE | disability examiner |
DI | Disability Insurance |
DRF | Disability Research File |
HIV | human immunodeficiency virus |
MCMC | Markov chain Monte Carlo |
MVP | multivariate probit |
SAMC | state agency medical consultant |
SSA | Social Security Administration |
SSI | Supplemental Security Income |
The Social Security Administration (SSA) operates two different programs offering cash benefits to persons with disabilities. Disability Insurance (DI) is funded through payroll tax contributions and aims to protect workers who cannot work because of a disability.1 The general fund of the Treasury finances Supplemental Security Income (SSI), which has no employment or contribution requirements, although its means test imposes strict income and asset limits. By design, SSI provides benefits for aged, blind, and disabled people with limited income and resources.
The SSA-831 Disability Determination and Transmittal form reports the primary and secondary diagnosis, diagnostic group, and impairment code related to the claimant's disability. Upon issuing a disability determination, disability examiners (DEs) and state agency medical consultants (SAMCs)2 assign primary and secondary diagnosis codes most relevant to the determination, choosing among listed impairments that approximately follow the taxonomy established by the International Classification of Diseases, 9th Revision (ICD-9). The 4-digit diagnosis codes often provide the only medical information available to most researchers and are among the most reliable predictors of the resulting initial disability determination outcome.
This paper examines the association between primary and secondary impairments. Understanding which combinations of impairments tend to appear together and which are infrequently linked in disability claims can be useful for a number of reasons. First, it is common practice in disability research to ignore the secondary diagnosis codes, which can result in a distorted picture of diagnostic incidence and can thereby complicate the analysis of determination outcomes. For instance, among 2009 claimants, the most common musculoskeletal impairments (disorders of the back and osteoarthrosis/allied disorders) are far more likely to appear as primary rather than as secondary diagnoses. Conversely, the most frequent mental impairments (affective/mood and anxiety disorders) are most often listed as secondary. Thus, excluding the secondary impairments can lead to underestimating the actual incidence of mental diagnoses in the applicant pool.
In recent decades, there has been growing interest in the study of multimorbidity, which involves the concurrence of multiple disorders in the same person (“comorbidity,” a subset of multimorbidity, refers to cases of two impairments only). Multimorbidity occurs among a majority of the elderly and will likely become increasingly prevalent as the population ages (Marengoni and others 2011; Sinnige and others 2015). Coexisting multiple disorders can have a synergistic effect on health and disability that goes beyond the additive effect of the individual impairments. Thus, the traditional single-disease paradigm in medicine may not provide an adequate picture of health or disability status. From the disability program's perspective, the percentage of initial DI claimants (including those concurrently applying for SSI) with an assigned secondary diagnosis has steadily increased over time. In 1997, a little over half of qualified claimants (workers whose medical evidence was evaluated by SSA) had a secondary diagnosis code (56.3%). By 2010, that percentage had increased to 71.4%.3
Many studies have found a significant effect of multimorbidity on the likelihood of disability, poor quality of life, and high health care costs. A better understanding of multimorbidity can have enormous implications for early intervention and cost containment. Huntley and others (2012) review many of the methods developed by epidemiologists to measure multimorbidity and its consequences. Some of them, such as the Chronic Disease Score and the RxRisk index, rely on pharmacy dispensing data as a proxy for the presence of chronic disease. Others have been derived and validated from large health maintenance organization databases (for example, the Adjusted Clinical Group System), medical practices (such as Duke University's Severity of Illness Index or the Charlson Comorbidity Index), or hospitals (such as the Cumulative Illness Rating Scale). In the context of this study, the sheer volume of the administrative data could prove a valuable alternative source of information to epidemiological researchers.
Another value of understanding the pattern of association between diagnoses is the potential to improve out-of-sample forecasts of program outcomes. After all, a secondary diagnosis may signal additional functional limitations or a more restricted residual functional capacity and, perhaps, a different likelihood of allowance. Applying a naïve Bayes classifier to the data used in this paper, I conducted a simple experiment to determine the predictive ability of individual variables on the initial determination (allowance or denial). Primary diagnosis had the lowest out-of-sample misclassification rate (29.6%), followed by age (31.7%) and secondary diagnosis (34.0%). The latter showed greater predictive capacity than an index of the earnings history of the claimants, the disability determination service office and state of origin, sex, ethnicity, concurrent claim status, reapplicant status, and years of education. The combination of primary and secondary diagnosis codes brought the misclassification rate to 27.1%, while the combination of all variables mentioned above reduced the error rate to 23.9%. Under this scheme, only the primary code and the age of the claimant had greater predictive power of the initial determination outcome than the secondary impairment. Ignoring the secondary diagnoses clearly leaves exploitable information on the table.
Finally, the correlation estimates between the diagnoses may help identify outliers or unusual trends that could merit further scrutiny. Over time, changes in the correlation between primary and secondary impairments could signal events of interest to policymakers, which may include
- Changes in claimant behavior,
- Changes in DE or SAMC interpretation of functional aspects of physical and mental limitations,
- Inconsistencies in applying disability determination guidelines by national or state disability determination services,
- External improvements in medical technology, or
- Epidemiological events.
In a microsimulation context, knowledge of how the diagnoses relate to one another would be useful in generating a synthetic population of future disability claimants with specific diagnostic characteristics.
This paper consists of five sections including this introduction. The second and third sections respectively describe the data and the model. I focused on estimating the correlation between the 100 most common primary impairments for workers who applied for DI benefits in 2009 (including those filing concurrent claims to both DI and SSI). The data are fitted to a Bayesian multivariate probit model, where I rely on an efficient Markov chain Monte Carlo (MCMC) algorithm suitable to large dimensional problems. In addition to providing details on the proposed model specification, the third section discusses some of the assumptions underlying the modeling approach. The fourth section covers the inferential findings. The resulting Bayesian posterior estimates suggest substantial patterns of association (in both magnitude and statistical significance) between many of the primary and secondary diagnoses. Discussion of the correlation estimates is selective in nature, given the involvement of more than 5,000 unique correlation parameters. Finally, the paper concludes with a summary of findings. The appendix provides detailed information about the model, its identification constraints, algorithmic implementation, MCMC convergence diagnostics, and advantages of the Bayes estimator over alternative approaches.
Data
One objective of this paper is to estimate the correlations between primary and secondary diagnoses among initial claims for disability benefits. To that end, I start with the entire universe of claimants to the DI program in 2009, based on an extract of SSA's Disability Research File (DRF). That data file, which includes records for claimants applying concurrently to both DI and SSI, contains a total of 2,748,402 records for 2009. I exclude claims for survivors and dependents and focus on initial disability determinations for claims filed by workers aged 18–65. After technical denials and cases pending an initial decision have also been excluded, the number of remaining observations is 1,637,021.4 Finally, I disregard more than 130 primary impairments to concentrate on the top 100 codes—which account for 96.4% of the claims—yielding a final count of 1,578,354 records.5 In summary, the analysis examines “qualified claimants.” Throughout the paper, I use the terms “claimant” and “qualified claimant” interchangeably to refer to 2009 claimants who cleared step 1 of the disability determination process and thus had their medical evidence evaluated by a DE and a SAMC.6
Table 1 lists the number and share of diagnoses for each of the 19 most common diagnoses and for the single least common diagnosis included in the sample. By definition, all 1,578,354 claimants have a specified primary impairment; 71.1% of them (1,122,520 cases) also have a secondary diagnosis. (The absence of a secondary diagnosis indicates either that no secondary impairment was assigned or that the secondary impairment was not among the 100 most common primary codes.) Combined, the data encompass 2.7 million primary and secondary diagnoses.
Impairment code and diagnosis | Number of diagnoses | Percentage of diagnoses | Cumulative percentage of total | ||||
---|---|---|---|---|---|---|---|
Total | Primary | Secondary | Total | Primary | Secondary | ||
Total | 2,700,874 | 1,578,354 | 1,122,520 | 100.00 | 100.00 | 100.00 | 100.00 |
2960 - Affective and mood disorders | 480,650 | 223,934 | 256,716 | 17.80 | 14.19 | 22.87 | 17.80 |
7240 - Disorders of back | 393,243 | 301,409 | 91,834 | 14.56 | 19.10 | 8.18 | 32.36 |
7150 - Osteoarthrosis and allied disorders | 156,852 | 102,201 | 54,651 | 5.81 | 6.48 | 4.87 | 38.16 |
3000 - Anxiety and obsessive-compulsive disorders | 135,672 | 37,340 | 98,332 | 5.02 | 2.37 | 8.76 | 43.19 |
2500 - Diabetes | 114,773 | 52,512 | 62,261 | 4.25 | 3.33 | 5.55 | 47.44 |
4010 - Essential hypertension | 107,496 | 24,646 | 82,850 | 3.98 | 1.56 | 7.38 | 51.42 |
2780 - Obesity | 69,425 | 18,719 | 50,706 | 2.57 | 1.19 | 4.52 | 53.99 |
7280 - Disorders of muscle, ligament, and fascia | 68,973 | 41,123 | 27,850 | 2.55 | 2.61 | 2.48 | 56.54 |
2940 - Organic mental disorders | 67,096 | 42,739 | 24,357 | 2.48 | 2.71 | 2.17 | 59.02 |
7160 - Other and unspecified arthropathies | 66,921 | 39,876 | 27,045 | 2.48 | 2.53 | 2.41 | 61.50 |
4960 - Chronic pulmonary insufficiency | 53,761 | 37,790 | 15,971 | 1.99 | 2.39 | 1.42 | 63.49 |
4140 - Chronic ischemic heart disease | 46,756 | 33,319 | 13,437 | 1.73 | 2.11 | 1.20 | 65.22 |
8270 - Fractures of lower limb | 34,910 | 26,699 | 8,211 | 1.29 | 1.69 | 0.73 | 66.52 |
4380 - Late effects of cerebrovascular disease | 34,613 | 28,562 | 6,051 | 1.28 | 1.81 | 0.54 | 67.80 |
2950 - Schizophrenic and other psychotic disorders | 33,534 | 27,787 | 5,747 | 1.24 | 1.76 | 0.51 | 69.04 |
4930 - Asthma | 32,631 | 15,531 | 17,100 | 1.21 | 0.98 | 1.52 | 70.25 |
3450 - Epilepsy | 32,528 | 22,693 | 9,835 | 1.20 | 1.44 | 0.88 | 71.45 |
5710 - Chronic liver disease and cirrhosis | 30,337 | 20,017 | 10,320 | 1.12 | 1.27 | 0.92 | 72.58 |
3010 - Personality disorders | 28,291 | 6,142 | 22,149 | 1.05 | 0.39 | 1.97 | 73.62 |
. . . | . . . | . . . | . . . | . . . | . . . | . . . | . . . |
1510 - Stomach cancer (malignant) | 1,872 | 1,714 | 158 | 0.07 | 0.11 | 0.01 | 100.00 |
SOURCE: Author's calculations using DRF 100% extract. | |||||||
NOTES: Data are for applicants who cleared step 1 of the disability determination process.
Diagnoses identified as "other" may be defined in the context of impairments not shown in this table.
. . . = omitted (20th–99th most common diagnoses).
|
Affective/mood disorders appear as the primary impairment for 223,934 claimants and as the secondary impairment for another 256,716 applicants. This condition represents 14.2% and 22.9% of all primary and secondary diagnoses, respectively. By contrast, a back disorder is more frequently assigned as a primary than a secondary impairment; in fact, it is the most common primary diagnosis. However, when both primary and secondary impairments are considered, affective/mood disorders constitute 17.8% of all impairments, while disorders of the back constitute 14.6% of the total.
For comparative purposes, Table 1 includes malignant cancer of the stomach—the impairment with the lowest incidence in the sample—with 1,872 occurrences (0.07% of all diagnoses). Table 1 also features the cumulative share of diagnoses that results from the addition of each successive disorder. The two most common impairments (affective/mood disorders and disorders of the back) dominate the other 98 disorders in terms of frequency, as they together represent close to one-third of all diagnoses. Interestingly, the third and fourth most frequent impairments (osteoarthrosis/allied disorders and anxiety disorders, respectively) also correspond to the musculoskeletal system and mental disorder diagnostic groups. The six most common impairments account for 51.4% of all assigned diagnoses, and the top 19 diagnoses account for 73.6% of the total.
Later sections of this paper describe many of the data features in detail, particularly in discussing the correlation estimates. Nevertheless, for reference purposes, Table 2 illustrates the distribution of all diagnoses (primary and secondary combined) by diagnostic group. The mental and musculoskeletal body systems are by far the two largest categories, accounting for roughly 30% and 27% of all diagnoses, respectively. Circulatory impairments are the third largest category (10.6%), with essential hypertension (see Table 1) constituting almost 42% of all circulatory diagnoses.
Diagnostic group | Number | Percentage |
---|---|---|
Total | 2,745,939 | 100.00 |
Mental disorders | 836,136 | 30.45 |
Musculoskeletal system and connective tissue diseases | 740,082 | 26.95 |
Circulatory system diseases | 291,780 | 10.63 |
Nervous system and sense organ diseases | 202,120 | 7.36 |
Endocrine, nutritional, and metabolic diseases | 196,476 | 7.16 |
Injuries | 129,260 | 4.71 |
Neoplasms | 119,233 | 4.34 |
Respiratory system diseases | 101,737 | 3.70 |
Digestive system diseases | 64,846 | 2.36 |
Genitourinary system diseases | 29,472 | 1.07 |
Infectious and parasitic diseases | 24,067 | 0.88 |
Blood and blood-forming organ diseases | 5,038 | 0.18 |
Skin and subcutaneous tissue diseases | 4,813 | 0.18 |
Congenital anomalies | 879 | 0.03 |
SOURCE: Author's calculations using DRF 100% extract. | ||
NOTES: Data are for applicants who cleared step 1 of the disability determination process.
The total count includes 45,065 cases with identical impairment codes entered as the primary and secondary diagnosis for a given claimant. The total count differs from that shown in Table 1, which excludes those duplicated diagnoses.
|
The nervous system/sense organ diseases group is equal in size to the endocrine/nutritional/metabolic disease group; each accounts for about 7% of the diagnoses. For the latter group, diabetes and obesity respectively account for 60.1% and 36.4% of the disorders. Injuries account for 4.7% of the diagnoses, while cancer and respiratory disorders constitute 4.3% and 3.7% of the impairments, respectively. Digestive disorders encompass another 2.4% of the total, with half of those diagnoses involving chronic liver disease/cirrhosis. A little more than 1% of the diagnoses correspond to the genitourinary body system, where almost 80% of cases involve chronic renal failure (not among the 19 most common diagnoses shown in Table 1). Infectious/parasitic diseases account for 0.9% of the impairments (mostly symptomatic and asymptomatic human immunodeficiency virus [HIV]).
The Model
For the purposes of this study, I treat the pairwise combination of the 100 diagnoses in the data within a multivariate probit (MVP) modeling framework. This model is often used to analyze binary correlated data—for instance, with multiple response surveys, where the respondent furnishes a binary yes/no answer to a set of p questions. The MVP model provides an appealing and flexible approach to investigate any meaningful relationships underlying the categorical data, as it can accommodate an arbitrarily complicated correlation structure and its parameters are easy to interpret. Until recently, however, its application was restricted to problems with a small number of dimensions p because of the computational difficulties involved with greater numbers of p.
The appendix of this paper provides technical details on the model, its identification constraints, and the algorithms implemented. I rely on the efficient MCMC sampler suggested by Edwards and Allenby (2003) to estimate a high dimensional Bayesian MVP model. Working with a 10% random sample of 157,835 observations, I derive posterior estimates of all the model's parameters, including 5,356 unique correlations describing the patterns of association between all the binary variables. The sampler proposed by Edwards and Allenby is particularly suitable for problems where there is a large number of responses p and the dependencies are poorly represented by a small number of underlying factors. The appendix expands on this point and discusses some of the advantages of the Bayes estimator over alternative approaches.
Model Specification
One useful feature of the MVP model is its ability to generate predictions of conditional probabilities. For this reason, I decided to augment the set of 100 binary responses representing the combination of diagnoses among the dependent variables with four additional binary indicators: sex (taking a value of 1 if male and 0 otherwise), whether reapplying (1 if the claimant had applied for DI benefits within the previous 10 years), whether filing concurrently (1 if applying for DI and SSI simultaneously), and initial determination (1 for an allowance). By moving the initial determination to the dependent variable column, I can estimate the probability of an initial allowance for a claimant, conditional on the specific combination of primary and secondary diagnoses, sex, concurrent application status, and application history.
A set of three predictor variables are treated as explanatory covariates: (1) an intercept, (2) the claimant's age at filing in 2009, and (3) the square of the applicant's age. The third predictor helps capture nonlinear behavior with respect to age (see Meseguer 2013, Chart 5). The specified MVP model results in 104 × 3 = 312 separate regression coefficients and a 104 × 104 correlation matrix with 5,356 unique correlation parameters that describe the pairwise patterns of association between the binary variables.
Assumptions, Interpretation, and Caveats
One fundamental goal of this paper is to gain a better understanding of the interaction between primary and secondary diagnoses. The specified MVP model accomplishes this by simultaneously modeling the association between 104 binary variables.7 The resulting estimated correlations between two impairments must be understood in terms of their relative frequency in the sample. For example, high positive correlation suggests that the two impairments combine far more often than expected, given their overall share in the data. For the most part, I report any correlation estimates of a certain magnitude that are statistically significant and, at times, I suggest a plausible explanation for the finding. In this context, it is important to recognize the various reasons why disease incidence rates and correlation patterns found in the general population may not reflect those among the pool of qualified disability claimants.
DI applicants do not necessarily represent a random sample of individuals with disabilities in the general population. The decision to apply itself acts as a filter, as not every person with a health impairment or diminished functional capacity decides to stop working. The volume of technical denials introduces a second filtering step. A substantial number of applicants are denied without any evaluation of the medical evidence because they fail to meet the substantial gainful activity limit, the contributory requirements of the DI program, or the asset limits imposed under SSI. In addition, the available data are censored because combinations of only two impairments are recorded. This could have a biasing effect if, for example, DEs systematically choose the same two out of three related conditions in the medical evidence when filling the primary and secondary diagnosis fields.
The model specification assumes that the order of the impairments does not matter. In other words, the model does not differentiate if a specific disorder appears as a primary or as a secondary diagnosis; it only matters that they combine. The implicit assumption is that, all else being equal, a claim with primary diagnosis A and secondary diagnosis B is similar to another with the order of the impairments reversed.
According to SSA's Program Operations Manual System, “the primary diagnosis in an allowance refers to the basic condition that rendered the individual disabled, or in a denial, the one that the evidence shows to have the most significant effect on the person's ability to work” (SSA 2017). Likewise, the secondary diagnosis should be the second most severe condition following the primary impairment. Specific exceptions to this rule apply to findings of material drug or alcohol addiction, symptomatic HIV, and statutory blindness.
One might expect the most severe impairment to appear as primary. For instance, the diagnostic group of impairments with the highest mortality is malignant neoplasms, which are much more likely to appear in the primary than in the secondary field. In addition, more than half of the neoplasms that appear as secondary impairments are associated with another malignant neoplasm as the primary diagnosis. On the other hand, alternative considerations, such as the strength of the evidence submitted by the claimant, could play a role in determining the order of the impairments. It is also possible that some DEs and SAMCs misclassify the diagnoses. The key question is whether identifying the order in which two diagnoses appear in a claim carries useful predictive content. The modeling framework for this analysis effectively renders the question moot.8
Finally, three of the 100 impairments in the sample are sex-specific: malignant neoplasms of the prostate, of the ovary, and of the uterus. This means that a few of the responses are actually mutually exclusive. In other words, the model assigns a tiny, but nonzero, probability to an outcome that is actually impossible, such as having a female claimant with a prostate cancer diagnosis or an applicant with cancers of both the uterus and the prostate. Technically, there is a difference between an outcome that is possible but rare enough that it is not observed in the data and an outcome that is not possible.
For practical purposes, this is not an issue of concern. The sizable negative magnitudes of the correlation estimates associated with these very few mutually exclusive impairment combinations guarantee the prediction of extremely small incidence probabilities. In a microsimulation context, this situation can be handled case by case, by simply discarding the rare simulated draw that represents a violation. Alternatively, I could have merged the three sex-specific impairments into a single category (say, “malignant neoplasms of the reproductive system”), but I saw greater value in preserving the original diagnoses.
Inferential Results
This section presents correlation estimates and related statistics calculated using the MVP model fitted to a 10% random sample of the DRF data (157,835 observations). I first examine correlations between diagnoses associated with each of the four nondiagnosis binary variables (initial allowance, sex, reapplicant status, and concurrent DI and SSI filing status). Then I present the correlation estimates between impairments. Note, however, that all descriptive statistics—such as counts or proportions between any two variables—are based on the entire population of qualified claimants (1,578,354 observations).
Analysis of the posterior estimates obtained from the model reveals that about 79% of the regression coefficients and 26.3% of the correlation parameters (1,407 of them) have t-statistics with an absolute value greater than 2. The latter measure simply refers to the ratio of the posterior mean over the posterior standard deviation. A more precise alternative Bayesian measure of the statistical significance of a parameter results from estimating the posterior probability that its value has the same sign as its mean.9 In this case, 33% of the correlations have at least 90% of their probability mass with the same sign as their mean. This measure assesses uncertainty of the direction of an effect, but not necessarily its magnitude. Overall, 15.6% of the correlations (838 of them) have posterior means with an absolute magnitude of at least 0.10. These findings suggest a substantial pattern of association (in both magnitude and statistical significance) between many of the primary and secondary diagnoses.10
Variables Correlated with an Initial Allowance
Given the large number of statistically significant correlation estimates, discussion of the findings is necessarily selective. In general, I present results for correlation parameters with a mean of at least 0.10 in absolute value and a high t-statistic. Table 3 presents estimates for some of the binary variables associated with an initial allowance, with detail for 54 diagnoses. Along with posterior mean estimates of the correlations and corresponding t-statistics, Table 3 shows the numerical standard error associated with the posterior mean, which enables one to infer the number of statistically significant digits of the posterior mean correlation estimate.11 The table also shows the initial-claim allowance rate associated with the particular impairment.
Diagnostic group, impairment code, and diagnosis | Initial allowances as a percentage of applicants | Correlation (posterior mean) | t-statistic | Numerical standard error |
---|---|---|---|---|
Concurrent | 26.85 | -0.2281 | -55.45 | 0.000090 |
Reapplicant | 28.51 | -0.1642 | -36.93 | 0.000102 |
Neoplasms (malignant) | ||||
1620 - Lung cancer | 94.33 | 0.4995 | 44.00 | 0.000609 |
1550 - Liver cancer | 96.48 | 0.4722 | 24.50 | 0.001680 |
1570 - Pancreatic cancer | 96.58 | 0.4581 | 18.41 | 0.002691 |
1910 - Brain cancer | 92.76 | 0.4570 | 25.94 | 0.001219 |
1500 - Esophageal cancer | 96.25 | 0.4219 | 16.81 | 0.002370 |
1950 - Soft tissue tumor of the head and neck | 86.33 | 0.3619 | 21.80 | 0.001161 |
1830 - Ovarian cancer | 86.29 | 0.3444 | 15.96 | 0.001767 |
1510 - Stomach cancer | 89.05 | 0.3187 | 11.38 | 0.002440 |
2070 - Leukemias | 80.68 | 0.2978 | 16.41 | 0.000928 |
2030 - Multiple myeloma | 84.87 | 0.2946 | 10.84 | 0.002558 |
1890 - Kidney cancer | 78.68 | 0.2840 | 13.42 | 0.001718 |
1790 - Uterine cancer | 76.98 | 0.2621 | 10.55 | 0.002376 |
1530 - Colon cancer | 73.02 | 0.2567 | 20.63 | 0.000445 |
1740 - Breast cancer | 58.39 | 0.1627 | 15.39 | 0.000500 |
2020 - Lymphoma | 59.75 | 0.1357 | 8.88 | 0.000596 |
Mental disorders | ||||
3180 - Intellectual disability | 72.26 | 0.4301 | 40.50 | 0.000533 |
2950 - Schizophrenic and other psychotic disorders | 55.50 | 0.2937 | 33.70 | 0.000286 |
2990 - Autistic disorders | 57.52 | 0.2861 | 12.12 | 0.001751 |
2940 - Organic mental disorders | 46.97 | 0.1501 | 20.81 | 0.000189 |
2960 - Affective and mood disorders | 24.43 | -0.1874 | -42.59 | 0.000092 |
Circulatory system diseases | ||||
4380 - Late effects of cerebrovascular disease | 68.88 | 0.2351 | 27.46 | 0.000282 |
4160 - Chronic pulmonary heart disease | 67.28 | 0.1700 | 7.93 | 0.001333 |
4280 - Heart failure | 61.53 | 0.1620 | 16.48 | 0.000380 |
4430 - Peripheral vascular disease | 65.23 | 0.1532 | 11.90 | 0.000491 |
4010 - Essential hypertension | 26.98 | -0.2250 | -35.34 | 0.000200 |
Injuries | ||||
8060 - Fracture of vertebral column | 67.50 | 0.2907 | 14.17 | 0.001648 |
8540 - Intracranial (traumatic brain) injury | 54.47 | 0.1684 | 10.01 | 0.001042 |
8480 - Sprains and strains—all types | 12.66 | -0.2121 | -17.33 | 0.000510 |
8180 - Fractures of upper limb | 22.63 | -0.1275 | -10.19 | 0.000670 |
8390 - Dislocations—all types | 22.31 | -0.1200 | -4.92 | 0.001701 |
8840 - Open soft tissue wound of upper limb | 21.36 | -0.1029 | -4.28 | 0.002034 |
Musculoskeletal system and connective tissue diseases | ||||
7240 - Disorders of back | 27.70 | -0.2297 | -51.04 | 0.000115 |
7280 - Disorders of muscle, ligament, and fascia | 26.16 | -0.1580 | -21.17 | 0.000214 |
7160 - Other and unspecified arthropathies | 30.46 | -0.1025 | -13.80 | 0.000157 |
Infectious and parasitic diseases | ||||
0430 - Symptomatic HIV | 50.32 | 0.1915 | 14.53 | 0.000675 |
0440 - Asymptomatic HIV | 9.13 | -0.1729 | -9.28 | 0.001217 |
Nervous system and sense organ diseases | ||||
3350 - Anterior horn cell disease | 97.25 | 0.3843 | 10.44 | 0.004066 |
3360 - Other spinal cord disorders | 74.37 | 0.2619 | 12.65 | 0.001295 |
3590 - Muscular dystrophies | 63.35 | 0.2271 | 8.80 | 0.002349 |
3430 - Cerebral palsy | 61.29 | 0.1975 | 8.85 | 0.002012 |
9070 - Late effects of injuries to the nervous system | 60.06 | 0.1907 | 12.00 | 0.000739 |
3320 - Parkinson's disease | 77.82 | 0.1884 | 10.12 | 0.001137 |
3310 - Other cerebral degenerations | 63.35 | 0.1636 | 7.79 | 0.001656 |
3400 - Multiple sclerosis | 50.90 | 0.1597 | 13.73 | 0.000469 |
3690 - Blindness and low vision | 47.47 | 0.1063 | 9.81 | 0.000380 |
3620 - Other retina disorders | 53.35 | 0.1038 | 5.74 | 0.001031 |
3460 - Migraine | 16.56 | -0.1326 | -10.98 | 0.000452 |
3450 - Epilepsy | 15.53 | -0.1659 | -16.07 | 0.000391 |
Genitourinary system diseases | ||||
5850 - Chronic renal failure | 83.87 | 0.4195 | 42.85 | 0.000538 |
5990 - Other urinary tract disorders | 20.82 | -0.1405 | -6.70 | 0.001172 |
Endocrine, nutritional, and metabolic diseases | ||||
2460 - All disorders of thyroid | 14.61 | -0.1537 | -9.30 | 0.001105 |
2500 - Diabetes | 32.01 | -0.1529 | -24.61 | 0.000147 |
Digestive system diseases | ||||
5530 - Hernias | 20.16 | -0.1528 | -7.22 | 0.001487 |
5690 - Other gastrointestinal system disorders | 20.38 | -0.1520 | -12.55 | 0.000304 |
SOURCE: Author's calculations using DRF 100% extract (for descriptive statistics) and MVP estimation model fitted to a DRF 10% random sample (for correlation estimates). | ||||
NOTES: Correlation estimates are selected for inclusion on the basis of having absolute values for the posterior mean of at least 0.10 and, generally, a t-statistic greater than 2.00.
Data are for applicants who cleared step 1 of the disability determination process.
Diagnoses are arranged by estimated correlation value. Negative correlations are listed by absolute value.
Diagnoses identified as "other" may be defined in the context of impairments not shown in this table.
|
The proportion of initial allowances among all 2009 qualified claimants is 37.9% (not shown). However, claimants that apply concurrently for DI and SSI have a lower share of initial allowances at 26.9%. Likewise, only 28.5% of claimants with a history of prior applications (reapplicants) receive an initial allowance. Table 3 shows the resulting negative correlation estimates.
Malignant Neoplasms
Among the diagnostic groups, neoplasms generally have very high initial allowance rates, leading to high positive estimates of the correlation with an initial allowance. For instance, 94.3% of claimants that have lung cancer as either a primary or a secondary impairment receive an initial allowance. The estimated posterior mean correlation of lung cancer with initial allowance is 0.50. Malignant neoplasms of the liver, pancreas, brain, and esophagus are associated with mean correlations above 0.40, while cancers of the ovaries, stomach, and soft tissue of the head and neck have mean correlations above 0.30. Other cancers with high positive correlation (greater than 0.20) with an initial allowance include leukemia, multiple myeloma, and neoplasms of the kidney, uterus, and colon; each has an initial allowance rate exceeding 70%. It is worth noticing the degree of variation in the share of initial allowances among the neoplasms. For example, breast cancer has a substantially lower initial allowance rate (58%) than do cancers of the lung, liver, or pancreas (all above 90%).
The probability of an initial allowance as a function of age, conditional on having a malignant neoplasm of the kidney as either a primary or a secondary diagnosis, appears as Panel A in Chart 1. The solid lines present a 90% credible interval of the model's estimated probabilities of an initial allowance, and the broken line denotes the estimated median probability. The squares indicate the corresponding data proportions, based on all 2009 qualified claimants. Because cancers tend toward relatively late ages of onset, the proportion of allowances varies considerably at the youngest ages because of the small number of observations (of more than 3,400 diagnoses, only 30 are for claimants aged 25 or younger). For instance, the share of allowances is 0% at ages 19 and 21, but it is 100% at age 20. This illustrates one of the advantages of using a formal statistical model instead of relying on direct observations to estimate underlying probabilities. Clearly, the model produces more reasonable conditional probability estimates of allowance at these ages, even as it is fitted with a subset of the data.
Estimated incidence probabilities of allowance of initial claims for disability benefits, by age and selected diagnosis, 2009
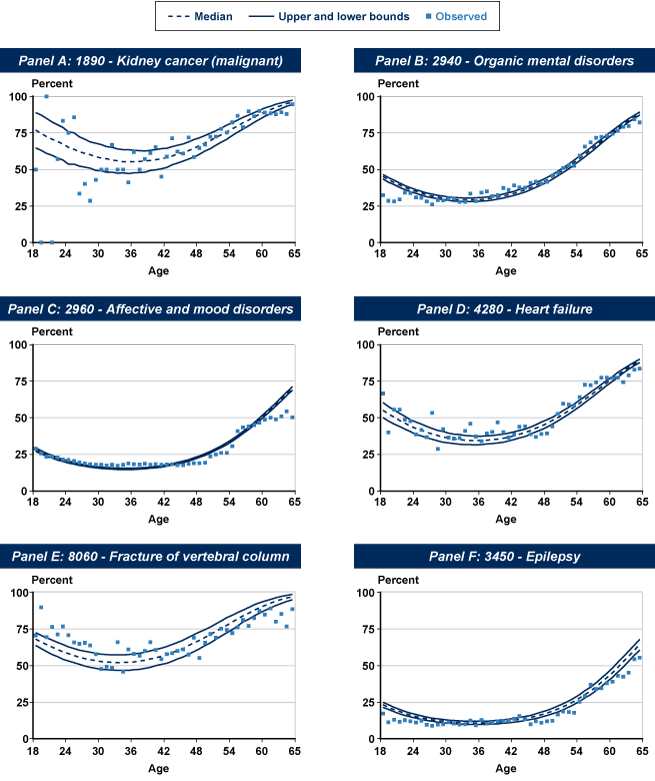
Age | Median | Lower bound | Upper bound | Observed |
---|---|---|---|---|
Panel A: 1890 - Kidney cancer (malignant) | ||||
18 | 76.92 | 64.71 | 88.89 | 50.00 |
19 | 74.68 | 63.00 | 87.14 | 0.00 |
20 | 72.50 | 61.05 | 84.89 | 100.00 |
21 | 70.74 | 59.76 | 82.44 | 0.00 |
22 | 68.97 | 57.74 | 80.65 | 57.14 |
23 | 67.39 | 56.40 | 78.71 | 83.33 |
24 | 65.24 | 53.71 | 75.74 | 75.00 |
25 | 63.70 | 53.58 | 75.00 | 85.71 |
26 | 62.26 | 52.40 | 72.22 | 33.33 |
27 | 61.05 | 51.64 | 70.78 | 40.00 |
28 | 60.00 | 50.85 | 68.80 | 28.57 |
29 | 58.73 | 49.21 | 67.94 | 42.86 |
30 | 57.70 | 49.15 | 66.97 | 50.00 |
31 | 57.24 | 48.18 | 65.72 | 50.00 |
32 | 56.52 | 48.18 | 65.23 | 66.67 |
33 | 55.91 | 47.49 | 63.99 | 50.00 |
34 | 55.36 | 47.79 | 63.67 | 50.00 |
35 | 55.32 | 47.33 | 63.51 | 41.18 |
36 | 55.37 | 47.64 | 63.02 | 61.90 |
37 | 55.56 | 48.00 | 62.93 | 50.00 |
38 | 55.66 | 48.17 | 62.74 | 57.14 |
39 | 56.16 | 49.24 | 63.03 | 61.29 |
40 | 56.71 | 49.79 | 63.51 | 65.38 |
41 | 57.35 | 50.39 | 64.29 | 45.16 |
42 | 58.13 | 51.49 | 64.51 | 58.70 |
43 | 59.27 | 53.12 | 65.73 | 71.43 |
44 | 60.51 | 54.25 | 66.56 | 62.26 |
45 | 61.77 | 55.73 | 67.50 | 60.81 |
46 | 62.96 | 57.20 | 68.79 | 71.79 |
47 | 64.38 | 58.49 | 70.44 | 58.43 |
48 | 66.25 | 60.33 | 71.57 | 64.77 |
49 | 68.12 | 62.58 | 73.29 | 66.98 |
50 | 69.79 | 64.31 | 74.89 | 72.32 |
51 | 71.81 | 66.50 | 76.51 | 72.48 |
52 | 73.69 | 68.55 | 78.18 | 77.71 |
53 | 75.71 | 70.69 | 80.27 | 75.32 |
54 | 77.78 | 73.20 | 81.95 | 82.28 |
55 | 79.74 | 75.47 | 83.88 | 86.71 |
56 | 81.85 | 77.64 | 85.66 | 78.92 |
57 | 83.88 | 79.95 | 87.29 | 89.70 |
58 | 85.75 | 82.26 | 88.92 | 86.43 |
59 | 87.61 | 84.29 | 90.52 | 90.05 |
60 | 89.40 | 86.37 | 91.96 | 88.89 |
61 | 91.00 | 88.20 | 93.21 | 88.39 |
62 | 92.43 | 89.99 | 94.53 | 87.72 |
63 | 93.77 | 91.65 | 95.59 | 89.19 |
64 | 94.97 | 93.21 | 96.54 | 87.93 |
65 | 96.00 | 94.36 | 97.33 | 94.64 |
Panel B: 2940 - Organic mental disorders | ||||
18 | 45.11 | 43.46 | 46.64 | 32.51 |
19 | 43.03 | 41.34 | 44.53 | 28.67 |
20 | 41.08 | 39.57 | 42.56 | 28.27 |
21 | 39.32 | 37.86 | 40.73 | 29.52 |
22 | 37.72 | 36.30 | 39.07 | 34.16 |
23 | 36.28 | 34.93 | 37.57 | 33.89 |
24 | 34.96 | 33.62 | 36.27 | 30.88 |
25 | 33.82 | 32.49 | 34.97 | 30.69 |
26 | 32.77 | 31.48 | 33.95 | 28.15 |
27 | 31.86 | 30.66 | 33.11 | 26.12 |
28 | 31.13 | 29.91 | 32.32 | 29.17 |
29 | 30.49 | 29.30 | 31.74 | 28.96 |
30 | 30.00 | 28.81 | 31.21 | 29.94 |
31 | 29.62 | 28.42 | 30.87 | 30.28 |
32 | 29.41 | 28.15 | 30.61 | 28.01 |
33 | 29.29 | 28.08 | 30.47 | 27.94 |
34 | 29.23 | 28.05 | 30.50 | 33.43 |
35 | 29.39 | 28.13 | 30.65 | 28.38 |
36 | 29.61 | 28.32 | 30.98 | 34.24 |
37 | 29.98 | 28.70 | 31.26 | 35.16 |
38 | 30.48 | 29.14 | 31.79 | 31.86 |
39 | 31.05 | 29.77 | 32.40 | 32.57 |
40 | 31.81 | 30.37 | 33.21 | 37.27 |
41 | 32.68 | 31.33 | 34.17 | 35.87 |
42 | 33.72 | 32.37 | 35.14 | 39.10 |
43 | 34.87 | 33.42 | 36.36 | 37.82 |
44 | 36.14 | 34.66 | 37.68 | 37.53 |
45 | 37.60 | 36.12 | 39.16 | 40.69 |
46 | 39.22 | 37.71 | 40.78 | 41.82 |
47 | 41.03 | 39.50 | 42.60 | 40.36 |
48 | 42.92 | 41.34 | 44.51 | 41.40 |
49 | 44.96 | 43.37 | 46.55 | 45.93 |
50 | 47.23 | 45.64 | 48.83 | 47.71 |
51 | 49.58 | 48.03 | 51.12 | 50.98 |
52 | 52.10 | 50.46 | 53.71 | 52.78 |
53 | 54.74 | 53.15 | 56.37 | 52.45 |
54 | 57.51 | 55.98 | 59.13 | 59.52 |
55 | 60.39 | 58.71 | 61.88 | 65.67 |
56 | 63.26 | 61.82 | 64.91 | 68.53 |
57 | 66.31 | 64.74 | 67.87 | 71.70 |
58 | 69.29 | 67.87 | 70.75 | 72.20 |
59 | 72.30 | 70.81 | 73.64 | 72.97 |
60 | 75.25 | 73.92 | 76.67 | 74.34 |
61 | 78.16 | 76.85 | 79.41 | 76.62 |
62 | 80.93 | 79.71 | 82.23 | 79.15 |
63 | 83.61 | 82.39 | 84.75 | 79.65 |
64 | 86.07 | 85.01 | 87.12 | 84.30 |
65 | 88.31 | 87.30 | 89.34 | 82.11 |
Panel C: 2960 - Affective and mood disorders | ||||
18 | 28.08 | 26.96 | 29.45 | 28.96 |
19 | 26.32 | 25.29 | 27.49 | 25.67 |
20 | 24.73 | 23.76 | 25.78 | 23.47 |
21 | 23.25 | 22.37 | 24.20 | 23.25 |
22 | 21.95 | 21.10 | 22.78 | 22.99 |
23 | 20.76 | 20.07 | 21.52 | 21.41 |
24 | 19.73 | 19.11 | 20.43 | 20.99 |
25 | 18.84 | 18.26 | 19.46 | 20.39 |
26 | 18.03 | 17.48 | 18.62 | 19.51 |
27 | 17.34 | 16.83 | 17.84 | 18.88 |
28 | 16.74 | 16.24 | 17.24 | 18.43 |
29 | 16.23 | 15.79 | 16.72 | 17.91 |
30 | 15.82 | 15.39 | 16.28 | 17.86 |
31 | 15.52 | 15.09 | 15.93 | 17.44 |
32 | 15.29 | 14.89 | 15.69 | 17.96 |
33 | 15.14 | 14.73 | 15.53 | 17.14 |
34 | 15.04 | 14.66 | 15.44 | 17.85 |
35 | 15.05 | 14.67 | 15.44 | 18.69 |
36 | 15.13 | 14.70 | 15.56 | 18.40 |
37 | 15.30 | 14.89 | 15.73 | 18.01 |
38 | 15.55 | 15.13 | 15.96 | 18.63 |
39 | 15.88 | 15.45 | 16.28 | 17.83 |
40 | 16.30 | 15.85 | 16.72 | 18.27 |
41 | 16.78 | 16.34 | 17.25 | 17.91 |
42 | 17.39 | 16.92 | 17.84 | 18.07 |
43 | 18.09 | 17.62 | 18.61 | 18.25 |
44 | 18.92 | 18.41 | 19.35 | 17.70 |
45 | 19.82 | 19.31 | 20.36 | 17.72 |
46 | 20.88 | 20.34 | 21.40 | 18.47 |
47 | 22.06 | 21.55 | 22.57 | 18.90 |
48 | 23.36 | 22.84 | 23.94 | 19.05 |
49 | 24.83 | 24.27 | 25.40 | 19.25 |
50 | 26.47 | 25.86 | 27.04 | 23.69 |
51 | 28.27 | 27.67 | 28.86 | 24.97 |
52 | 30.19 | 29.58 | 30.81 | 25.94 |
53 | 32.37 | 31.69 | 33.01 | 25.98 |
54 | 34.67 | 34.03 | 35.34 | 30.58 |
55 | 37.15 | 36.42 | 37.86 | 40.91 |
56 | 39.84 | 39.14 | 40.63 | 43.31 |
57 | 42.70 | 41.94 | 43.54 | 44.16 |
58 | 45.72 | 44.89 | 46.59 | 44.93 |
59 | 48.91 | 48.08 | 49.85 | 46.64 |
60 | 52.26 | 51.27 | 53.22 | 48.69 |
61 | 55.72 | 54.72 | 56.69 | 50.09 |
62 | 59.18 | 58.17 | 60.21 | 48.93 |
63 | 62.77 | 61.60 | 63.89 | 50.90 |
64 | 66.39 | 65.22 | 67.57 | 54.35 |
65 | 69.97 | 68.78 | 71.15 | 50.28 |
Panel D: 4280 - Heart failure | ||||
18 | 55.00 | 50.05 | 60.39 | 66.67 |
19 | 52.67 | 47.71 | 57.70 | 40.00 |
20 | 50.39 | 45.72 | 55.30 | 55.56 |
21 | 48.24 | 43.98 | 53.00 | 55.56 |
22 | 46.33 | 42.13 | 50.90 | 48.57 |
23 | 44.36 | 40.58 | 48.69 | 47.73 |
24 | 42.79 | 38.59 | 46.99 | 38.46 |
25 | 41.55 | 37.56 | 45.59 | 41.67 |
26 | 40.00 | 36.26 | 43.96 | 36.76 |
27 | 38.94 | 35.28 | 42.60 | 53.16 |
28 | 37.82 | 34.29 | 41.32 | 28.74 |
29 | 36.82 | 33.33 | 40.50 | 42.11 |
30 | 36.22 | 32.76 | 39.49 | 36.36 |
31 | 35.55 | 32.22 | 38.79 | 35.90 |
32 | 35.09 | 31.76 | 38.47 | 36.29 |
33 | 34.64 | 31.65 | 37.95 | 41.09 |
34 | 34.47 | 31.73 | 37.54 | 45.86 |
35 | 34.38 | 31.52 | 37.43 | 37.28 |
36 | 34.59 | 31.59 | 37.34 | 33.85 |
37 | 34.74 | 32.01 | 37.54 | 39.20 |
38 | 35.04 | 32.27 | 37.76 | 40.28 |
39 | 35.41 | 32.70 | 38.22 | 46.73 |
40 | 36.05 | 33.26 | 38.85 | 40.14 |
41 | 36.77 | 34.16 | 39.52 | 36.56 |
42 | 37.68 | 35.00 | 40.33 | 38.48 |
43 | 38.78 | 36.18 | 41.28 | 43.94 |
44 | 39.88 | 37.27 | 42.21 | 44.11 |
45 | 41.26 | 38.72 | 43.61 | 38.73 |
46 | 42.82 | 40.32 | 45.18 | 36.84 |
47 | 44.37 | 41.83 | 46.96 | 39.01 |
48 | 46.18 | 43.61 | 48.63 | 39.14 |
49 | 48.08 | 45.67 | 50.36 | 44.02 |
50 | 50.10 | 47.63 | 52.49 | 52.48 |
51 | 52.33 | 50.03 | 54.80 | 59.68 |
52 | 54.81 | 52.53 | 57.06 | 59.05 |
53 | 57.29 | 55.18 | 59.43 | 58.41 |
54 | 59.94 | 57.63 | 61.98 | 64.08 |
55 | 62.58 | 60.35 | 64.63 | 72.62 |
56 | 65.32 | 63.29 | 67.43 | 72.33 |
57 | 68.16 | 66.10 | 69.93 | 74.08 |
58 | 71.02 | 69.13 | 72.75 | 77.39 |
59 | 73.84 | 72.13 | 75.52 | 77.36 |
60 | 76.66 | 74.84 | 78.15 | 77.13 |
61 | 79.34 | 77.73 | 80.98 | 77.51 |
62 | 82.01 | 80.53 | 83.43 | 74.30 |
63 | 84.48 | 83.13 | 85.73 | 79.08 |
64 | 86.82 | 85.57 | 87.95 | 82.86 |
65 | 88.94 | 87.79 | 90.02 | 83.52 |
Panel E: 8060 - Fracture of vertebral column | ||||
18 | 68.12 | 63.65 | 72.48 | 70.59 |
19 | 66.16 | 61.64 | 70.69 | 89.66 |
20 | 64.34 | 59.71 | 69.04 | 69.39 |
21 | 62.58 | 57.61 | 67.33 | 76.47 |
22 | 60.96 | 56.05 | 65.73 | 71.28 |
23 | 59.48 | 54.34 | 64.43 | 76.92 |
24 | 58.16 | 53.26 | 62.77 | 70.97 |
25 | 56.83 | 51.99 | 61.72 | 65.82 |
26 | 55.76 | 50.78 | 60.63 | 65.00 |
27 | 54.74 | 49.71 | 59.86 | 65.52 |
28 | 54.01 | 48.86 | 59.15 | 63.64 |
29 | 53.32 | 48.13 | 58.35 | 57.69 |
30 | 52.74 | 47.42 | 57.85 | 47.76 |
31 | 52.51 | 47.22 | 57.63 | 49.23 |
32 | 52.31 | 46.90 | 57.40 | 48.33 |
33 | 52.07 | 46.68 | 57.26 | 65.96 |
34 | 52.23 | 46.78 | 57.36 | 45.90 |
35 | 52.20 | 46.71 | 57.52 | 60.98 |
36 | 52.58 | 47.16 | 58.08 | 58.06 |
37 | 53.19 | 47.53 | 58.38 | 56.94 |
38 | 53.64 | 48.12 | 59.25 | 60.00 |
39 | 54.53 | 48.78 | 60.00 | 65.98 |
40 | 55.34 | 50.00 | 61.04 | 60.71 |
41 | 56.33 | 50.76 | 61.98 | 54.29 |
42 | 57.52 | 51.63 | 63.07 | 57.69 |
43 | 58.99 | 52.92 | 64.41 | 58.51 |
44 | 60.40 | 54.60 | 65.88 | 60.00 |
45 | 61.75 | 55.92 | 67.45 | 60.92 |
46 | 63.71 | 57.79 | 69.25 | 57.50 |
47 | 65.60 | 59.62 | 71.16 | 69.07 |
48 | 67.17 | 61.49 | 72.85 | 55.14 |
49 | 69.17 | 63.76 | 74.55 | 65.52 |
50 | 71.28 | 65.69 | 76.49 | 71.56 |
51 | 73.47 | 67.83 | 78.49 | 68.93 |
52 | 75.57 | 70.08 | 80.49 | 75.28 |
53 | 77.60 | 72.52 | 82.71 | 74.42 |
54 | 79.90 | 74.64 | 84.35 | 72.16 |
55 | 81.85 | 76.96 | 86.34 | 76.24 |
56 | 83.99 | 79.95 | 87.95 | 81.25 |
57 | 85.91 | 81.68 | 89.79 | 77.01 |
58 | 87.88 | 83.88 | 91.60 | 82.26 |
59 | 89.53 | 85.89 | 92.91 | 87.50 |
60 | 91.21 | 87.65 | 94.13 | 84.81 |
61 | 92.66 | 89.48 | 95.34 | 89.01 |
62 | 94.01 | 91.20 | 96.36 | 80.00 |
63 | 95.13 | 92.54 | 97.27 | 85.45 |
64 | 96.20 | 93.94 | 98.01 | 76.92 |
65 | 97.04 | 95.11 | 98.63 | 88.46 |
Panel F: 3450 - Epilepsy | ||||
18 | 23.25 | 21.56 | 24.99 | 17.39 |
19 | 21.53 | 19.99 | 23.23 | 11.41 |
20 | 19.93 | 18.40 | 21.51 | 12.91 |
21 | 18.58 | 17.10 | 20.05 | 11.61 |
22 | 17.33 | 15.91 | 18.73 | 12.78 |
23 | 16.25 | 14.98 | 17.63 | 12.04 |
24 | 15.30 | 14.06 | 16.58 | 11.19 |
25 | 14.43 | 13.25 | 15.67 | 12.23 |
26 | 13.70 | 12.50 | 14.88 | 9.46 |
27 | 13.05 | 11.93 | 14.18 | 8.79 |
28 | 12.48 | 11.39 | 13.58 | 9.68 |
29 | 12.04 | 10.95 | 13.10 | 10.03 |
30 | 11.66 | 10.60 | 12.74 | 11.35 |
31 | 11.35 | 10.30 | 12.46 | 10.31 |
32 | 11.12 | 10.04 | 12.14 | 9.63 |
33 | 10.96 | 9.93 | 12.06 | 9.87 |
34 | 10.86 | 9.82 | 11.96 | 12.55 |
35 | 10.81 | 9.73 | 11.99 | 9.17 |
36 | 10.92 | 9.83 | 11.96 | 12.60 |
37 | 10.96 | 9.90 | 12.10 | 10.52 |
38 | 11.15 | 10.05 | 12.31 | 10.98 |
39 | 11.39 | 10.29 | 12.60 | 12.13 |
40 | 11.69 | 10.54 | 12.93 | 11.99 |
41 | 12.10 | 10.92 | 13.42 | 12.36 |
42 | 12.60 | 11.39 | 13.86 | 13.70 |
43 | 13.20 | 11.86 | 14.57 | 15.36 |
44 | 13.85 | 12.44 | 15.18 | 13.29 |
45 | 14.58 | 13.14 | 16.04 | 10.04 |
46 | 15.45 | 13.92 | 17.01 | 11.92 |
47 | 16.44 | 14.86 | 18.15 | 10.91 |
48 | 17.58 | 15.95 | 19.27 | 12.04 |
49 | 18.92 | 17.13 | 20.70 | 12.32 |
50 | 20.24 | 18.30 | 22.27 | 16.73 |
51 | 21.84 | 19.87 | 23.94 | 18.75 |
52 | 23.65 | 21.60 | 25.83 | 18.33 |
53 | 25.59 | 23.45 | 27.79 | 17.80 |
54 | 27.78 | 25.50 | 30.15 | 25.30 |
55 | 30.19 | 27.78 | 32.92 | 29.32 |
56 | 32.75 | 30.21 | 35.33 | 36.96 |
57 | 35.54 | 32.86 | 38.25 | 34.20 |
58 | 38.61 | 35.64 | 41.43 | 34.46 |
59 | 41.77 | 38.91 | 44.74 | 38.07 |
60 | 45.22 | 42.00 | 48.36 | 38.90 |
61 | 48.86 | 45.52 | 52.15 | 43.05 |
62 | 52.69 | 49.11 | 55.95 | 42.32 |
63 | 56.40 | 52.99 | 59.78 | 45.13 |
64 | 60.50 | 56.97 | 64.04 | 54.31 |
65 | 64.41 | 60.57 | 67.95 | 55.56 |
Mental Disorders
Among the mental disorders, several impairments show high positive correlation with an initial allowance, including intellectual disability (0.43), schizophrenic/other psychotic disorders (0.29), autistic disorders (0.29), and organic mental impairments (0.15). On the other hand, the most common diagnosis overall (affective/mood disorders) has an estimated mean correlation with an initial allowance of −0.19, reflecting a lower-than-average proportion of allowances at 24.4%.12 Panels B and C of Chart 1 provide a comparison of the estimated age-specific conditional probabilities of an initial allowance for organic mental impairments and affective/mood disorders, respectively.
Circulatory Impairments
Several circulatory diagnoses share positive correlations with an initial allowance and an allowance rate in excess of 60%, including late effects of cerebrovascular disease (0.24), chronic pulmonary heart disease (0.17), heart failure (0.16), and peripheral vascular disease (0.15). Panel D of Chart 1 shows conditional probability estimates for heart failure. One exception to the relatively high allowance rates in the circulatory diagnostic group is that for essential hypertension, with a mean correlation of −0.23 and a 27% allowance rate. Essential hypertension constitutes almost 42% of all circulatory diagnoses and is the sixth most common of all impairments.
Injuries
In the injuries category, a number of impairments have very low initial allowance rates (less than 25%), including fractures of the upper limb, dislocations, open wounds of the upper limb, and sprains/strains of all types. By contrast, two impairments have initial allowance rates over 50%: fractures of the vertebral column with spinal cord lesions and intracranial (traumatic brain) injuries, which have estimated posterior mean correlations of 0.29 and 0.17, respectively. Age-specific predicted probabilities of an initial allowance for a diagnosis involving a fracture of the vertebral column appear in Panel E of Chart 1.
Musculoskeletal Impairments
Disorders of the back is the second most common impairment overall, representing 14.6% of all primary and secondary diagnoses combined (Table 1). Along with two other musculoskeletal-system diagnoses (disorders of the muscle/ligament/fascia and other/unspecified arthropathies), these disorders exhibit relatively low initial allowance rates (below 30%), resulting in negative estimated mean correlations with initial allowance in Table 3.
Infectious/Parasitic Diseases
Two impairments—symptomatic HIV and asymptomatic HIV—account for more than 80% of the infectious/parasitic diagnoses and have statistically significant estimated correlations with an initial allowance. Only 9% of claimants diagnosed with an asymptomatic HIV infection receive an initial allowance, yielding a mean correlation of −0.17. On the other hand, 50% of symptomatic HIV diagnoses result in an allowance and a posterior mean correlation estimate of 0.19.
Nervous System and Sense Organ Diseases
Ten of the impairments in this category are positively correlated with an initial allowance: anterior horn cell disease13 (with a 97% initial allowance rate), other diseases of the spinal cord, muscular dystrophies, late effects of injuries to the nervous system, cerebral palsy, Parkinson's disease, other cerebral degenerations, multiple sclerosis, blindness/low vision, and other retina disorders. By contrast, epilepsy and migraine have very low allowance rates (about 15%) and negative mean correlations (−0.16 and −0.13, respectively). Epilepsy is the most commonly diagnosed impairment in this diagnostic group and the 17th most frequent overall. Age-specific probability estimates of an initial allowance associated with epilepsy appear in Panel F of Chart 1.
Other Disorders
Additional impairments with low initial allowance rates are hernias and other disorders of the gastrointestinal system (digestive system diseases), diabetes and all disorders of the thyroid (endocrine/nutritional/metabolic diseases), and other disorders of the urinary tract (genitourinary system diseases). Conversely, chronic renal failure, with an 84% allowance rate, has an estimated 0.42 mean correlation with an initial allowance.
Variables Correlated with Sex
Table 4 presents correlation estimates associated with sex, where the binary variable takes a value of 1 if male. Men represent 53.5% of all 2009 qualified claimants (not shown). On average, female filers are somewhat younger (a median age difference of 1 year). Male applicants have a 40.6% initial allowance rate, compared with 34.7% for female claimants. Chart 2 shows the proportion of applicants who are men, by age. Although men account for at least 50% of applicants at every age, the lowest male shares of applicants occur between the ages of 28 through 38. From age 39 upward, the proportion increases steadily, so that by age 61 the male share of claimants is closer to 58%. Some of this variation can be attributed to the differences by sex in the age of onset associated with a number of impairments.
Diagnostic group, impairment code, and diagnosis | Men as a percentage of applicants | Correlation (posterior mean) | t-statistic | Numerical standard error |
---|---|---|---|---|
Neoplasms (malignant) | ||||
1850 - Prostate cancer | 100.00 | 0.5342 | 16.04 | 0.005111 |
1500 - Esophageal cancer | 86.03 | 0.2525 | 10.42 | 0.002312 |
1950 - Soft tissue tumor of the head and neck | 76.09 | 0.1796 | 10.51 | 0.000931 |
1890 - Kidney cancer | 70.91 | 0.1389 | 6.85 | 0.001172 |
1550 - Liver cancer | 66.53 | 0.1084 | 6.70 | 0.000913 |
1740 - Breast cancer | 1.06 | -0.6107 | -50.62 | 0.001068 |
1830 - Ovarian cancer | 0.00 | -0.4750 | -19.07 | 0.002969 |
1790 - Uterine cancer | 0.00 | -0.4490 | -15.56 | 0.003630 |
Mental disorders | ||||
2990 - Autistic disorders | 83.13 | 0.2693 | 9.13 | 0.002672 |
2950 - Schizophrenic and other psychotic disorders | 65.92 | 0.1504 | 16.53 | 0.000278 |
3030 - Substance addiction (alcohol) | 69.79 | 0.1392 | 11.88 | 0.000345 |
3152 - Learning disorder | 67.25 | 0.1281 | 6.95 | 0.001082 |
3140 - Attention deficit/hyperactivity disorder | 65.73 | 0.1279 | 7.77 | 0.000857 |
3180 - Intellectual disability | 63.88 | 0.1125 | 9.97 | 0.000459 |
2940 - Organic mental disorders | 62.31 | 0.1072 | 15.48 | 0.000185 |
2960 - Affective and mood disorders | 43.63 | -0.1951 | -48.26 | 0.000102 |
3000 - Anxiety and obsessive-compulsive disorders | 43.70 | -0.1224 | -21.82 | 0.000117 |
Circulatory system diseases | ||||
4140 - Chronic ischemic heart disease | 74.58 | 0.2207 | 27.19 | 0.000227 |
4100 - Acute myocardial infarction | 75.01 | 0.1802 | 10.04 | 0.001029 |
4280 - Heart failure | 69.33 | 0.1489 | 14.56 | 0.000278 |
4250 - Cardiomyopathy | 71.12 | 0.1407 | 11.43 | 0.000578 |
4430 - Peripheral vascular disease | 69.83 | 0.1137 | 8.80 | 0.000508 |
3950 - Diseases of aortic valve | 68.45 | 0.1097 | 5.36 | 0.000943 |
Injuries | ||||
8060 - Fracture of vertebral column | 75.96 | 0.1749 | 8.51 | 0.001149 |
9050 - Late effects of musculoskeletal and connective tissue injuries (amputation) | 72.15 | 0.1514 | 13.08 | 0.000385 |
8940 - Open soft tissue wound of lower limb | 70.27 | 0.1372 | 6.15 | 0.001752 |
8540 - Intracranial (traumatic brain) injury | 68.35 | 0.1312 | 7.41 | 0.000896 |
8840 - Open soft tissue wound of upper limb | 71.15 | 0.1193 | 5.08 | 0.001589 |
8270 - Fractures of lower limb | 64.72 | 0.1108 | 12.49 | 0.000216 |
8290 - Other fractures of bones | 67.11 | 0.1084 | 7.88 | 0.000542 |
Musculoskeletal system and connective tissue diseases | ||||
7100 - Diffuse diseases of connective tissue | 15.09 | -0.3365 | -22.21 | 0.000798 |
7140 - Rheumatoid arthritis | 31.22 | -0.2087 | -20.04 | 0.000350 |
7370 - Curvature of spine | 38.32 | -0.1096 | -6.22 | 0.000968 |
Infectious and parasitic diseases | ||||
0430 - Symptomatic HIV | 75.68 | 0.2021 | 15.27 | 0.000851 |
0440 - Asymptomatic HIV | 69.85 | 0.1199 | 7.73 | 0.000615 |
1350 - Sarcoidosis | 40.85 | -0.1034 | -4.51 | 0.001882 |
Endocrine, nutritional, and metabolic diseases | ||||
2740 - Gout | 87.87 | 0.2925 | 13.28 | 0.001498 |
2460 - All disorders of thyroid | 21.78 | -0.2274 | -12.89 | 0.001055 |
2780 - Obesity | 43.06 | -0.1150 | -17.10 | 0.000207 |
Nervous system and sense organ diseases | ||||
3320 - Parkinson's disease | 69.57 | 0.1235 | 6.34 | 0.001258 |
3690 - Blindness and low vision | 62.72 | 0.1004 | 9.09 | 0.000400 |
3400 - Multiple sclerosis | 28.94 | -0.2149 | -17.81 | 0.000418 |
3460 - Migraine | 30.40 | -0.2025 | -18.07 | 0.000418 |
3540 - Carpal tunnel syndrome | 33.72 | -0.1529 | -12.55 | 0.000537 |
Respiratory system diseases | ||||
7800 - Sleep-related breathing disorders | 67.04 | 0.1030 | 6.48 | 0.000663 |
4930 - Asthma | 36.64 | -0.1585 | -17.91 | 0.000257 |
Digestive system diseases | ||||
5530 - Hernias | 70.24 | 0.1536 | 8.03 | 0.001171 |
5710 - Chronic liver disease and cirrhosis | 67.32 | 0.1369 | 14.84 | 0.000278 |
SOURCE: Author's calculations using DRF 100% extract (for descriptive statistics) and MVP estimation model fitted to a DRF 10% random sample (for correlation estimates). | ||||
NOTES: Correlation estimates are selected for inclusion on the basis of having absolute values for the posterior mean of at least 0.10 and, generally, a t-statistic greater than 2.00.
Data are for applicants who cleared step 1 of the disability determination process.
Diagnoses are arranged by estimated correlation value. Negative correlations are listed by absolute value.
Diagnoses identified as "other" may be defined in the context of impairments not shown in this table.
|
Men as a percentage of initial disability-benefit claimants, by age, 2009
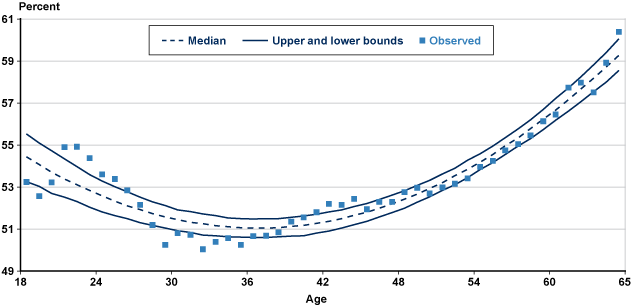
Age | Median | Lower bound | Upper bound | Observed |
---|---|---|---|---|
18 | 54.43 | 53.26 | 55.52 | 53.24 |
19 | 54.07 | 53.02 | 55.09 | 52.56 |
20 | 53.69 | 52.69 | 54.72 | 53.22 |
21 | 53.38 | 52.51 | 54.34 | 54.89 |
22 | 53.10 | 52.30 | 53.96 | 54.91 |
23 | 52.84 | 52.03 | 53.59 | 54.36 |
24 | 52.57 | 51.80 | 53.28 | 53.60 |
25 | 52.32 | 51.63 | 53.00 | 53.38 |
26 | 52.09 | 51.48 | 52.77 | 52.83 |
27 | 51.91 | 51.30 | 52.51 | 52.15 |
28 | 51.73 | 51.17 | 52.29 | 51.18 |
29 | 51.58 | 51.04 | 52.12 | 50.25 |
30 | 51.43 | 50.92 | 51.91 | 50.80 |
31 | 51.32 | 50.84 | 51.82 | 50.71 |
32 | 51.24 | 50.71 | 51.72 | 50.04 |
33 | 51.15 | 50.67 | 51.63 | 50.39 |
34 | 51.10 | 50.63 | 51.52 | 50.56 |
35 | 51.05 | 50.61 | 51.48 | 50.24 |
36 | 51.04 | 50.59 | 51.47 | 50.65 |
37 | 51.05 | 50.59 | 51.48 | 50.66 |
38 | 51.06 | 50.62 | 51.49 | 50.84 |
39 | 51.12 | 50.65 | 51.55 | 51.35 |
40 | 51.16 | 50.68 | 51.61 | 51.56 |
41 | 51.26 | 50.80 | 51.70 | 51.79 |
42 | 51.36 | 50.90 | 51.81 | 52.19 |
43 | 51.49 | 51.04 | 51.90 | 52.14 |
44 | 51.65 | 51.20 | 52.06 | 52.44 |
45 | 51.80 | 51.37 | 52.21 | 51.93 |
46 | 51.99 | 51.56 | 52.40 | 52.29 |
47 | 52.19 | 51.78 | 52.61 | 52.29 |
48 | 52.41 | 51.99 | 52.82 | 52.76 |
49 | 52.66 | 52.27 | 53.08 | 52.97 |
50 | 52.93 | 52.53 | 53.31 | 52.69 |
51 | 53.21 | 52.84 | 53.61 | 52.98 |
52 | 53.53 | 53.10 | 53.89 | 53.15 |
53 | 53.84 | 53.44 | 54.28 | 53.41 |
54 | 54.18 | 53.83 | 54.58 | 53.95 |
55 | 54.55 | 54.15 | 54.95 | 54.24 |
56 | 54.95 | 54.54 | 55.34 | 54.74 |
57 | 55.33 | 54.94 | 55.77 | 55.03 |
58 | 55.77 | 55.29 | 56.19 | 55.45 |
59 | 56.22 | 55.73 | 56.68 | 56.13 |
60 | 56.66 | 56.17 | 57.22 | 56.45 |
61 | 57.16 | 56.61 | 57.71 | 57.72 |
62 | 57.66 | 57.06 | 58.24 | 57.97 |
63 | 58.18 | 57.54 | 58.82 | 57.51 |
64 | 58.71 | 57.99 | 59.40 | 58.92 |
65 | 59.27 | 58.54 | 60.05 | 60.38 |
Malignant Neoplasms
Three diagnoses in the neoplastic diagnostic group are sex-specific. Obviously, the men's share of applicants diagnosed with cancer of the prostate is 100% and for cancers of the ovaries and uterus it is 0%. About 1% of breast cancer diagnoses involve men, which seems to fit with the pattern in the general population (women are about 100 times more likely than men to have a breast cancer diagnosis in their lifetime) (American Cancer Society 2018). The other listed types of cancer exhibit much higher incidence among men. For instance, Table 4 shows that 86% of the diagnoses involving esophageal cancer correspond to male claimants, yielding a positive posterior mean correlation of 0.25. Likewise, men account for more than 70% of kidney cancers and of soft tissue tumors of the head and neck, and for 67% of liver cancer cases. Among all neoplasms combined, however, men account for 49.6% of diagnosed claimants. This is due to the influence of breast cancer, which is the most common neoplastic impairment after lung cancer and is obviously overwhelmingly female.
Mental Disorders
Although men constitute 53.5% of all claimants, they account for only about 44% of applicants with affective/mood disorder and anxiety disorder diagnoses. Thus, women are more likely than men to be diagnosed with these two impairments, which combine to make up 22.8% of all primary and secondary diagnoses (overall, they are the first and fourth most common disorders, respectively). However, men are more likely than women to be diagnosed with each of the other mental impairments listed in Table 4. For instance, 83% of applicants diagnosed with autism are men. Likewise, men account for more than 60% of applicants diagnosed with schizophrenia, substance addiction (alcohol), attention deficit disorder, learning disorder, intellectual disability, and organic mental impairments. Overall, men account for 48.6% of all mental diagnoses.
Mental impairments with a higher incidence among men tend to have relatively high allowance rates (except for addiction), while female-dominated mental disorders experience below-average allowance rates. These differences in the sex composition of mental disorders are bound to have important distributional implications for any policy targeting the disability determination process for mental impairments. Specifically, making disability determination for mental disorders more stringent is likely to result in a disproportionately larger share of female claimants being denied or delayed eventual entry into the disability rolls.
Panel A of Chart 3 displays the probability of having an affective/mood disorder diagnosis as a function of age, by sex. For a 30-year-old male claimant, the probability of having an affective/mood disorder as a primary or secondary impairment is roughly 38%. For a woman aged 30, the probability is about 52%. It is worth noting that the peak ages of onset for affective/mood disorders (early and mid-thirties) roughly correspond with the age range at which the men's share of applicants overall is lowest (Chart 2). In other words, the age of onset and the size of the affective/mood and anxiety disorder categories are driving factors in the aggregate variation in sex composition by age. For comparative purposes, Panel B of Chart 3 presents the incidence probabilities associated with a second mental disorder (schizophrenia).
Estimated incidence probabilities of selected diagnoses for initial disability-benefit claimants, by age and sex, 2009
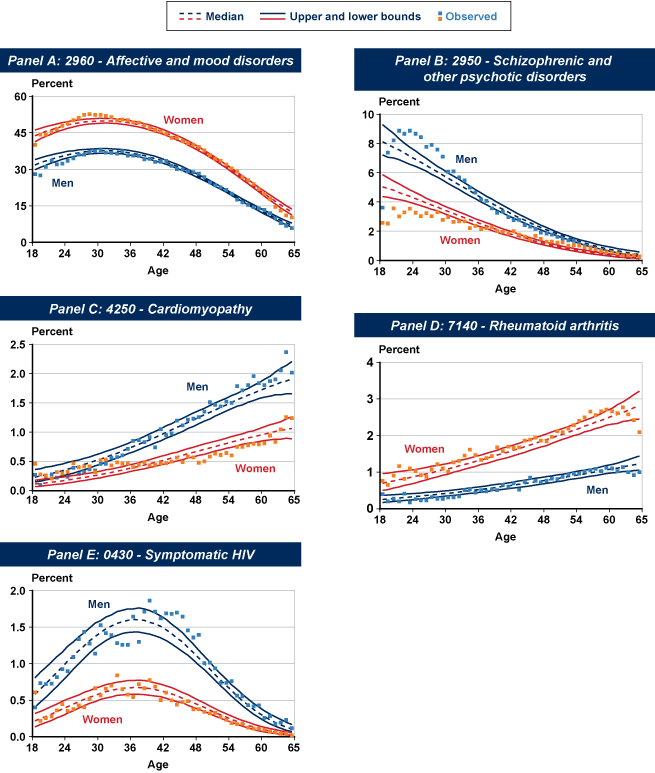
Age | Men | Women | ||||||
---|---|---|---|---|---|---|---|---|
Median | Lower bound | Upper bound | Observed | Median | Lower bound | Upper bound | Observed | |
Panel A: 2960 - Affective and mood disorders | ||||||||
18 | 31.83 | 29.85 | 34.02 | 28.07 | 43.76 | 41.36 | 46.09 | 40.03 |
19 | 32.70 | 30.80 | 34.73 | 27.54 | 44.64 | 42.50 | 46.85 | 44.23 |
20 | 33.51 | 31.65 | 35.37 | 30.98 | 45.59 | 43.55 | 47.47 | 44.27 |
21 | 34.19 | 32.55 | 35.97 | 33.53 | 46.33 | 44.47 | 48.12 | 45.42 |
22 | 34.86 | 33.35 | 36.46 | 32.02 | 47.02 | 45.44 | 48.64 | 46.57 |
23 | 35.44 | 34.11 | 36.91 | 32.32 | 47.65 | 46.18 | 49.16 | 47.84 |
24 | 35.97 | 34.74 | 37.40 | 33.49 | 48.19 | 46.84 | 49.60 | 48.84 |
25 | 36.41 | 35.22 | 37.61 | 34.49 | 48.70 | 47.43 | 50.00 | 50.18 |
26 | 36.77 | 35.66 | 37.98 | 36.05 | 49.06 | 47.93 | 50.32 | 51.05 |
27 | 37.11 | 36.06 | 38.19 | 36.13 | 49.38 | 48.29 | 50.56 | 52.36 |
28 | 37.32 | 36.32 | 38.29 | 37.17 | 49.63 | 48.59 | 50.71 | 52.63 |
29 | 37.49 | 36.54 | 38.45 | 37.42 | 49.79 | 48.81 | 50.85 | 52.40 |
30 | 37.57 | 36.68 | 38.52 | 37.92 | 49.84 | 48.96 | 50.90 | 52.35 |
31 | 37.56 | 36.67 | 38.50 | 36.93 | 49.89 | 49.00 | 50.89 | 51.96 |
32 | 37.52 | 36.63 | 38.45 | 36.77 | 49.83 | 48.87 | 50.79 | 51.41 |
33 | 37.40 | 36.45 | 38.32 | 36.48 | 49.69 | 48.73 | 50.65 | 50.20 |
34 | 37.18 | 36.31 | 38.12 | 37.51 | 49.47 | 48.49 | 50.48 | 50.45 |
35 | 36.89 | 35.97 | 37.81 | 35.70 | 49.15 | 48.19 | 50.19 | 50.18 |
36 | 36.53 | 35.65 | 37.43 | 35.68 | 48.81 | 47.83 | 49.76 | 49.29 |
37 | 36.11 | 35.15 | 37.02 | 35.49 | 48.32 | 47.35 | 49.35 | 49.17 |
38 | 35.61 | 34.68 | 36.53 | 34.09 | 47.77 | 46.82 | 48.73 | 48.02 |
39 | 35.01 | 34.18 | 35.93 | 34.30 | 47.18 | 46.19 | 48.24 | 46.95 |
40 | 34.40 | 33.50 | 35.37 | 33.03 | 46.48 | 45.50 | 47.53 | 46.18 |
41 | 33.67 | 32.79 | 34.55 | 32.97 | 45.73 | 44.72 | 46.74 | 45.60 |
42 | 32.93 | 32.05 | 33.78 | 33.45 | 44.89 | 43.92 | 45.91 | 44.62 |
43 | 32.07 | 31.25 | 32.96 | 31.43 | 43.99 | 43.09 | 44.87 | 42.90 |
44 | 31.21 | 30.41 | 32.05 | 30.11 | 43.01 | 42.06 | 43.94 | 42.99 |
45 | 30.24 | 29.46 | 31.11 | 30.01 | 41.98 | 41.10 | 42.82 | 41.43 |
46 | 29.27 | 28.52 | 30.04 | 29.17 | 40.83 | 39.96 | 41.66 | 40.78 |
47 | 28.21 | 27.52 | 28.94 | 28.96 | 39.62 | 38.79 | 40.48 | 40.06 |
48 | 27.10 | 26.44 | 27.74 | 28.22 | 38.37 | 37.61 | 39.19 | 39.20 |
49 | 25.94 | 25.34 | 26.57 | 26.80 | 37.04 | 36.35 | 37.79 | 37.78 |
50 | 24.79 | 24.19 | 25.39 | 24.58 | 35.68 | 34.97 | 36.40 | 35.76 |
51 | 23.58 | 23.02 | 24.21 | 23.44 | 34.23 | 33.57 | 34.96 | 34.41 |
52 | 22.33 | 21.81 | 22.93 | 22.61 | 32.75 | 32.10 | 33.44 | 33.46 |
53 | 21.10 | 20.58 | 21.65 | 21.54 | 31.23 | 30.54 | 31.92 | 31.93 |
54 | 19.84 | 19.28 | 20.39 | 20.13 | 29.68 | 28.95 | 30.41 | 30.41 |
55 | 18.56 | 18.05 | 19.17 | 17.56 | 28.09 | 27.40 | 28.85 | 29.08 |
56 | 17.29 | 16.74 | 17.90 | 16.50 | 26.47 | 25.75 | 27.28 | 26.78 |
57 | 16.04 | 15.42 | 16.67 | 15.36 | 24.87 | 24.02 | 25.70 | 25.58 |
58 | 14.80 | 14.16 | 15.50 | 14.35 | 23.27 | 22.37 | 24.15 | 23.87 |
59 | 13.57 | 12.89 | 14.32 | 14.00 | 21.60 | 20.70 | 22.52 | 21.93 |
60 | 12.40 | 11.65 | 13.14 | 13.26 | 19.99 | 19.00 | 21.03 | 19.88 |
61 | 11.24 | 10.49 | 12.01 | 12.01 | 18.42 | 17.32 | 19.52 | 17.85 |
62 | 10.13 | 9.36 | 10.95 | 9.91 | 16.88 | 15.76 | 18.05 | 14.51 |
63 | 9.09 | 8.26 | 9.91 | 7.98 | 15.37 | 14.18 | 16.57 | 12.99 |
64 | 8.10 | 7.25 | 8.94 | 6.89 | 13.89 | 12.76 | 15.09 | 11.16 |
65 | 7.16 | 6.33 | 8.00 | 5.83 | 12.48 | 11.25 | 13.76 | 10.25 |
Panel B: 2950 - Schizophrenic and other psychotic disorders | ||||||||
18 | 8.14 | 7.22 | 9.30 | 3.61 | 5.05 | 4.38 | 5.88 | 2.59 |
19 | 7.95 | 7.08 | 9.00 | 7.39 | 4.93 | 4.33 | 5.66 | 2.55 |
20 | 7.74 | 7.02 | 8.70 | 8.22 | 4.81 | 4.25 | 5.44 | 3.59 |
21 | 7.59 | 6.90 | 8.34 | 8.88 | 4.66 | 4.16 | 5.25 | 3.03 |
22 | 7.36 | 6.76 | 8.04 | 8.68 | 4.53 | 4.10 | 5.04 | 3.30 |
23 | 7.15 | 6.58 | 7.77 | 8.88 | 4.39 | 3.98 | 4.85 | 3.55 |
24 | 6.94 | 6.45 | 7.51 | 8.69 | 4.23 | 3.87 | 4.66 | 3.28 |
25 | 6.73 | 6.29 | 7.23 | 8.46 | 4.10 | 3.76 | 4.46 | 3.06 |
26 | 6.51 | 6.11 | 7.00 | 7.78 | 3.94 | 3.65 | 4.27 | 3.25 |
27 | 6.30 | 5.91 | 6.73 | 7.91 | 3.81 | 3.51 | 4.11 | 3.05 |
28 | 6.08 | 5.70 | 6.46 | 7.61 | 3.66 | 3.41 | 3.96 | 3.32 |
29 | 5.86 | 5.49 | 6.23 | 7.12 | 3.51 | 3.25 | 3.77 | 2.80 |
30 | 5.64 | 5.27 | 5.99 | 6.08 | 3.36 | 3.12 | 3.63 | 2.98 |
31 | 5.42 | 5.06 | 5.78 | 6.11 | 3.22 | 2.99 | 3.48 | 2.67 |
32 | 5.19 | 4.86 | 5.54 | 5.70 | 3.08 | 2.83 | 3.32 | 2.67 |
33 | 4.97 | 4.64 | 5.32 | 5.47 | 2.93 | 2.69 | 3.19 | 2.67 |
34 | 4.76 | 4.42 | 5.09 | 4.99 | 2.78 | 2.57 | 3.02 | 2.22 |
35 | 4.53 | 4.23 | 4.87 | 4.66 | 2.64 | 2.43 | 2.86 | 2.38 |
36 | 4.32 | 4.01 | 4.63 | 4.19 | 2.51 | 2.31 | 2.73 | 2.15 |
37 | 4.11 | 3.83 | 4.41 | 4.07 | 2.37 | 2.19 | 2.60 | 2.22 |
38 | 3.91 | 3.63 | 4.21 | 3.59 | 2.25 | 2.06 | 2.46 | 2.30 |
39 | 3.71 | 3.44 | 4.00 | 3.27 | 2.12 | 1.93 | 2.32 | 2.01 |
40 | 3.51 | 3.24 | 3.78 | 3.35 | 1.99 | 1.81 | 2.18 | 1.96 |
41 | 3.33 | 3.07 | 3.57 | 2.99 | 1.88 | 1.71 | 2.05 | 1.85 |
42 | 3.12 | 2.90 | 3.36 | 2.77 | 1.75 | 1.60 | 1.94 | 2.00 |
43 | 2.94 | 2.72 | 3.17 | 2.79 | 1.64 | 1.49 | 1.81 | 1.77 |
44 | 2.76 | 2.57 | 2.97 | 2.42 | 1.54 | 1.40 | 1.68 | 1.64 |
45 | 2.59 | 2.40 | 2.80 | 2.34 | 1.43 | 1.30 | 1.57 | 1.45 |
46 | 2.42 | 2.24 | 2.60 | 2.16 | 1.33 | 1.21 | 1.46 | 1.68 |
47 | 2.26 | 2.10 | 2.43 | 1.91 | 1.23 | 1.11 | 1.35 | 1.49 |
48 | 2.11 | 1.95 | 2.25 | 1.85 | 1.14 | 1.03 | 1.25 | 1.31 |
49 | 1.96 | 1.82 | 2.09 | 1.79 | 1.06 | 0.95 | 1.16 | 1.30 |
50 | 1.82 | 1.68 | 1.95 | 1.61 | 0.97 | 0.86 | 1.08 | 1.22 |
51 | 1.68 | 1.54 | 1.81 | 1.49 | 0.88 | 0.80 | 0.99 | 1.25 |
52 | 1.55 | 1.43 | 1.69 | 1.39 | 0.81 | 0.72 | 0.91 | 1.02 |
53 | 1.43 | 1.30 | 1.55 | 1.34 | 0.74 | 0.66 | 0.84 | 1.07 |
54 | 1.31 | 1.18 | 1.43 | 1.13 | 0.67 | 0.59 | 0.77 | 1.03 |
55 | 1.20 | 1.06 | 1.34 | 1.03 | 0.62 | 0.53 | 0.72 | 0.90 |
56 | 1.10 | 0.96 | 1.24 | 0.92 | 0.56 | 0.47 | 0.65 | 0.85 |
57 | 1.00 | 0.87 | 1.14 | 0.74 | 0.50 | 0.43 | 0.60 | 0.78 |
58 | 0.91 | 0.77 | 1.05 | 0.70 | 0.45 | 0.37 | 0.55 | 0.67 |
59 | 0.82 | 0.69 | 0.97 | 0.54 | 0.41 | 0.33 | 0.50 | 0.59 |
60 | 0.75 | 0.61 | 0.90 | 0.50 | 0.37 | 0.29 | 0.46 | 0.57 |
61 | 0.67 | 0.54 | 0.83 | 0.43 | 0.33 | 0.25 | 0.42 | 0.49 |
62 | 0.60 | 0.47 | 0.76 | 0.35 | 0.29 | 0.22 | 0.38 | 0.36 |
63 | 0.54 | 0.41 | 0.70 | 0.24 | 0.26 | 0.18 | 0.36 | 0.39 |
64 | 0.48 | 0.36 | 0.64 | 0.27 | 0.23 | 0.16 | 0.32 | 0.39 |
65 | 0.43 | 0.31 | 0.58 | 0.26 | 0.20 | 0.13 | 0.29 | 0.28 |
Panel C: 4250 - Cardiomyopathy | ||||||||
18 | 0.23 | 0.15 | 0.36 | 0.27 | 0.11 | 0.07 | 0.18 | 0.46 |
19 | 0.25 | 0.16 | 0.37 | 0.10 | 0.12 | 0.07 | 0.19 | 0.27 |
20 | 0.27 | 0.18 | 0.40 | 0.22 | 0.13 | 0.09 | 0.20 | 0.25 |
21 | 0.29 | 0.20 | 0.41 | 0.27 | 0.14 | 0.09 | 0.21 | 0.20 |
22 | 0.31 | 0.22 | 0.43 | 0.22 | 0.15 | 0.11 | 0.22 | 0.32 |
23 | 0.34 | 0.25 | 0.45 | 0.30 | 0.17 | 0.12 | 0.23 | 0.29 |
24 | 0.36 | 0.27 | 0.48 | 0.23 | 0.18 | 0.13 | 0.25 | 0.32 |
25 | 0.39 | 0.30 | 0.50 | 0.39 | 0.19 | 0.14 | 0.26 | 0.46 |
26 | 0.42 | 0.32 | 0.52 | 0.29 | 0.21 | 0.16 | 0.27 | 0.39 |
27 | 0.45 | 0.36 | 0.55 | 0.32 | 0.22 | 0.17 | 0.29 | 0.41 |
28 | 0.48 | 0.38 | 0.58 | 0.36 | 0.24 | 0.19 | 0.30 | 0.41 |
29 | 0.51 | 0.42 | 0.61 | 0.35 | 0.26 | 0.20 | 0.31 | 0.31 |
30 | 0.54 | 0.45 | 0.63 | 0.46 | 0.28 | 0.22 | 0.33 | 0.52 |
31 | 0.57 | 0.49 | 0.67 | 0.46 | 0.29 | 0.24 | 0.35 | 0.53 |
32 | 0.61 | 0.52 | 0.70 | 0.56 | 0.31 | 0.25 | 0.37 | 0.43 |
33 | 0.64 | 0.55 | 0.73 | 0.57 | 0.33 | 0.27 | 0.39 | 0.48 |
34 | 0.67 | 0.59 | 0.77 | 0.60 | 0.35 | 0.29 | 0.41 | 0.46 |
35 | 0.71 | 0.63 | 0.80 | 0.71 | 0.37 | 0.31 | 0.43 | 0.46 |
36 | 0.75 | 0.66 | 0.85 | 0.85 | 0.39 | 0.33 | 0.45 | 0.40 |
37 | 0.79 | 0.70 | 0.88 | 0.86 | 0.41 | 0.36 | 0.48 | 0.44 |
38 | 0.83 | 0.74 | 0.93 | 0.82 | 0.44 | 0.37 | 0.50 | 0.49 |
39 | 0.87 | 0.77 | 0.97 | 0.91 | 0.46 | 0.40 | 0.53 | 0.43 |
40 | 0.91 | 0.81 | 1.01 | 0.74 | 0.48 | 0.41 | 0.56 | 0.44 |
41 | 0.95 | 0.85 | 1.06 | 1.05 | 0.51 | 0.44 | 0.59 | 0.47 |
42 | 0.99 | 0.89 | 1.10 | 0.94 | 0.53 | 0.46 | 0.61 | 0.46 |
43 | 1.04 | 0.93 | 1.14 | 0.98 | 0.56 | 0.48 | 0.64 | 0.49 |
44 | 1.08 | 0.97 | 1.19 | 1.19 | 0.58 | 0.50 | 0.65 | 0.56 |
45 | 1.12 | 1.01 | 1.23 | 1.12 | 0.60 | 0.53 | 0.69 | 0.49 |
46 | 1.17 | 1.06 | 1.28 | 1.21 | 0.63 | 0.55 | 0.71 | 0.58 |
47 | 1.21 | 1.09 | 1.33 | 1.23 | 0.65 | 0.57 | 0.74 | 0.56 |
48 | 1.25 | 1.14 | 1.37 | 1.26 | 0.68 | 0.60 | 0.77 | 0.47 |
49 | 1.29 | 1.19 | 1.40 | 1.35 | 0.70 | 0.62 | 0.79 | 0.50 |
50 | 1.34 | 1.22 | 1.45 | 1.51 | 0.73 | 0.65 | 0.82 | 0.58 |
51 | 1.38 | 1.27 | 1.50 | 1.47 | 0.75 | 0.67 | 0.84 | 0.63 |
52 | 1.42 | 1.31 | 1.54 | 1.44 | 0.78 | 0.69 | 0.87 | 0.61 |
53 | 1.47 | 1.36 | 1.58 | 1.52 | 0.80 | 0.72 | 0.89 | 0.64 |
54 | 1.51 | 1.40 | 1.62 | 1.50 | 0.83 | 0.74 | 0.92 | 0.60 |
55 | 1.55 | 1.44 | 1.66 | 1.79 | 0.85 | 0.76 | 0.94 | 0.75 |
56 | 1.59 | 1.47 | 1.70 | 1.71 | 0.88 | 0.79 | 0.97 | 0.75 |
57 | 1.63 | 1.51 | 1.75 | 1.81 | 0.90 | 0.81 | 1.00 | 0.77 |
58 | 1.67 | 1.54 | 1.79 | 1.96 | 0.92 | 0.82 | 1.02 | 0.80 |
59 | 1.71 | 1.57 | 1.84 | 1.84 | 0.94 | 0.84 | 1.05 | 0.79 |
60 | 1.74 | 1.60 | 1.90 | 1.80 | 0.96 | 0.86 | 1.09 | 0.81 |
61 | 1.78 | 1.61 | 1.95 | 1.87 | 0.99 | 0.87 | 1.12 | 0.94 |
62 | 1.81 | 1.63 | 2.03 | 1.90 | 1.01 | 0.88 | 1.15 | 0.83 |
63 | 1.85 | 1.63 | 2.09 | 2.06 | 1.03 | 0.88 | 1.18 | 1.05 |
64 | 1.87 | 1.65 | 2.13 | 2.37 | 1.05 | 0.89 | 1.22 | 1.26 |
65 | 1.90 | 1.65 | 2.21 | 2.01 | 1.06 | 0.88 | 1.26 | 1.24 |
Panel D: 7140 - Rheumatoid arthritis | ||||||||
18 | 0.26 | 0.17 | 0.37 | 0.40 | 0.70 | 0.50 | 0.96 | 0.76 |
19 | 0.27 | 0.18 | 0.37 | 0.20 | 0.73 | 0.52 | 0.97 | 0.65 |
20 | 0.28 | 0.20 | 0.39 | 0.27 | 0.76 | 0.56 | 1.00 | 0.90 |
21 | 0.29 | 0.21 | 0.39 | 0.20 | 0.79 | 0.59 | 1.01 | 1.16 |
22 | 0.31 | 0.23 | 0.41 | 0.41 | 0.82 | 0.64 | 1.04 | 0.81 |
23 | 0.32 | 0.24 | 0.41 | 0.18 | 0.85 | 0.67 | 1.06 | 1.10 |
24 | 0.33 | 0.26 | 0.42 | 0.27 | 0.89 | 0.71 | 1.07 | 1.03 |
25 | 0.35 | 0.27 | 0.43 | 0.22 | 0.92 | 0.75 | 1.10 | 0.92 |
26 | 0.36 | 0.28 | 0.45 | 0.23 | 0.95 | 0.79 | 1.13 | 0.87 |
27 | 0.37 | 0.31 | 0.46 | 0.32 | 0.98 | 0.84 | 1.16 | 1.07 |
28 | 0.39 | 0.32 | 0.47 | 0.27 | 1.02 | 0.87 | 1.18 | 1.22 |
29 | 0.41 | 0.33 | 0.48 | 0.27 | 1.06 | 0.91 | 1.21 | 1.10 |
30 | 0.42 | 0.35 | 0.50 | 0.31 | 1.09 | 0.95 | 1.23 | 1.02 |
31 | 0.44 | 0.37 | 0.51 | 0.31 | 1.13 | 1.00 | 1.27 | 1.26 |
32 | 0.46 | 0.39 | 0.53 | 0.34 | 1.17 | 1.04 | 1.32 | 1.31 |
33 | 0.47 | 0.41 | 0.55 | 0.51 | 1.21 | 1.08 | 1.35 | 1.45 |
34 | 0.49 | 0.42 | 0.57 | 0.54 | 1.25 | 1.12 | 1.39 | 1.61 |
35 | 0.51 | 0.43 | 0.58 | 0.44 | 1.29 | 1.15 | 1.43 | 1.47 |
36 | 0.53 | 0.45 | 0.61 | 0.51 | 1.33 | 1.21 | 1.47 | 1.34 |
37 | 0.55 | 0.46 | 0.63 | 0.47 | 1.38 | 1.24 | 1.51 | 1.39 |
38 | 0.57 | 0.49 | 0.65 | 0.53 | 1.42 | 1.28 | 1.56 | 1.44 |
39 | 0.59 | 0.51 | 0.67 | 0.51 | 1.47 | 1.33 | 1.60 | 1.68 |
40 | 0.61 | 0.53 | 0.69 | 0.65 | 1.51 | 1.37 | 1.66 | 1.58 |
41 | 0.63 | 0.55 | 0.71 | 0.61 | 1.55 | 1.40 | 1.69 | 1.70 |
42 | 0.65 | 0.57 | 0.73 | 0.69 | 1.60 | 1.45 | 1.74 | 1.66 |
43 | 0.67 | 0.59 | 0.76 | 0.52 | 1.65 | 1.50 | 1.79 | 1.68 |
44 | 0.69 | 0.60 | 0.78 | 0.73 | 1.69 | 1.54 | 1.85 | 1.87 |
45 | 0.71 | 0.62 | 0.80 | 0.85 | 1.74 | 1.60 | 1.89 | 1.89 |
46 | 0.73 | 0.65 | 0.83 | 0.76 | 1.79 | 1.64 | 1.95 | 1.94 |
47 | 0.76 | 0.67 | 0.85 | 0.72 | 1.84 | 1.69 | 1.99 | 1.86 |
48 | 0.78 | 0.69 | 0.88 | 0.77 | 1.89 | 1.73 | 2.05 | 1.85 |
49 | 0.81 | 0.71 | 0.90 | 0.82 | 1.94 | 1.79 | 2.10 | 1.97 |
50 | 0.83 | 0.74 | 0.93 | 0.81 | 1.99 | 1.85 | 2.15 | 2.12 |
51 | 0.85 | 0.76 | 0.94 | 0.87 | 2.04 | 1.90 | 2.19 | 2.09 |
52 | 0.88 | 0.80 | 0.97 | 0.93 | 2.10 | 1.95 | 2.25 | 2.20 |
53 | 0.90 | 0.81 | 1.00 | 0.96 | 2.14 | 2.00 | 2.31 | 2.29 |
54 | 0.93 | 0.83 | 1.03 | 0.97 | 2.20 | 2.04 | 2.36 | 2.41 |
55 | 0.95 | 0.86 | 1.05 | 1.02 | 2.26 | 2.11 | 2.41 | 2.50 |
56 | 0.98 | 0.89 | 1.08 | 1.06 | 2.31 | 2.14 | 2.47 | 2.66 |
57 | 1.01 | 0.91 | 1.11 | 1.09 | 2.37 | 2.19 | 2.54 | 2.56 |
58 | 1.04 | 0.93 | 1.15 | 1.03 | 2.41 | 2.23 | 2.60 | 2.66 |
59 | 1.06 | 0.96 | 1.18 | 1.12 | 2.47 | 2.28 | 2.68 | 2.71 |
60 | 1.09 | 0.98 | 1.21 | 1.10 | 2.53 | 2.32 | 2.75 | 2.67 |
61 | 1.11 | 0.99 | 1.25 | 1.02 | 2.58 | 2.33 | 2.85 | 2.62 |
62 | 1.14 | 1.02 | 1.29 | 1.13 | 2.64 | 2.39 | 2.92 | 2.77 |
63 | 1.17 | 1.02 | 1.34 | 1.00 | 2.69 | 2.41 | 3.02 | 2.58 |
64 | 1.20 | 1.04 | 1.40 | 0.92 | 2.76 | 2.44 | 3.12 | 2.43 |
65 | 1.22 | 1.05 | 1.44 | 1.00 | 2.81 | 2.45 | 3.21 | 2.09 |
Panel E: 0430 - Symptomatic HIV | ||||||||
18 | 0.58 | 0.40 | 0.81 | 0.40 | 0.22 | 0.14 | 0.32 | 0.61 |
19 | 0.66 | 0.47 | 0.88 | 0.73 | 0.25 | 0.17 | 0.35 | 0.22 |
20 | 0.73 | 0.54 | 0.95 | 0.72 | 0.28 | 0.19 | 0.38 | 0.25 |
21 | 0.80 | 0.62 | 1.02 | 0.73 | 0.31 | 0.22 | 0.42 | 0.28 |
22 | 0.88 | 0.69 | 1.09 | 0.82 | 0.35 | 0.26 | 0.45 | 0.36 |
23 | 0.95 | 0.76 | 1.16 | 0.92 | 0.38 | 0.28 | 0.48 | 0.45 |
24 | 1.03 | 0.84 | 1.24 | 0.90 | 0.41 | 0.32 | 0.52 | 0.35 |
25 | 1.10 | 0.93 | 1.30 | 1.09 | 0.45 | 0.36 | 0.54 | 0.38 |
26 | 1.18 | 1.00 | 1.37 | 1.33 | 0.48 | 0.39 | 0.57 | 0.43 |
27 | 1.24 | 1.07 | 1.42 | 1.44 | 0.51 | 0.43 | 0.61 | 0.40 |
28 | 1.31 | 1.15 | 1.49 | 1.27 | 0.54 | 0.46 | 0.63 | 0.52 |
29 | 1.37 | 1.21 | 1.55 | 1.14 | 0.57 | 0.48 | 0.66 | 0.66 |
30 | 1.43 | 1.26 | 1.59 | 1.53 | 0.59 | 0.51 | 0.69 | 0.57 |
31 | 1.48 | 1.32 | 1.64 | 1.42 | 0.62 | 0.53 | 0.71 | 0.66 |
32 | 1.51 | 1.36 | 1.66 | 1.39 | 0.64 | 0.54 | 0.73 | 0.70 |
33 | 1.55 | 1.38 | 1.71 | 1.28 | 0.65 | 0.57 | 0.75 | 0.84 |
34 | 1.58 | 1.41 | 1.73 | 1.25 | 0.66 | 0.57 | 0.76 | 0.65 |
35 | 1.59 | 1.42 | 1.75 | 1.26 | 0.67 | 0.58 | 0.77 | 0.58 |
36 | 1.60 | 1.43 | 1.75 | 1.64 | 0.67 | 0.58 | 0.77 | 0.55 |
37 | 1.60 | 1.43 | 1.76 | 1.30 | 0.67 | 0.58 | 0.77 | 0.72 |
38 | 1.59 | 1.42 | 1.75 | 1.71 | 0.67 | 0.58 | 0.77 | 0.66 |
39 | 1.57 | 1.40 | 1.72 | 1.86 | 0.66 | 0.57 | 0.75 | 0.77 |
40 | 1.54 | 1.38 | 1.69 | 1.71 | 0.65 | 0.56 | 0.74 | 0.69 |
41 | 1.51 | 1.35 | 1.65 | 1.62 | 0.63 | 0.55 | 0.72 | 0.50 |
42 | 1.46 | 1.32 | 1.60 | 1.69 | 0.61 | 0.53 | 0.70 | 0.61 |
43 | 1.41 | 1.27 | 1.54 | 1.68 | 0.59 | 0.50 | 0.67 | 0.50 |
44 | 1.35 | 1.22 | 1.49 | 1.70 | 0.56 | 0.48 | 0.64 | 0.44 |
45 | 1.30 | 1.17 | 1.42 | 1.64 | 0.53 | 0.46 | 0.60 | 0.48 |
46 | 1.22 | 1.12 | 1.34 | 1.46 | 0.50 | 0.43 | 0.58 | 0.49 |
47 | 1.15 | 1.05 | 1.27 | 1.36 | 0.47 | 0.40 | 0.54 | 0.37 |
48 | 1.08 | 0.98 | 1.18 | 1.39 | 0.43 | 0.37 | 0.50 | 0.38 |
49 | 1.00 | 0.92 | 1.10 | 1.01 | 0.40 | 0.35 | 0.46 | 0.34 |
50 | 0.93 | 0.84 | 1.02 | 1.01 | 0.36 | 0.31 | 0.42 | 0.32 |
51 | 0.85 | 0.77 | 0.93 | 0.94 | 0.33 | 0.28 | 0.38 | 0.33 |
52 | 0.77 | 0.70 | 0.86 | 0.74 | 0.30 | 0.25 | 0.35 | 0.27 |
53 | 0.70 | 0.63 | 0.79 | 0.74 | 0.27 | 0.22 | 0.32 | 0.23 |
54 | 0.63 | 0.55 | 0.71 | 0.76 | 0.24 | 0.20 | 0.28 | 0.19 |
55 | 0.56 | 0.49 | 0.65 | 0.56 | 0.21 | 0.17 | 0.25 | 0.17 |
56 | 0.49 | 0.43 | 0.58 | 0.55 | 0.18 | 0.14 | 0.22 | 0.18 |
57 | 0.43 | 0.37 | 0.52 | 0.43 | 0.15 | 0.12 | 0.20 | 0.13 |
58 | 0.37 | 0.31 | 0.46 | 0.42 | 0.13 | 0.10 | 0.17 | 0.11 |
59 | 0.32 | 0.26 | 0.41 | 0.43 | 0.11 | 0.08 | 0.15 | 0.11 |
60 | 0.27 | 0.22 | 0.35 | 0.26 | 0.09 | 0.07 | 0.13 | 0.10 |
61 | 0.23 | 0.18 | 0.31 | 0.28 | 0.08 | 0.05 | 0.11 | 0.07 |
62 | 0.19 | 0.14 | 0.27 | 0.17 | 0.06 | 0.04 | 0.10 | 0.06 |
63 | 0.16 | 0.12 | 0.23 | 0.20 | 0.05 | 0.03 | 0.08 | 0.04 |
64 | 0.13 | 0.09 | 0.20 | 0.23 | 0.04 | 0.02 | 0.07 | 0.05 |
65 | 0.11 | 0.07 | 0.17 | 0.11 | 0.03 | 0.02 | 0.05 | 0.02 |
Circulatory System Diseases
Men constitute 62.7% of all cases with a primary or secondary diagnosis pertaining to the circulatory system. They account for more than 70% of cases of chronic ischemic heart disease (0.22 mean correlation), acute myocardial infarction, and cardiomyopathy; and for more than 65% of the diagnoses of heart failure, peripheral vascular disease, and diseases of the aortic valve. Panel C of Chart 3 shows the conditional probabilities associated with a diagnosis of cardiomyopathy as a function of age and sex.
Injuries
In 64.2% of cases involving injuries, the claimant is male. Over 70% of the diagnoses of fractures of the vertebral column, amputation, and open wounds of the upper and lower limbs are for male applicants, as are more than 60% of the intracranial injuries, fractures of the lower limb, and other fractures of bones.
Musculoskeletal Impairments
Unlike affective/mood disorders, which are more frequent among women, the male share of diagnoses for the second most common impairment (disorders of the back) stands at 54.7%. This is only slightly higher than the men's share of all claims (53.5%). There are nevertheless three musculoskeletal diagnoses negatively correlated with male applicants. Only 15% of claimants with a diagnosis involving a diffuse disease of the connective tissue are men, yielding a posterior mean correlation estimate of −0.34. In addition, the male composition of claimants with curvature of the spine and with rheumatoid arthritis is 38% and 31%, respectively. Panel D of Chart 3 shows the incidence probabilities for rheumatoid arthritis, by age and sex. Overall, men constitute 52% of the musculoskeletal diagnoses.
Infectious/Parasitic Diseases
Men account for 68.3% of infectious/parasitic disease diagnoses overall. The two most common impairments (symptomatic and asymptomatic HIV) show much higher shares of incidence for men, at 75.7% and 69.9%, respectively. The estimated age-specific probabilities for a primary or secondary diagnosis of symptomatic HIV, by sex, appear in Panel E of Chart 3. A third diagnosis (sarcoidosis) is a disease characterized by the formation of clusters of chronic inflammatory cells in one or multiple organs. It often affects the lungs and the lymphatic system and is typically more prevalent in women. Only about 41% of claims with a sarcoidosis diagnosis involve men.
Nervous System and Sense Organ Diseases
About 52% of diagnoses in the nervous system/sense organs category involve male claimants. Men dominate diagnoses of Parkinson's disease and blindness/low vision (69.6% and 62.7%, respectively). On the other hand, multiple sclerosis, migraine, and carpal tunnel syndrome diagnoses involve men in about one-third of the cases or less.
Other Disorders
Additional impairments with a higher-than-average share of male claimants include sleep-related breathing disorders (respiratory system), gout (endocrine/nutritional/metabolic group), and both hernias and chronic liver disease/cirrhosis (digestive impairments). On the other hand, female-dominated diagnoses in Table 4 are obesity and all disorders of the thyroid (endocrine/nutritional/metabolic category) and asthma (respiratory system).
In summary, male claimants have higher shares of diagnoses in the following diagnostic groups: circulatory system, injuries, infectious/parasitic diseases (where symptomatic HIV is the most common impairment), and digestive system (because of the high incidence of chronic liver disease/cirrhosis). Men also dominate diagnoses in the genitourinary group (59.7%), where the most common diagnosis is chronic renal failure.14 Men account for about 52% of diagnoses in the musculoskeletal system and in the nervous system/sense organs categories. They also make up 51.9% of the diagnoses in the endocrine/nutritional/metabolic group, where men's greater proportion of diabetes diagnoses offsets the higher share of women diagnosed with obesity. Finally, men account for less than half of the respiratory system, mental impairment, and malignant neoplasm diagnoses.
Variables Correlated with Concurrent Status
Of the more than 1.5 million qualified DI claimants in 2009, 52.5% applied concurrently to SSI. Of the concurrent-claim applicants, 52.9% are men, suggesting little difference in the aggregate sex composition of the two subpopulations. However, concurrent-claim applicants are substantially younger. Chart 4 shows estimated probabilities that DI applicants concurrently apply for SSI, by age. About 70% of DI claimants aged 18 through 35 apply concurrently. However, the concurrent-claim share of applicants declines steadily with age, so that by age 60, DI-only filers compose roughly three-quarters of initial claims. The difference in median age between concurrent-claim and DI-only applicants is 8 years.
Concurrent-claim applicants as a percentage of initial disability-benefit claimants, by age, 2009
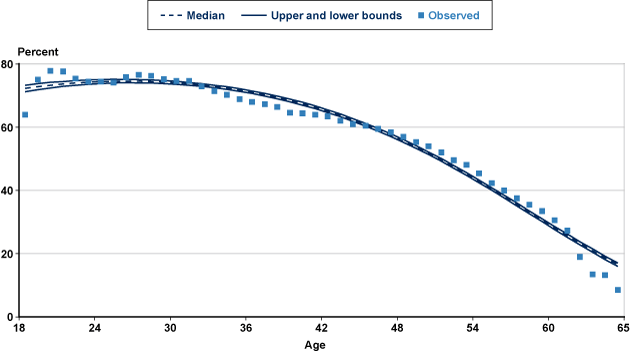
Age | Median | Lower bound | Upper bound | Observed |
---|---|---|---|---|
18 | 72.27 | 71.22 | 73.27 | 63.99 |
19 | 72.81 | 71.84 | 73.74 | 75.06 |
20 | 73.26 | 72.36 | 74.14 | 77.77 |
21 | 73.67 | 72.88 | 74.44 | 77.65 |
22 | 73.99 | 73.27 | 74.73 | 75.35 |
23 | 74.23 | 73.54 | 74.90 | 74.36 |
24 | 74.41 | 73.77 | 75.05 | 74.39 |
25 | 74.50 | 73.93 | 75.12 | 74.05 |
26 | 74.55 | 74.05 | 75.09 | 75.80 |
27 | 74.53 | 74.01 | 75.03 | 76.50 |
28 | 74.42 | 73.93 | 74.92 | 76.25 |
29 | 74.25 | 73.78 | 74.73 | 75.17 |
30 | 74.01 | 73.57 | 74.46 | 74.62 |
31 | 73.71 | 73.27 | 74.12 | 74.66 |
32 | 73.33 | 72.92 | 73.74 | 72.86 |
33 | 72.87 | 72.45 | 73.27 | 71.40 |
34 | 72.33 | 71.90 | 72.75 | 70.23 |
35 | 71.71 | 71.29 | 72.12 | 68.91 |
36 | 71.03 | 70.62 | 71.41 | 67.99 |
37 | 70.26 | 69.83 | 70.65 | 67.23 |
38 | 69.40 | 68.98 | 69.81 | 66.41 |
39 | 68.45 | 68.01 | 68.87 | 64.63 |
40 | 67.40 | 66.97 | 67.86 | 64.40 |
41 | 66.27 | 65.82 | 66.70 | 63.90 |
42 | 65.06 | 64.60 | 65.48 | 63.37 |
43 | 63.74 | 63.27 | 64.16 | 61.99 |
44 | 62.31 | 61.87 | 62.71 | 60.99 |
45 | 60.78 | 60.36 | 61.21 | 60.45 |
46 | 59.18 | 58.73 | 59.58 | 59.48 |
47 | 57.45 | 57.01 | 57.86 | 58.41 |
48 | 55.62 | 55.21 | 56.08 | 56.86 |
49 | 53.71 | 53.29 | 54.14 | 55.29 |
50 | 51.72 | 51.31 | 52.12 | 53.92 |
51 | 49.62 | 49.20 | 50.03 | 52.02 |
52 | 47.44 | 47.01 | 47.84 | 49.58 |
53 | 45.16 | 44.72 | 45.58 | 48.03 |
54 | 42.81 | 42.41 | 43.25 | 45.44 |
55 | 40.43 | 40.01 | 40.81 | 42.28 |
56 | 38.00 | 37.57 | 38.43 | 39.96 |
57 | 35.52 | 35.08 | 35.95 | 37.47 |
58 | 33.04 | 32.62 | 33.47 | 35.43 |
59 | 30.55 | 30.08 | 31.01 | 33.39 |
60 | 28.07 | 27.57 | 28.56 | 30.54 |
61 | 25.63 | 25.11 | 26.14 | 27.28 |
62 | 23.24 | 22.73 | 23.73 | 18.88 |
63 | 20.90 | 20.38 | 21.45 | 13.35 |
64 | 18.65 | 18.11 | 19.19 | 13.16 |
65 | 16.54 | 15.97 | 17.03 | 8.49 |
Concurrent-claim applicants represent a unique population whose members have enough of a recent work history to qualify under DI, yet are poor enough to meet SSI's strict asset limits. Concurrent-claim applicants have a lower socioeconomic status than DI-only applicants (lower earnings histories, fewer assets, and lower educational attainment). Moreover, younger workers are assumed to be more capable of adapting to new work environments and thus, they face more stringent disability determination standards under the vocational grid rules. In addition, because different impairments vary in their typical age of onset, the diagnostic mix of concurrent-claim applicants can differ substantially from that of DI-only claimants. All of these factors contribute to the large differences observed in initial allowance rates. Some 50.1% of DI-only claimants are initially allowed, compared to only 26.8% of concurrent-claim applicants.
Table 5 displays some of the correlation estimates among the binary variables in the model that are associated with concurrent-claim status. There is an overlap between concurrent-claim applicants and reapplicants. About 65% of claimants with a prior application are also concurrent-claim applicants, resulting in a posterior mean correlation estimate of 0.25. The implication is that claimants who have applied to the DI program before are also more likely to subsequently apply to both DI and SSI simultaneously. It is plausible that, over time, denied unemployed claimants consume enough of their savings and assets to meet SSI eligibility criteria as well.
Diagnostic group, impairment code, and diagnosis | Concurrent claims as a percentage of applicants | Correlation (posterior mean) | t-statistic | Numerical standard error |
---|---|---|---|---|
Reapplicant | 65.07 | 0.2502 | 58.90 | 0.000048 |
Neoplasms (malignant) | ||||
1910 - Brain cancer | 25.28 | -0.2144 | -11.68 | 0.001167 |
2070 - Leukemias | 30.98 | -0.1597 | -8.87 | 0.001123 |
1570 - Pancreatic cancer | 24.62 | -0.1429 | -7.00 | 0.001190 |
2030 - Multiple myeloma | 20.06 | -0.1337 | -5.21 | 0.001994 |
2020 - Lymphoma | 36.64 | -0.1257 | -7.47 | 0.000913 |
1500 - Esophageal cancer | 26.60 | -0.1188 | -5.24 | 0.002049 |
1740 - Breast cancer | 34.67 | -0.1145 | -10.69 | 0.000493 |
1830 - Ovarian cancer | 32.30 | -0.1123 | -5.10 | 0.001828 |
Mental disorders | ||||
3040 - Substance addiction (drugs) | 84.29 | 0.2323 | 17.34 | 0.000683 |
3030 - Substance addiction (alcohol) | 76.29 | 0.1951 | 16.34 | 0.000378 |
3010 - Personality disorders | 77.58 | 0.1610 | 14.64 | 0.000334 |
2950 - Schizophrenic and other psychotic disorders | 74.18 | 0.1434 | 15.71 | 0.000248 |
2990 - Autistic disorders | 47.05 | -0.1605 | -5.82 | 0.002451 |
3180 - Intellectual disability | 48.76 | -0.1130 | -10.15 | 0.000412 |
Nervous system and sense organ diseases | ||||
3350 - Anterior horn cell disease | 12.80 | -0.2636 | -8.20 | 0.003283 |
3400 - Multiple sclerosis | 32.56 | -0.2166 | -17.92 | 0.000438 |
3320 - Parkinson's disease | 16.60 | -0.2077 | -10.21 | 0.001094 |
3430 - Cerebral palsy | 41.56 | -0.1547 | -6.40 | 0.002038 |
3590 - Muscular dystrophies | 36.00 | -0.1541 | -5.74 | 0.002111 |
3360 - Other spinal cord disorders | 39.50 | -0.1250 | -5.41 | 0.001868 |
3540 - Carpal tunnel syndrome | 40.54 | -0.1083 | -8.79 | 0.000422 |
3580 - Myoneural disorders | 38.86 | -0.1041 | -4.20 | 0.001822 |
Infectious and parasitic diseases | ||||
0440 - Asymptomatic HIV | 79.73 | 0.1821 | 10.89 | 0.000941 |
0430 - Symptomatic HIV | 73.13 | 0.1294 | 9.42 | 0.000593 |
Circulatory system diseases | ||||
4010 - Essential hypertension | 56.08 | 0.1433 | 23.05 | 0.000146 |
Digestive system diseases | ||||
5710 - Chronic liver disease and cirrhosis | 60.04 | 0.1244 | 12.50 | 0.000288 |
Injuries | ||||
8060 - Fracture of vertebral column | 46.90 | -0.1194 | -5.71 | 0.001320 |
SOURCE: Author's calculations using DRF 100% extract (for descriptive statistics) and MVP estimation model fitted to a DRF 10% random sample (for correlation estimates). | ||||
NOTES: Correlation estimates are selected for inclusion on the basis of having absolute values for the posterior mean of at least 0.10 and, generally, a t-statistic greater than 2.00.
Data are for applicants who cleared step 1 of the disability determination process.
Diagnoses are arranged by estimated correlation value. Negative correlations are listed by absolute value.
Diagnoses identified as "other" may be defined in the context of impairments not shown in this table.
|
Malignant Neoplasms
Neoplastic impairments, which have very high initial allowance rates and late average ages of onset, are negatively correlated with concurrent-claim status. For example, only 25% of applicants with either a primary or secondary diagnosis involving a malignant neoplasm of the brain filed concurrent claims, resulting in a posterior mean correlation of −0.21. Other cancers with low proportions of concurrent-claim applicants and negative correlations include leukemia, lymphoma, multiple myeloma, and malignant neoplasms of the pancreas, esophagus, ovaries, and breast. The conditional probabilities associated with the latter appear in Panel A of Chart 5. The proportion of concurrent-claim applicants among all neoplastic diagnoses is 33.2% (not shown).
Estimated incidence probabilities of selected diagnoses for initial DI-only and concurrent-claim applicants, by age, 2009
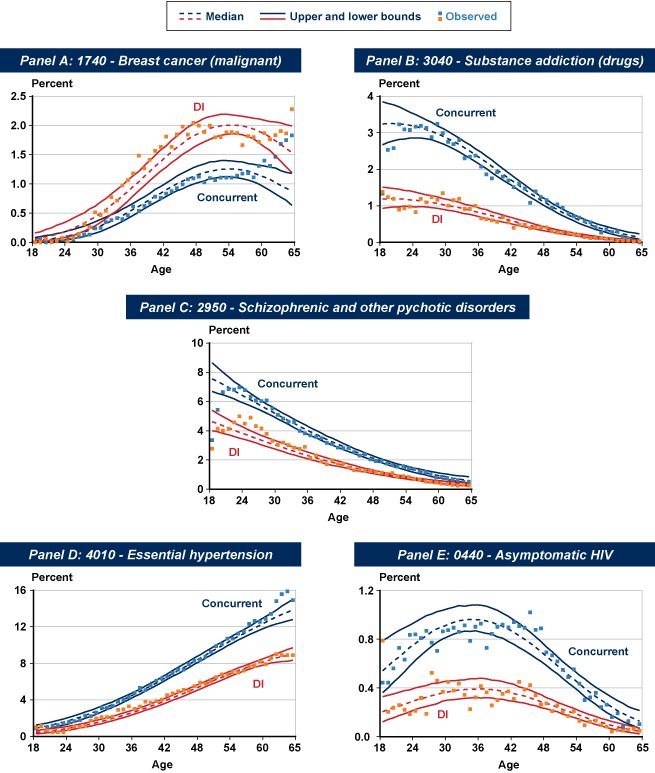
Age | Concurrent-claim | DI-only | ||||||
---|---|---|---|---|---|---|---|---|
Median | Lower bound | Upper bound | Observed | Median | Lower bound | Upper bound | Observed | |
Panel A: 1740 - Breast cancer (malignant) | ||||||||
18 | 0.03 | 0.01 | 0.08 | 0.00 | 0.06 | 0.02 | 0.16 | 0.00 |
19 | 0.04 | 0.01 | 0.10 | 0.07 | 0.08 | 0.02 | 0.18 | 0.00 |
20 | 0.05 | 0.01 | 0.12 | 0.00 | 0.10 | 0.03 | 0.22 | 0.06 |
21 | 0.06 | 0.02 | 0.13 | 0.03 | 0.12 | 0.04 | 0.25 | 0.00 |
22 | 0.07 | 0.03 | 0.15 | 0.04 | 0.14 | 0.06 | 0.29 | 0.03 |
23 | 0.09 | 0.04 | 0.17 | 0.05 | 0.17 | 0.07 | 0.33 | 0.07 |
24 | 0.11 | 0.05 | 0.20 | 0.10 | 0.20 | 0.09 | 0.37 | 0.02 |
25 | 0.13 | 0.06 | 0.22 | 0.05 | 0.24 | 0.12 | 0.41 | 0.08 |
26 | 0.16 | 0.08 | 0.25 | 0.09 | 0.28 | 0.16 | 0.46 | 0.15 |
27 | 0.18 | 0.10 | 0.28 | 0.14 | 0.33 | 0.19 | 0.50 | 0.31 |
28 | 0.21 | 0.13 | 0.32 | 0.14 | 0.38 | 0.24 | 0.57 | 0.26 |
29 | 0.25 | 0.16 | 0.35 | 0.24 | 0.44 | 0.29 | 0.62 | 0.52 |
30 | 0.28 | 0.19 | 0.39 | 0.25 | 0.50 | 0.34 | 0.70 | 0.47 |
31 | 0.33 | 0.22 | 0.43 | 0.35 | 0.57 | 0.41 | 0.75 | 0.54 |
32 | 0.36 | 0.27 | 0.47 | 0.38 | 0.64 | 0.48 | 0.83 | 0.77 |
33 | 0.41 | 0.31 | 0.52 | 0.42 | 0.72 | 0.54 | 0.90 | 0.72 |
34 | 0.46 | 0.36 | 0.57 | 0.40 | 0.79 | 0.62 | 0.98 | 0.97 |
35 | 0.51 | 0.41 | 0.62 | 0.46 | 0.87 | 0.71 | 1.07 | 1.06 |
36 | 0.57 | 0.46 | 0.67 | 0.62 | 0.96 | 0.80 | 1.14 | 1.18 |
37 | 0.62 | 0.52 | 0.73 | 0.53 | 1.04 | 0.89 | 1.22 | 1.49 |
38 | 0.68 | 0.58 | 0.78 | 0.62 | 1.13 | 0.97 | 1.31 | 1.27 |
39 | 0.73 | 0.63 | 0.84 | 0.68 | 1.21 | 1.05 | 1.40 | 1.41 |
40 | 0.78 | 0.68 | 0.90 | 0.79 | 1.30 | 1.13 | 1.49 | 1.56 |
41 | 0.84 | 0.73 | 0.96 | 0.76 | 1.38 | 1.22 | 1.59 | 1.63 |
42 | 0.90 | 0.78 | 1.01 | 0.84 | 1.47 | 1.29 | 1.65 | 1.81 |
43 | 0.95 | 0.84 | 1.07 | 0.88 | 1.55 | 1.37 | 1.74 | 1.64 |
44 | 1.00 | 0.88 | 1.13 | 0.93 | 1.62 | 1.43 | 1.82 | 1.85 |
45 | 1.05 | 0.92 | 1.19 | 0.99 | 1.69 | 1.51 | 1.90 | 1.98 |
46 | 1.09 | 0.96 | 1.23 | 1.08 | 1.77 | 1.56 | 1.97 | 1.84 |
47 | 1.13 | 1.00 | 1.28 | 1.10 | 1.82 | 1.62 | 2.03 | 2.04 |
48 | 1.16 | 1.03 | 1.31 | 1.12 | 1.87 | 1.67 | 2.07 | 1.99 |
49 | 1.19 | 1.06 | 1.34 | 1.03 | 1.91 | 1.72 | 2.13 | 1.89 |
50 | 1.22 | 1.09 | 1.38 | 1.10 | 1.95 | 1.76 | 2.15 | 2.00 |
51 | 1.23 | 1.10 | 1.38 | 1.06 | 1.97 | 1.81 | 2.17 | 1.80 |
52 | 1.25 | 1.12 | 1.40 | 1.11 | 1.99 | 1.83 | 2.19 | 1.80 |
53 | 1.25 | 1.13 | 1.40 | 1.11 | 2.00 | 1.85 | 2.19 | 1.88 |
54 | 1.26 | 1.12 | 1.39 | 1.10 | 2.01 | 1.86 | 2.18 | 1.89 |
55 | 1.25 | 1.10 | 1.38 | 1.14 | 2.00 | 1.85 | 2.17 | 1.87 |
56 | 1.24 | 1.09 | 1.38 | 1.17 | 1.99 | 1.84 | 2.16 | 1.67 |
57 | 1.22 | 1.07 | 1.35 | 1.19 | 1.97 | 1.81 | 2.14 | 1.82 |
58 | 1.19 | 1.04 | 1.34 | 1.11 | 1.94 | 1.77 | 2.12 | 1.80 |
59 | 1.15 | 0.99 | 1.32 | 1.31 | 1.90 | 1.71 | 2.11 | 1.72 |
60 | 1.12 | 0.94 | 1.31 | 1.40 | 1.86 | 1.63 | 2.09 | 1.87 |
61 | 1.08 | 0.89 | 1.28 | 1.31 | 1.80 | 1.57 | 2.07 | 1.76 |
62 | 1.03 | 0.83 | 1.28 | 1.46 | 1.74 | 1.48 | 2.06 | 1.83 |
63 | 0.97 | 0.77 | 1.23 | 1.69 | 1.67 | 1.39 | 2.04 | 1.90 |
64 | 0.93 | 0.72 | 1.20 | 1.75 | 1.61 | 1.29 | 2.01 | 1.87 |
65 | 0.88 | 0.64 | 1.18 | 1.83 | 1.54 | 1.19 | 1.99 | 2.28 |
Panel B: 3040 - Substance addiction (drugs) | ||||||||
18 | 3.24 | 2.67 | 3.85 | 1.33 | 1.19 | 0.94 | 1.51 | 1.38 |
19 | 3.25 | 2.74 | 3.80 | 2.54 | 1.19 | 0.95 | 1.49 | 1.24 |
20 | 3.25 | 2.78 | 3.77 | 2.58 | 1.20 | 0.97 | 1.48 | 1.19 |
21 | 3.24 | 2.81 | 3.71 | 3.23 | 1.19 | 0.97 | 1.46 | 0.89 |
22 | 3.23 | 2.83 | 3.64 | 3.09 | 1.19 | 0.99 | 1.42 | 0.94 |
23 | 3.20 | 2.85 | 3.57 | 3.08 | 1.17 | 0.98 | 1.41 | 0.98 |
24 | 3.17 | 2.85 | 3.50 | 3.17 | 1.15 | 0.97 | 1.37 | 0.83 |
25 | 3.14 | 2.86 | 3.42 | 3.18 | 1.13 | 0.98 | 1.34 | 1.19 |
26 | 3.09 | 2.84 | 3.35 | 3.13 | 1.11 | 0.97 | 1.30 | 1.10 |
27 | 3.04 | 2.81 | 3.28 | 2.89 | 1.09 | 0.95 | 1.28 | 1.24 |
28 | 2.97 | 2.76 | 3.19 | 3.24 | 1.07 | 0.93 | 1.25 | 1.16 |
29 | 2.91 | 2.70 | 3.12 | 3.00 | 1.04 | 0.90 | 1.20 | 1.34 |
30 | 2.84 | 2.63 | 3.04 | 2.75 | 1.01 | 0.88 | 1.17 | 1.01 |
31 | 2.76 | 2.56 | 2.95 | 2.69 | 0.98 | 0.86 | 1.13 | 1.16 |
32 | 2.68 | 2.48 | 2.85 | 2.80 | 0.95 | 0.82 | 1.09 | 1.19 |
33 | 2.59 | 2.39 | 2.75 | 2.31 | 0.92 | 0.79 | 1.04 | 0.91 |
34 | 2.49 | 2.30 | 2.67 | 2.34 | 0.88 | 0.77 | 1.02 | 0.91 |
35 | 2.39 | 2.21 | 2.56 | 2.38 | 0.85 | 0.73 | 0.98 | 0.99 |
36 | 2.30 | 2.11 | 2.47 | 2.07 | 0.81 | 0.69 | 0.93 | 0.66 |
37 | 2.19 | 2.02 | 2.36 | 1.86 | 0.77 | 0.65 | 0.89 | 0.64 |
38 | 2.09 | 1.90 | 2.25 | 1.75 | 0.73 | 0.62 | 0.83 | 0.60 |
39 | 1.98 | 1.81 | 2.14 | 1.93 | 0.69 | 0.60 | 0.80 | 0.56 |
40 | 1.87 | 1.71 | 2.02 | 1.80 | 0.65 | 0.56 | 0.75 | 0.68 |
41 | 1.76 | 1.62 | 1.91 | 1.70 | 0.62 | 0.53 | 0.71 | 0.55 |
42 | 1.66 | 1.53 | 1.80 | 1.52 | 0.57 | 0.49 | 0.67 | 0.40 |
43 | 1.55 | 1.42 | 1.69 | 1.60 | 0.54 | 0.46 | 0.62 | 0.48 |
44 | 1.45 | 1.33 | 1.58 | 1.47 | 0.50 | 0.43 | 0.58 | 0.49 |
45 | 1.35 | 1.24 | 1.47 | 1.08 | 0.46 | 0.40 | 0.54 | 0.39 |
46 | 1.25 | 1.15 | 1.36 | 1.39 | 0.43 | 0.37 | 0.50 | 0.41 |
47 | 1.16 | 1.06 | 1.26 | 1.24 | 0.40 | 0.34 | 0.46 | 0.41 |
48 | 1.07 | 0.97 | 1.17 | 1.15 | 0.36 | 0.31 | 0.41 | 0.41 |
49 | 0.98 | 0.89 | 1.07 | 0.96 | 0.33 | 0.28 | 0.38 | 0.31 |
50 | 0.89 | 0.81 | 0.98 | 1.04 | 0.30 | 0.25 | 0.35 | 0.27 |
51 | 0.82 | 0.73 | 0.90 | 0.95 | 0.27 | 0.23 | 0.32 | 0.27 |
52 | 0.75 | 0.66 | 0.83 | 0.79 | 0.24 | 0.21 | 0.29 | 0.26 |
53 | 0.67 | 0.59 | 0.76 | 0.72 | 0.22 | 0.19 | 0.26 | 0.23 |
54 | 0.61 | 0.52 | 0.69 | 0.68 | 0.19 | 0.16 | 0.23 | 0.21 |
55 | 0.54 | 0.46 | 0.63 | 0.58 | 0.17 | 0.14 | 0.21 | 0.14 |
56 | 0.49 | 0.41 | 0.58 | 0.55 | 0.15 | 0.12 | 0.19 | 0.12 |
57 | 0.43 | 0.36 | 0.52 | 0.41 | 0.13 | 0.11 | 0.17 | 0.11 |
58 | 0.39 | 0.32 | 0.47 | 0.27 | 0.12 | 0.09 | 0.15 | 0.11 |
59 | 0.34 | 0.27 | 0.43 | 0.36 | 0.10 | 0.08 | 0.13 | 0.09 |
60 | 0.30 | 0.23 | 0.39 | 0.28 | 0.09 | 0.07 | 0.12 | 0.09 |
61 | 0.26 | 0.19 | 0.35 | 0.30 | 0.08 | 0.05 | 0.11 | 0.05 |
62 | 0.23 | 0.16 | 0.32 | 0.17 | 0.06 | 0.04 | 0.09 | 0.05 |
63 | 0.20 | 0.14 | 0.28 | 0.10 | 0.05 | 0.04 | 0.08 | 0.05 |
64 | 0.17 | 0.11 | 0.26 | 0.04 | 0.05 | 0.03 | 0.07 | 0.02 |
65 | 0.15 | 0.09 | 0.23 | 0.00 | 0.04 | 0.02 | 0.06 | 0.03 |
Panel C: 2950 - Schizophrenic and other pychotic disorders | ||||||||
18 | 7.54 | 6.68 | 8.63 | 3.34 | 4.62 | 3.99 | 5.40 | 2.77 |
19 | 7.36 | 6.55 | 8.31 | 5.41 | 4.47 | 3.92 | 5.16 | 4.12 |
20 | 7.15 | 6.45 | 7.99 | 6.64 | 4.33 | 3.80 | 4.95 | 3.98 |
21 | 6.95 | 6.32 | 7.67 | 6.84 | 4.18 | 3.72 | 4.74 | 4.14 |
22 | 6.73 | 6.17 | 7.35 | 6.80 | 4.04 | 3.61 | 4.53 | 4.58 |
23 | 6.53 | 6.00 | 7.12 | 6.95 | 3.88 | 3.50 | 4.35 | 4.99 |
24 | 6.31 | 5.86 | 6.83 | 6.77 | 3.75 | 3.40 | 4.18 | 4.46 |
25 | 6.11 | 5.69 | 6.54 | 6.31 | 3.62 | 3.28 | 3.99 | 4.89 |
26 | 5.90 | 5.53 | 6.29 | 6.07 | 3.47 | 3.17 | 3.84 | 4.33 |
27 | 5.69 | 5.36 | 6.06 | 6.03 | 3.34 | 3.04 | 3.68 | 4.15 |
28 | 5.48 | 5.19 | 5.81 | 6.06 | 3.21 | 2.92 | 3.54 | 3.79 |
29 | 5.29 | 4.99 | 5.62 | 5.52 | 3.08 | 2.81 | 3.40 | 3.31 |
30 | 5.08 | 4.79 | 5.39 | 5.08 | 2.95 | 2.68 | 3.25 | 3.02 |
31 | 4.89 | 4.61 | 5.19 | 4.83 | 2.84 | 2.57 | 3.14 | 3.18 |
32 | 4.69 | 4.42 | 5.00 | 4.62 | 2.71 | 2.44 | 2.99 | 3.00 |
33 | 4.49 | 4.21 | 4.78 | 4.58 | 2.60 | 2.33 | 2.87 | 2.84 |
34 | 4.30 | 4.02 | 4.58 | 3.99 | 2.46 | 2.23 | 2.74 | 2.75 |
35 | 4.11 | 3.84 | 4.40 | 3.82 | 2.35 | 2.13 | 2.59 | 2.89 |
36 | 3.92 | 3.66 | 4.19 | 3.68 | 2.23 | 2.01 | 2.48 | 2.12 |
37 | 3.74 | 3.51 | 4.01 | 3.59 | 2.13 | 1.92 | 2.36 | 2.29 |
38 | 3.57 | 3.32 | 3.82 | 3.48 | 2.04 | 1.81 | 2.26 | 1.91 |
39 | 3.40 | 3.17 | 3.63 | 3.17 | 1.93 | 1.72 | 2.13 | 1.72 |
40 | 3.22 | 2.99 | 3.48 | 3.08 | 1.83 | 1.62 | 2.03 | 1.96 |
41 | 3.07 | 2.86 | 3.29 | 2.85 | 1.73 | 1.55 | 1.92 | 1.70 |
42 | 2.91 | 2.71 | 3.11 | 2.79 | 1.64 | 1.48 | 1.82 | 1.74 |
43 | 2.75 | 2.56 | 2.96 | 2.81 | 1.54 | 1.39 | 1.72 | 1.47 |
44 | 2.60 | 2.43 | 2.78 | 2.53 | 1.45 | 1.31 | 1.62 | 1.30 |
45 | 2.46 | 2.29 | 2.63 | 2.30 | 1.37 | 1.22 | 1.51 | 1.32 |
46 | 2.31 | 2.16 | 2.48 | 2.38 | 1.29 | 1.16 | 1.42 | 1.27 |
47 | 2.18 | 2.03 | 2.34 | 2.04 | 1.21 | 1.09 | 1.34 | 1.24 |
48 | 2.05 | 1.90 | 2.20 | 1.91 | 1.14 | 1.02 | 1.26 | 1.17 |
49 | 1.93 | 1.80 | 2.07 | 1.92 | 1.07 | 0.96 | 1.17 | 1.12 |
50 | 1.81 | 1.67 | 1.94 | 1.75 | 0.99 | 0.90 | 1.10 | 1.05 |
51 | 1.69 | 1.56 | 1.84 | 1.64 | 0.93 | 0.83 | 1.02 | 1.09 |
52 | 1.59 | 1.45 | 1.73 | 1.54 | 0.87 | 0.77 | 0.96 | 0.90 |
53 | 1.48 | 1.35 | 1.63 | 1.58 | 0.81 | 0.72 | 0.89 | 0.88 |
54 | 1.39 | 1.24 | 1.54 | 1.42 | 0.75 | 0.66 | 0.83 | 0.81 |
55 | 1.30 | 1.14 | 1.44 | 1.38 | 0.69 | 0.61 | 0.78 | 0.67 |
56 | 1.20 | 1.05 | 1.37 | 1.22 | 0.64 | 0.56 | 0.73 | 0.66 |
57 | 1.12 | 0.97 | 1.29 | 1.16 | 0.59 | 0.51 | 0.68 | 0.52 |
58 | 1.04 | 0.87 | 1.21 | 1.01 | 0.54 | 0.46 | 0.64 | 0.51 |
59 | 0.96 | 0.81 | 1.15 | 0.80 | 0.50 | 0.41 | 0.60 | 0.44 |
60 | 0.90 | 0.73 | 1.08 | 0.85 | 0.46 | 0.37 | 0.56 | 0.39 |
61 | 0.83 | 0.65 | 1.03 | 0.75 | 0.42 | 0.33 | 0.53 | 0.35 |
62 | 0.76 | 0.58 | 0.96 | 0.67 | 0.38 | 0.30 | 0.49 | 0.28 |
63 | 0.71 | 0.52 | 0.91 | 0.61 | 0.35 | 0.26 | 0.46 | 0.25 |
64 | 0.65 | 0.47 | 0.88 | 0.50 | 0.31 | 0.23 | 0.43 | 0.29 |
65 | 0.60 | 0.41 | 0.83 | 0.51 | 0.29 | 0.20 | 0.39 | 0.25 |
Panel D: 4010 - Essential hypertension | ||||||||
18 | 0.89 | 0.61 | 1.22 | 0.89 | 0.44 | 0.28 | 0.63 | 0.99 |
19 | 1.00 | 0.71 | 1.34 | 1.16 | 0.50 | 0.33 | 0.71 | 1.13 |
20 | 1.13 | 0.81 | 1.46 | 0.95 | 0.57 | 0.39 | 0.79 | 0.65 |
21 | 1.27 | 0.93 | 1.61 | 1.17 | 0.64 | 0.45 | 0.85 | 0.45 |
22 | 1.41 | 1.07 | 1.75 | 1.25 | 0.72 | 0.52 | 0.94 | 0.47 |
23 | 1.57 | 1.22 | 1.92 | 1.46 | 0.81 | 0.60 | 1.03 | 0.44 |
24 | 1.72 | 1.39 | 2.10 | 1.56 | 0.90 | 0.69 | 1.14 | 0.75 |
25 | 1.92 | 1.56 | 2.27 | 1.68 | 1.01 | 0.79 | 1.25 | 1.09 |
26 | 2.10 | 1.76 | 2.47 | 1.80 | 1.11 | 0.90 | 1.35 | 1.04 |
27 | 2.30 | 1.97 | 2.66 | 2.26 | 1.23 | 1.03 | 1.47 | 1.15 |
28 | 2.53 | 2.19 | 2.86 | 2.25 | 1.36 | 1.12 | 1.59 | 1.70 |
29 | 2.74 | 2.41 | 3.09 | 2.58 | 1.49 | 1.26 | 1.74 | 1.99 |
30 | 2.98 | 2.65 | 3.32 | 3.18 | 1.64 | 1.40 | 1.88 | 2.12 |
31 | 3.22 | 2.93 | 3.56 | 3.11 | 1.78 | 1.56 | 2.02 | 2.11 |
32 | 3.49 | 3.18 | 3.77 | 3.38 | 1.96 | 1.71 | 2.19 | 2.15 |
33 | 3.75 | 3.46 | 4.06 | 3.83 | 2.12 | 1.88 | 2.36 | 2.89 |
34 | 4.03 | 3.76 | 4.31 | 4.01 | 2.29 | 2.06 | 2.56 | 2.97 |
35 | 4.32 | 4.04 | 4.60 | 4.29 | 2.48 | 2.25 | 2.74 | 3.29 |
36 | 4.61 | 4.35 | 4.88 | 4.77 | 2.68 | 2.44 | 2.93 | 3.00 |
37 | 4.91 | 4.63 | 5.18 | 5.32 | 2.89 | 2.63 | 3.13 | 3.28 |
38 | 5.22 | 4.95 | 5.49 | 5.05 | 3.08 | 2.84 | 3.34 | 3.83 |
39 | 5.53 | 5.25 | 5.82 | 5.63 | 3.31 | 3.06 | 3.57 | 3.72 |
40 | 5.85 | 5.56 | 6.14 | 6.10 | 3.53 | 3.27 | 3.80 | 3.85 |
41 | 6.18 | 5.92 | 6.49 | 6.33 | 3.75 | 3.50 | 4.02 | 4.03 |
42 | 6.51 | 6.22 | 6.84 | 6.52 | 4.00 | 3.72 | 4.26 | 4.51 |
43 | 6.85 | 6.56 | 7.19 | 6.95 | 4.23 | 3.95 | 4.52 | 4.69 |
44 | 7.18 | 6.87 | 7.55 | 7.31 | 4.48 | 4.19 | 4.76 | 4.90 |
45 | 7.53 | 7.20 | 7.90 | 7.30 | 4.71 | 4.42 | 5.00 | 5.12 |
46 | 7.87 | 7.54 | 8.23 | 7.90 | 4.95 | 4.67 | 5.26 | 5.01 |
47 | 8.21 | 7.88 | 8.59 | 8.30 | 5.21 | 4.93 | 5.49 | 5.49 |
48 | 8.56 | 8.22 | 8.93 | 8.38 | 5.47 | 5.17 | 5.76 | 5.86 |
49 | 8.90 | 8.57 | 9.28 | 8.75 | 5.72 | 5.42 | 6.01 | 5.76 |
50 | 9.25 | 8.90 | 9.62 | 9.24 | 5.97 | 5.68 | 6.28 | 6.07 |
51 | 9.58 | 9.23 | 9.99 | 9.88 | 6.23 | 5.92 | 6.53 | 6.49 |
52 | 9.93 | 9.55 | 10.32 | 10.09 | 6.47 | 6.17 | 6.75 | 6.80 |
53 | 10.27 | 9.92 | 10.65 | 10.70 | 6.71 | 6.44 | 7.00 | 6.76 |
54 | 10.61 | 10.22 | 11.01 | 10.71 | 6.95 | 6.68 | 7.23 | 7.03 |
55 | 10.93 | 10.53 | 11.36 | 11.22 | 7.19 | 6.91 | 7.47 | 6.96 |
56 | 11.25 | 10.83 | 11.68 | 11.49 | 7.41 | 7.15 | 7.71 | 7.19 |
57 | 11.56 | 11.12 | 12.03 | 12.33 | 7.63 | 7.35 | 7.92 | 7.65 |
58 | 11.88 | 11.40 | 12.37 | 12.64 | 7.84 | 7.56 | 8.14 | 7.71 |
59 | 12.17 | 11.65 | 12.76 | 12.54 | 8.04 | 7.73 | 8.36 | 8.15 |
60 | 12.48 | 11.90 | 13.11 | 12.86 | 8.24 | 7.87 | 8.60 | 7.88 |
61 | 12.79 | 12.12 | 13.47 | 13.42 | 8.41 | 8.02 | 8.81 | 8.55 |
62 | 13.07 | 12.31 | 13.83 | 14.82 | 8.58 | 8.11 | 9.06 | 8.99 |
63 | 13.33 | 12.46 | 14.26 | 15.59 | 8.73 | 8.19 | 9.24 | 8.90 |
64 | 13.60 | 12.64 | 14.63 | 15.89 | 8.85 | 8.23 | 9.50 | 8.90 |
65 | 13.85 | 12.78 | 15.00 | 14.94 | 8.97 | 8.32 | 9.70 | 8.89 |
Panel E: 0440 - Asymptomatic HIV | ||||||||
18 | 0.54 | 0.36 | 0.79 | 0.44 | 0.20 | 0.12 | 0.33 | 0.79 |
19 | 0.58 | 0.40 | 0.81 | 0.45 | 0.22 | 0.14 | 0.34 | 0.21 |
20 | 0.62 | 0.45 | 0.84 | 0.56 | 0.24 | 0.16 | 0.35 | 0.24 |
21 | 0.66 | 0.49 | 0.87 | 0.73 | 0.25 | 0.17 | 0.37 | 0.26 |
22 | 0.69 | 0.53 | 0.89 | 0.63 | 0.27 | 0.19 | 0.38 | 0.22 |
23 | 0.73 | 0.58 | 0.92 | 0.84 | 0.28 | 0.21 | 0.38 | 0.19 |
24 | 0.76 | 0.63 | 0.94 | 0.84 | 0.30 | 0.22 | 0.40 | 0.21 |
25 | 0.80 | 0.67 | 0.97 | 0.81 | 0.31 | 0.24 | 0.41 | 0.32 |
26 | 0.83 | 0.71 | 0.99 | 0.87 | 0.32 | 0.25 | 0.42 | 0.19 |
27 | 0.86 | 0.74 | 1.01 | 0.77 | 0.34 | 0.26 | 0.44 | 0.52 |
28 | 0.88 | 0.78 | 1.03 | 0.71 | 0.35 | 0.27 | 0.44 | 0.46 |
29 | 0.90 | 0.80 | 1.04 | 0.86 | 0.36 | 0.29 | 0.45 | 0.37 |
30 | 0.92 | 0.82 | 1.05 | 0.88 | 0.37 | 0.30 | 0.46 | 0.45 |
31 | 0.94 | 0.84 | 1.06 | 0.90 | 0.38 | 0.30 | 0.46 | 0.38 |
32 | 0.95 | 0.86 | 1.08 | 0.86 | 0.38 | 0.31 | 0.46 | 0.44 |
33 | 0.96 | 0.87 | 1.07 | 0.91 | 0.39 | 0.31 | 0.47 | 0.34 |
34 | 0.96 | 0.86 | 1.08 | 0.79 | 0.39 | 0.32 | 0.48 | 0.23 |
35 | 0.96 | 0.87 | 1.08 | 0.90 | 0.39 | 0.32 | 0.47 | 0.32 |
36 | 0.96 | 0.86 | 1.08 | 0.93 | 0.39 | 0.32 | 0.48 | 0.41 |
37 | 0.95 | 0.85 | 1.07 | 0.73 | 0.39 | 0.32 | 0.47 | 0.37 |
38 | 0.94 | 0.84 | 1.05 | 0.85 | 0.39 | 0.32 | 0.47 | 0.26 |
39 | 0.92 | 0.82 | 1.04 | 0.90 | 0.38 | 0.31 | 0.46 | 0.30 |
40 | 0.91 | 0.81 | 1.01 | 0.92 | 0.37 | 0.31 | 0.45 | 0.35 |
41 | 0.88 | 0.78 | 0.99 | 0.90 | 0.37 | 0.30 | 0.44 | 0.37 |
42 | 0.86 | 0.76 | 0.96 | 0.94 | 0.35 | 0.29 | 0.43 | 0.31 |
43 | 0.83 | 0.73 | 0.93 | 0.91 | 0.34 | 0.28 | 0.41 | 0.45 |
44 | 0.80 | 0.71 | 0.89 | 0.90 | 0.33 | 0.28 | 0.40 | 0.39 |
45 | 0.77 | 0.68 | 0.86 | 1.02 | 0.32 | 0.26 | 0.38 | 0.42 |
46 | 0.73 | 0.65 | 0.82 | 0.88 | 0.30 | 0.25 | 0.36 | 0.34 |
47 | 0.70 | 0.62 | 0.78 | 0.89 | 0.29 | 0.24 | 0.35 | 0.26 |
48 | 0.66 | 0.59 | 0.74 | 0.69 | 0.27 | 0.23 | 0.32 | 0.27 |
49 | 0.62 | 0.55 | 0.70 | 0.67 | 0.26 | 0.22 | 0.31 | 0.21 |
50 | 0.58 | 0.52 | 0.66 | 0.62 | 0.24 | 0.20 | 0.28 | 0.26 |
51 | 0.55 | 0.48 | 0.62 | 0.55 | 0.22 | 0.19 | 0.27 | 0.21 |
52 | 0.51 | 0.44 | 0.58 | 0.49 | 0.21 | 0.17 | 0.25 | 0.17 |
53 | 0.47 | 0.40 | 0.54 | 0.55 | 0.19 | 0.16 | 0.23 | 0.21 |
54 | 0.43 | 0.37 | 0.50 | 0.44 | 0.18 | 0.14 | 0.21 | 0.13 |
55 | 0.40 | 0.33 | 0.47 | 0.30 | 0.16 | 0.13 | 0.20 | 0.09 |
56 | 0.36 | 0.30 | 0.44 | 0.32 | 0.15 | 0.12 | 0.18 | 0.12 |
57 | 0.33 | 0.26 | 0.41 | 0.36 | 0.13 | 0.10 | 0.17 | 0.13 |
58 | 0.30 | 0.23 | 0.38 | 0.24 | 0.12 | 0.09 | 0.15 | 0.10 |
59 | 0.27 | 0.20 | 0.35 | 0.26 | 0.10 | 0.07 | 0.14 | 0.08 |
60 | 0.24 | 0.17 | 0.32 | 0.16 | 0.09 | 0.07 | 0.13 | 0.04 |
61 | 0.21 | 0.15 | 0.30 | 0.19 | 0.08 | 0.05 | 0.11 | 0.06 |
62 | 0.19 | 0.12 | 0.27 | 0.08 | 0.07 | 0.04 | 0.10 | 0.05 |
63 | 0.17 | 0.10 | 0.25 | 0.13 | 0.06 | 0.04 | 0.09 | 0.06 |
64 | 0.15 | 0.09 | 0.23 | 0.08 | 0.05 | 0.03 | 0.08 | 0.06 |
65 | 0.13 | 0.07 | 0.21 | 0.10 | 0.04 | 0.02 | 0.07 | 0.05 |
Mental Disorders
The share of concurrent-claim applicants among all cases with a mental diagnosis is high (61.5%). This group represents a subset of much younger claimants in low socioeconomic status. Concurrent-claim applicants account for 84% of drug-related and 76% of alcohol-related substance addiction diagnoses in initial DI applications, resulting in posterior mean correlations of 0.23 and 0.20, respectively.15 The age-specific conditional probabilities of a drug addiction diagnosis appear in Panel B of Chart 5. Likewise, 78% and 74% of DI claimants diagnosed with personality disorders and with schizophrenia, respectively, apply concurrently. The conditional probabilities associated with the latter appear in Panel C of Chart 5. Although not shown in Table 5, concurrent-claim applicants account for 62% of affective/mood impairments, yielding a statistically significant posterior mean correlation of 0.08.
Nervous System and Sense Organ Diseases
As previously discussed, multiple impairments in the nervous system/sense organs category have high initial allowance rates. Among DI applicants with those impairments, the proportion that files concurrently is in most cases well below the overall average of 52.5%. For example, more than 80% of the diagnoses involving anterior horn cell disease and Parkinson's disease encompass DI-only claimants, resulting in posterior mean correlations of −0.26 and −0.21, respectively. When multiple sclerosis, muscular dystrophies, other diseases of the spinal cord, myoneural disorders, cerebral palsy, or carpal tunnel syndrome are diagnosed, concurrent-claim applicants compose less than 42% of cases. Of the disorders in this body system with statistically significant correlation estimates in Table 5, only carpal tunnel syndrome has a relatively low share of allowances. About 48.5% of all nervous system/sense organs diagnoses involve applicants who filed concurrently.
Other Disorders
The remaining correlations highlighted in Table 5 include two low allowance-rate diagnoses that are positively correlated with concurrent-application status (essential hypertension and asymptomatic HIV), with their respective age-specific conditional probabilities shown in Panels D and E of Chart 5. Of DI claimants with essential hypertension, 56% file concurrently; of those diagnosed with asymptomatic HIV, 80% do. Conversely, chronic liver disease/cirrhosis and symptomatic HIV are two disorders with positive correlation with concurrent-claim status, but higher allowance rates. Of DI claimants with chronic liver disease/cirrhosis, 60% file concurrently; of those with symptomatic HIV, 73% do. In addition, fractures of the vertebral column are less likely among concurrent applicants (−0.12 posterior mean correlation), although this is not the case for many of the other impairments classified as injuries. For instance, 57.5% of claims with the most common diagnosis in this body group (fractures of the lower limb) involve applicants filing concurrently, resulting in a low but positive and statistically significant mean estimated correlation of 0.02 with concurrent-claim status.
In summary, contributing to the substantial difference in initial allowance rates between DI-only and concurrent-claim applicants is their distinct diagnostic composition. Concurrent-claim filers account for lower proportions of cancers and impairments of the nervous system/sense organs. They also account for lower shares of the high allowance-rate circulatory impairments, but a greater share of essential hypertension. Among DI applicants, concurrent-claim filers represent the majority of mental impairment diagnoses, the minority of fractures of the vertebral column (but more of the other injuries associated with a low allowance rate), and a higher share of chronic liver disease/cirrhosis and HIV infection (both symptomatic and asymptomatic).
Variables Correlated with Reapplicant Status
An applicant whose claim is denied has the opportunity to appeal a decision within 60 days of notification and can always file a new initial application after the deadline. As discussed previously, one of the binary variables introduced in the model identifies whether a claimant had applied for DI benefits within the previous 10 years. I refer to these claimants as reapplicants; conversely, I refer to those applying to the program for the first time within a 10-year window as new applicants. Among those filing initial DI claims in 2009, 29.4% had previously applied to the program in or after 1999. Chart 6 shows the reapplicant share of all applicants, and the estimated probability of reapplicant status, by age. The reapplicant share is only about 20% for filers aged 21, peaks at about 37% for filers in their 40s, and dips back to 20% among those aged 60 or older. The median age of reapplicants is 47, while for new applicants it is 50. Table 6 presents correlation estimates among the binary variables in the model that are associated with reapplicant status. Given the overlap between reapplicant and concurrent-claim filers, it is not surprising to find many similarities between Tables 5 and 6.
Reapplicants as a percentage of all applicants filing initial claims for disability benefits, by age, 2009
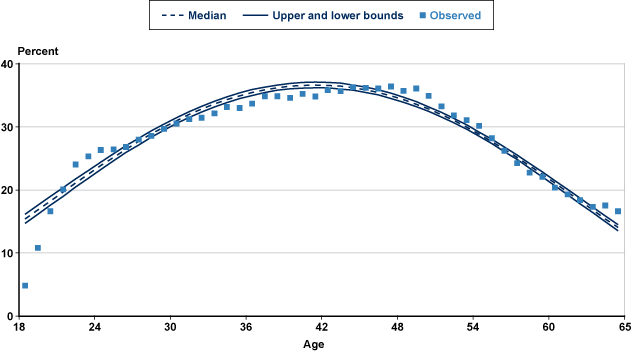
Age | Median | Lower bound | Upper bound | Observed |
---|---|---|---|---|
18 | 15.40 | 14.69 | 16.15 | 4.84 |
19 | 16.81 | 16.14 | 17.55 | 10.77 |
20 | 18.24 | 17.57 | 18.95 | 16.59 |
21 | 19.68 | 18.99 | 20.34 | 20.07 |
22 | 21.08 | 20.43 | 21.73 | 24.01 |
23 | 22.48 | 21.82 | 23.08 | 25.31 |
24 | 23.84 | 23.23 | 24.42 | 26.30 |
25 | 25.17 | 24.59 | 25.73 | 26.45 |
26 | 26.45 | 25.92 | 27.00 | 26.82 |
27 | 27.68 | 27.14 | 28.20 | 27.98 |
28 | 28.86 | 28.37 | 29.35 | 28.58 |
29 | 29.96 | 29.48 | 30.42 | 29.68 |
30 | 30.98 | 30.53 | 31.45 | 30.54 |
31 | 31.96 | 31.49 | 32.38 | 31.21 |
32 | 32.81 | 32.36 | 33.27 | 31.45 |
33 | 33.61 | 33.17 | 34.07 | 32.11 |
34 | 34.31 | 33.84 | 34.72 | 33.13 |
35 | 34.92 | 34.48 | 35.37 | 33.00 |
36 | 35.45 | 34.98 | 35.90 | 33.71 |
37 | 35.86 | 35.43 | 36.30 | 34.86 |
38 | 36.19 | 35.80 | 36.64 | 34.85 |
39 | 36.45 | 36.02 | 36.87 | 34.60 |
40 | 36.57 | 36.15 | 37.04 | 35.20 |
41 | 36.64 | 36.18 | 37.10 | 34.83 |
42 | 36.59 | 36.17 | 37.05 | 35.82 |
43 | 36.44 | 36.00 | 36.91 | 35.68 |
44 | 36.20 | 35.79 | 36.62 | 36.27 |
45 | 35.88 | 35.45 | 36.30 | 36.20 |
46 | 35.44 | 35.04 | 35.85 | 36.07 |
47 | 34.90 | 34.49 | 35.33 | 36.41 |
48 | 34.30 | 33.92 | 34.70 | 35.68 |
49 | 33.59 | 33.20 | 33.99 | 36.06 |
50 | 32.80 | 32.43 | 33.16 | 34.90 |
51 | 31.93 | 31.55 | 32.29 | 33.24 |
52 | 31.00 | 30.62 | 31.32 | 31.80 |
53 | 29.94 | 29.61 | 30.32 | 31.09 |
54 | 28.84 | 28.48 | 29.17 | 30.14 |
55 | 27.67 | 27.33 | 28.02 | 28.19 |
56 | 26.45 | 26.07 | 26.80 | 26.23 |
57 | 25.16 | 24.81 | 25.54 | 24.25 |
58 | 23.83 | 23.46 | 24.21 | 22.77 |
59 | 22.47 | 22.07 | 22.84 | 22.07 |
60 | 21.08 | 20.68 | 21.48 | 20.36 |
61 | 19.66 | 19.23 | 20.07 | 19.29 |
62 | 18.25 | 17.81 | 18.68 | 18.35 |
63 | 16.82 | 16.38 | 17.25 | 17.30 |
64 | 15.41 | 14.95 | 15.87 | 17.51 |
65 | 14.04 | 13.53 | 14.49 | 16.59 |
Reapplicants may represent an imperfect proxy for claimants at the margin of the definition of disability because on one hand, they were denied benefits in the past; but on the other hand, enough time may have elapsed for their health to potentially deteriorate further. It is not surprising that Table 6 shows many malignant neoplasms are negatively correlated with reapplicant status—both because cancers tend to have late onset ages while reapplicants tend to be younger, and because cancers have a very high likelihood of allowance for first-time applicants. Thus, the shares of reapplicants among claimants diagnosed with malignant cancers of the brain, ovaries, esophagus, and pancreas are less than 10%. Similarly, less than 15% of diagnoses of neoplasms of the stomach, colon, lung, kidney, liver, and uterus involve reapplicants.
Diagnostic group, impairment code, and diagnosis | Reapplicants a as a percentage of applicants | Correlation (posterior mean) | t-statistic | Numerical standard error |
---|---|---|---|---|
Neoplasms (malignant) | ||||
1910 - Brain cancer | 8.58 | -0.2583 | -12.70 | 0.001538 |
1510 - Stomach cancer | 11.43 | -0.2413 | -7.59 | 0.003225 |
1830 - Ovarian cancer | 9.95 | -0.2143 | -8.34 | 0.000939 |
1620 - Lung cancer | 11.08 | -0.1950 | -15.01 | 0.000603 |
1500 - Esophageal cancer | 8.93 | -0.1934 | -7.12 | 0.002455 |
1530 - Colon cancer | 13.42 | -0.1924 | -11.97 | 0.002473 |
1890 - Kidney cancer | 12.69 | -0.1833 | -6.71 | 0.002393 |
1550 - Liver cancer | 12.10 | -0.1711 | -8.79 | 0.001236 |
1570 - Pancreatic cancer | 8.96 | -0.1631 | -6.73 | 0.001895 |
2070 - Leukemias | 12.30 | -0.1592 | -7.59 | 0.001333 |
1950 - Soft tissue tumor of the head and neck | 15.01 | -0.1464 | -7.38 | 0.001291 |
2020 - Lymphoma | 16.08 | -0.1444 | -7.39 | 0.000998 |
2030 - Multiple myeloma | 10.95 | -0.1410 | -4.34 | 0.003882 |
1790 - Uterine cancer | 12.38 | -0.1310 | -5.67 | 0.001474 |
1740 - Breast cancer | 16.53 | -0.1284 | -11.16 | 0.000410 |
Mental disorders | ||||
3195 - Borderline intellectual functioning | 43.82 | 0.1252 | 9.69 | 0.000569 |
2950 - Schizophrenic and other psychotic disorders | 39.68 | 0.1157 | 12.39 | 0.000342 |
3010 - Personality disorders | 37.83 | 0.1066 | 10.48 | 0.000268 |
2960 - Affective and mood disorders | 35.00 | 0.1032 | 23.62 | 0.000071 |
Nervous system and sense organ diseases | ||||
3450 - Epilepsy | 41.81 | 0.1224 | 12.99 | 0.000259 |
3350 - Anterior horn cell disease | 6.35 | -0.2107 | -6.48 | 0.003345 |
3310 - Other cerebral degenerations | 19.00 | -0.1076 | -4.52 | 0.001699 |
Injuries | ||||
8060 - Fracture of vertebral column | 18.87 | -0.1392 | -6.37 | 0.001755 |
8540 - Intracranial (traumatic brain) injury | 19.72 | -0.1210 | -6.72 | 0.000786 |
Infectious and parasitic diseases | ||||
0440 - Asymptomatic HIV | 50.22 | 0.1476 | 9.14 | 0.000850 |
SOURCE: Author's calculations using DRF 100% extract (for descriptive statistics) and MVP estimation model fitted to a DRF 10% random sample (for correlation estimates). | ||||
NOTES: Correlation estimates are selected for inclusion on the basis of having absolute values for the posterior mean of at least 0.10 and, generally, a t-statistic greater than 2.00.
Data are for applicants who cleared step 1 of the disability determination process.
Diagnoses are arranged by estimated correlation value. Negative correlations are listed by absolute value.
Diagnoses identified as "other" may be defined in the context of impairments not shown in this table.
|
||||
a. Previous DI claim filed 1999–2008. |
Panel A of Chart 7 illustrates the age-specific conditional probabilities corresponding to lung cancer for new and reapplying initial claimants. For a new applicant aged 63, the probability of having a primary or secondary diagnosis involving a malignant neoplasm of the lungs is about 4%. For a reapplicant, however, the likelihood of this diagnosis at the same age is less than 2%. The posterior mean correlation estimate in this case is −0.20. Overall, reapplicants account for 13.6% of all applicants diagnosed with neoplasms (not shown).
Estimated incidence probabilities of selected diagnoses for new and reapplying initial disability-benefit claimants, by age, 2009
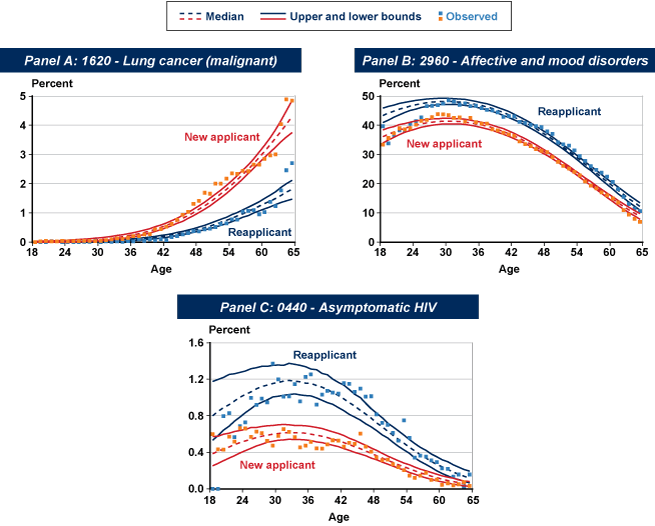
Age | Reapplicant | New Applicant | ||||||
---|---|---|---|---|---|---|---|---|
Median | Lower bound | Upper bound | Observed | Median | Lower bound | Upper bound | Observed | |
Panel A: 1620 - Lung cancer (malignant) | ||||||||
18 | 0.00 | 0.00 | 0.01 | 0.00 | 0.01 | 0.00 | 0.03 | 0.00 |
19 | 0.00 | 0.00 | 0.01 | 0.00 | 0.01 | 0.00 | 0.04 | 0.03 |
20 | 0.00 | 0.00 | 0.01 | 0.00 | 0.01 | 0.00 | 0.04 | 0.05 |
21 | 0.00 | 0.00 | 0.01 | 0.04 | 0.02 | 0.00 | 0.05 | 0.03 |
22 | 0.00 | 0.00 | 0.02 | 0.03 | 0.02 | 0.01 | 0.06 | 0.03 |
23 | 0.01 | 0.00 | 0.02 | 0.00 | 0.03 | 0.01 | 0.06 | 0.06 |
24 | 0.01 | 0.00 | 0.02 | 0.00 | 0.03 | 0.01 | 0.07 | 0.03 |
25 | 0.01 | 0.00 | 0.03 | 0.00 | 0.04 | 0.01 | 0.08 | 0.04 |
26 | 0.01 | 0.00 | 0.03 | 0.00 | 0.05 | 0.02 | 0.10 | 0.04 |
27 | 0.01 | 0.00 | 0.03 | 0.02 | 0.06 | 0.03 | 0.11 | 0.05 |
28 | 0.02 | 0.01 | 0.04 | 0.02 | 0.07 | 0.03 | 0.12 | 0.05 |
29 | 0.02 | 0.01 | 0.05 | 0.06 | 0.08 | 0.04 | 0.14 | 0.05 |
30 | 0.03 | 0.01 | 0.05 | 0.00 | 0.10 | 0.05 | 0.16 | 0.05 |
31 | 0.03 | 0.02 | 0.06 | 0.03 | 0.11 | 0.07 | 0.18 | 0.07 |
32 | 0.04 | 0.02 | 0.07 | 0.01 | 0.14 | 0.09 | 0.20 | 0.10 |
33 | 0.05 | 0.03 | 0.08 | 0.03 | 0.16 | 0.11 | 0.23 | 0.13 |
34 | 0.06 | 0.04 | 0.09 | 0.01 | 0.19 | 0.13 | 0.26 | 0.11 |
35 | 0.07 | 0.05 | 0.11 | 0.04 | 0.22 | 0.16 | 0.29 | 0.15 |
36 | 0.09 | 0.06 | 0.12 | 0.02 | 0.25 | 0.19 | 0.33 | 0.21 |
37 | 0.10 | 0.07 | 0.14 | 0.10 | 0.30 | 0.23 | 0.37 | 0.21 |
38 | 0.12 | 0.09 | 0.16 | 0.05 | 0.34 | 0.27 | 0.42 | 0.27 |
39 | 0.14 | 0.10 | 0.18 | 0.09 | 0.39 | 0.32 | 0.47 | 0.28 |
40 | 0.16 | 0.12 | 0.20 | 0.06 | 0.45 | 0.37 | 0.53 | 0.44 |
41 | 0.19 | 0.14 | 0.23 | 0.11 | 0.51 | 0.43 | 0.59 | 0.46 |
42 | 0.21 | 0.17 | 0.26 | 0.07 | 0.58 | 0.50 | 0.66 | 0.53 |
43 | 0.24 | 0.20 | 0.29 | 0.18 | 0.65 | 0.57 | 0.74 | 0.63 |
44 | 0.28 | 0.23 | 0.33 | 0.22 | 0.73 | 0.64 | 0.82 | 0.76 |
45 | 0.32 | 0.26 | 0.37 | 0.27 | 0.82 | 0.72 | 0.92 | 0.93 |
46 | 0.36 | 0.30 | 0.42 | 0.32 | 0.91 | 0.82 | 1.02 | 1.04 |
47 | 0.40 | 0.33 | 0.47 | 0.39 | 1.01 | 0.92 | 1.13 | 1.32 |
48 | 0.45 | 0.38 | 0.53 | 0.38 | 1.13 | 1.02 | 1.25 | 1.42 |
49 | 0.50 | 0.43 | 0.58 | 0.44 | 1.25 | 1.13 | 1.37 | 1.70 |
50 | 0.56 | 0.47 | 0.65 | 0.47 | 1.38 | 1.25 | 1.51 | 1.66 |
51 | 0.62 | 0.53 | 0.72 | 0.52 | 1.51 | 1.39 | 1.64 | 2.00 |
52 | 0.68 | 0.58 | 0.78 | 0.62 | 1.66 | 1.52 | 1.79 | 2.01 |
53 | 0.75 | 0.64 | 0.86 | 0.65 | 1.81 | 1.67 | 1.95 | 2.18 |
54 | 0.82 | 0.71 | 0.94 | 0.79 | 1.97 | 1.83 | 2.11 | 2.36 |
55 | 0.90 | 0.78 | 1.02 | 0.78 | 2.14 | 2.00 | 2.28 | 2.33 |
56 | 0.98 | 0.85 | 1.11 | 1.05 | 2.32 | 2.17 | 2.46 | 2.45 |
57 | 1.06 | 0.92 | 1.20 | 1.07 | 2.50 | 2.36 | 2.65 | 2.43 |
58 | 1.14 | 1.00 | 1.29 | 1.10 | 2.70 | 2.54 | 2.85 | 2.66 |
59 | 1.23 | 1.07 | 1.39 | 0.95 | 2.90 | 2.72 | 3.08 | 2.67 |
60 | 1.32 | 1.15 | 1.50 | 1.03 | 3.11 | 2.90 | 3.32 | 2.86 |
61 | 1.41 | 1.22 | 1.62 | 1.38 | 3.34 | 3.09 | 3.58 | 2.97 |
62 | 1.51 | 1.29 | 1.73 | 1.23 | 3.57 | 3.26 | 3.86 | 3.00 |
63 | 1.61 | 1.36 | 1.88 | 1.78 | 3.80 | 3.44 | 4.17 | 4.04 |
64 | 1.71 | 1.42 | 2.01 | 2.46 | 4.04 | 3.60 | 4.50 | 4.89 |
65 | 1.81 | 1.47 | 2.12 | 2.70 | 4.31 | 3.76 | 4.86 | 4.85 |
Panel B: 2960 - Affective and mood disorders | ||||||||
18 | 43.42 | 41.00 | 45.91 | 39.71 | 36.14 | 34.01 | 38.40 | 33.36 |
19 | 44.15 | 41.83 | 46.55 | 33.89 | 36.99 | 35.03 | 39.09 | 35.65 |
20 | 44.86 | 42.85 | 46.94 | 36.70 | 37.78 | 35.95 | 39.62 | 37.29 |
21 | 45.56 | 43.64 | 47.49 | 38.34 | 38.45 | 36.83 | 40.26 | 39.03 |
22 | 46.08 | 44.43 | 47.90 | 38.38 | 39.08 | 37.62 | 40.65 | 38.65 |
23 | 46.62 | 45.08 | 48.25 | 40.55 | 39.61 | 38.25 | 41.13 | 39.01 |
24 | 47.08 | 45.62 | 48.56 | 41.46 | 40.10 | 38.85 | 41.51 | 40.31 |
25 | 47.45 | 46.10 | 48.75 | 42.65 | 40.54 | 39.32 | 41.80 | 41.50 |
26 | 47.72 | 46.46 | 48.99 | 46.58 | 40.82 | 39.73 | 42.02 | 41.86 |
27 | 47.94 | 46.78 | 49.15 | 46.78 | 41.10 | 40.10 | 42.19 | 42.78 |
28 | 48.07 | 47.02 | 49.22 | 46.99 | 41.30 | 40.30 | 42.27 | 43.81 |
29 | 48.18 | 47.14 | 49.30 | 47.47 | 41.40 | 40.48 | 42.46 | 43.78 |
30 | 48.21 | 47.20 | 49.23 | 48.53 | 41.44 | 40.55 | 42.43 | 43.48 |
31 | 48.13 | 47.18 | 49.12 | 48.11 | 41.41 | 40.57 | 42.36 | 42.63 |
32 | 48.03 | 47.01 | 49.01 | 47.04 | 41.31 | 40.47 | 42.21 | 42.73 |
33 | 47.83 | 46.85 | 48.85 | 47.48 | 41.15 | 40.27 | 42.09 | 41.31 |
34 | 47.55 | 46.59 | 48.55 | 46.65 | 40.89 | 40.02 | 41.79 | 42.55 |
35 | 47.20 | 46.23 | 48.18 | 46.67 | 40.57 | 39.69 | 41.49 | 41.05 |
36 | 46.82 | 45.83 | 47.80 | 45.95 | 40.18 | 39.30 | 41.10 | 40.59 |
37 | 46.28 | 45.31 | 47.38 | 45.29 | 39.73 | 38.81 | 40.66 | 40.60 |
38 | 45.76 | 44.76 | 46.82 | 45.14 | 39.20 | 38.30 | 40.06 | 38.69 |
39 | 45.10 | 44.15 | 46.17 | 44.44 | 38.58 | 37.65 | 39.47 | 38.35 |
40 | 44.43 | 43.43 | 45.50 | 42.89 | 37.93 | 36.99 | 38.88 | 37.50 |
41 | 43.66 | 42.68 | 44.72 | 43.10 | 37.16 | 36.28 | 38.08 | 36.90 |
42 | 42.82 | 41.86 | 43.85 | 43.01 | 36.41 | 35.51 | 37.24 | 36.43 |
43 | 41.94 | 41.06 | 42.93 | 41.19 | 35.53 | 34.66 | 36.36 | 34.55 |
44 | 40.98 | 40.05 | 41.95 | 40.90 | 34.59 | 33.81 | 35.45 | 33.59 |
45 | 39.94 | 39.05 | 40.89 | 40.12 | 33.64 | 32.83 | 34.41 | 32.88 |
46 | 38.90 | 37.94 | 39.77 | 39.67 | 32.60 | 31.86 | 33.38 | 31.91 |
47 | 37.70 | 36.84 | 38.57 | 39.36 | 31.50 | 30.77 | 32.24 | 31.33 |
48 | 36.50 | 35.67 | 37.33 | 38.03 | 30.36 | 29.72 | 31.03 | 30.84 |
49 | 35.20 | 34.47 | 36.03 | 37.05 | 29.17 | 28.59 | 29.80 | 29.10 |
50 | 33.91 | 33.18 | 34.62 | 34.24 | 27.94 | 27.36 | 28.56 | 27.53 |
51 | 32.54 | 31.85 | 33.33 | 33.56 | 26.68 | 26.13 | 27.33 | 26.13 |
52 | 31.13 | 30.42 | 31.90 | 33.00 | 25.39 | 24.89 | 25.99 | 25.22 |
53 | 29.73 | 29.03 | 30.43 | 31.45 | 24.08 | 23.56 | 24.65 | 24.09 |
54 | 28.28 | 27.53 | 28.98 | 29.43 | 22.75 | 22.20 | 23.36 | 22.89 |
55 | 26.77 | 25.97 | 27.51 | 27.89 | 21.40 | 20.83 | 22.02 | 20.85 |
56 | 25.26 | 24.51 | 26.07 | 26.04 | 20.05 | 19.49 | 20.71 | 19.42 |
57 | 23.77 | 22.90 | 24.69 | 24.76 | 18.71 | 18.05 | 19.39 | 18.42 |
58 | 22.25 | 21.27 | 23.17 | 23.81 | 17.37 | 16.70 | 18.13 | 17.05 |
59 | 20.71 | 19.82 | 21.71 | 22.43 | 16.04 | 15.33 | 16.83 | 16.08 |
60 | 19.24 | 18.21 | 20.28 | 20.60 | 14.73 | 13.96 | 15.57 | 15.00 |
61 | 17.76 | 16.62 | 18.85 | 18.23 | 13.48 | 12.63 | 14.31 | 13.58 |
62 | 16.32 | 15.23 | 17.54 | 15.60 | 12.22 | 11.36 | 13.15 | 11.00 |
63 | 14.93 | 13.71 | 16.15 | 13.06 | 11.06 | 10.14 | 11.99 | 9.49 |
64 | 13.57 | 12.39 | 14.81 | 11.51 | 9.93 | 9.02 | 10.88 | 8.04 |
65 | 12.26 | 11.00 | 13.51 | 10.65 | 8.85 | 7.90 | 9.82 | 6.97 |
Panel C: 0440 - Asymptomatic HIV | ||||||||
18 | 0.80 | 0.53 | 1.17 | 0.00 | 0.38 | 0.25 | 0.57 | 0.60 |
19 | 0.85 | 0.58 | 1.20 | 0.00 | 0.41 | 0.28 | 0.58 | 0.43 |
20 | 0.89 | 0.64 | 1.22 | 0.80 | 0.44 | 0.31 | 0.60 | 0.43 |
21 | 0.92 | 0.69 | 1.25 | 0.83 | 0.46 | 0.34 | 0.61 | 0.57 |
22 | 0.96 | 0.74 | 1.26 | 0.57 | 0.48 | 0.36 | 0.63 | 0.52 |
23 | 1.00 | 0.79 | 1.27 | 0.69 | 0.50 | 0.39 | 0.64 | 0.66 |
24 | 1.03 | 0.83 | 1.29 | 0.73 | 0.53 | 0.42 | 0.65 | 0.66 |
25 | 1.06 | 0.88 | 1.32 | 1.00 | 0.54 | 0.44 | 0.67 | 0.57 |
26 | 1.09 | 0.92 | 1.33 | 0.92 | 0.56 | 0.47 | 0.67 | 0.63 |
27 | 1.12 | 0.95 | 1.34 | 0.99 | 0.58 | 0.49 | 0.68 | 0.61 |
28 | 1.14 | 0.97 | 1.34 | 0.95 | 0.59 | 0.51 | 0.69 | 0.53 |
29 | 1.15 | 1.00 | 1.35 | 1.37 | 0.60 | 0.52 | 0.69 | 0.47 |
30 | 1.16 | 1.01 | 1.35 | 1.20 | 0.61 | 0.53 | 0.70 | 0.58 |
31 | 1.18 | 1.02 | 1.35 | 1.01 | 0.61 | 0.54 | 0.71 | 0.65 |
32 | 1.18 | 1.03 | 1.38 | 1.01 | 0.61 | 0.54 | 0.70 | 0.62 |
33 | 1.18 | 1.04 | 1.36 | 1.15 | 0.61 | 0.54 | 0.70 | 0.56 |
34 | 1.17 | 1.03 | 1.35 | 0.96 | 0.61 | 0.54 | 0.69 | 0.45 |
35 | 1.16 | 1.02 | 1.34 | 1.23 | 0.61 | 0.54 | 0.69 | 0.47 |
36 | 1.15 | 1.02 | 1.31 | 1.25 | 0.60 | 0.53 | 0.68 | 0.51 |
37 | 1.13 | 1.00 | 1.30 | 0.92 | 0.59 | 0.51 | 0.67 | 0.44 |
38 | 1.11 | 0.97 | 1.26 | 1.03 | 0.58 | 0.51 | 0.66 | 0.44 |
39 | 1.09 | 0.94 | 1.25 | 1.07 | 0.56 | 0.49 | 0.64 | 0.49 |
40 | 1.05 | 0.92 | 1.20 | 1.05 | 0.54 | 0.48 | 0.62 | 0.53 |
41 | 1.02 | 0.89 | 1.17 | 1.05 | 0.53 | 0.46 | 0.61 | 0.53 |
42 | 0.98 | 0.85 | 1.14 | 1.16 | 0.51 | 0.44 | 0.58 | 0.46 |
43 | 0.95 | 0.82 | 1.09 | 1.15 | 0.48 | 0.42 | 0.55 | 0.50 |
44 | 0.91 | 0.79 | 1.04 | 1.06 | 0.46 | 0.40 | 0.53 | 0.49 |
45 | 0.86 | 0.75 | 1.00 | 1.10 | 0.44 | 0.38 | 0.50 | 0.61 |
46 | 0.82 | 0.71 | 0.94 | 1.01 | 0.41 | 0.36 | 0.48 | 0.46 |
47 | 0.77 | 0.68 | 0.88 | 1.02 | 0.39 | 0.34 | 0.45 | 0.41 |
48 | 0.72 | 0.64 | 0.83 | 0.82 | 0.36 | 0.32 | 0.42 | 0.34 |
49 | 0.68 | 0.59 | 0.78 | 0.72 | 0.34 | 0.29 | 0.39 | 0.32 |
50 | 0.63 | 0.56 | 0.72 | 0.70 | 0.31 | 0.27 | 0.36 | 0.32 |
51 | 0.59 | 0.51 | 0.68 | 0.61 | 0.29 | 0.25 | 0.33 | 0.27 |
52 | 0.54 | 0.46 | 0.63 | 0.53 | 0.26 | 0.23 | 0.31 | 0.24 |
53 | 0.50 | 0.43 | 0.59 | 0.75 | 0.24 | 0.20 | 0.28 | 0.20 |
54 | 0.46 | 0.39 | 0.54 | 0.56 | 0.22 | 0.18 | 0.26 | 0.14 |
55 | 0.41 | 0.34 | 0.50 | 0.34 | 0.20 | 0.16 | 0.24 | 0.12 |
56 | 0.37 | 0.30 | 0.46 | 0.36 | 0.18 | 0.14 | 0.22 | 0.14 |
57 | 0.34 | 0.27 | 0.42 | 0.35 | 0.16 | 0.12 | 0.20 | 0.17 |
58 | 0.30 | 0.23 | 0.38 | 0.31 | 0.14 | 0.11 | 0.18 | 0.10 |
59 | 0.27 | 0.20 | 0.35 | 0.29 | 0.12 | 0.09 | 0.16 | 0.10 |
60 | 0.24 | 0.17 | 0.32 | 0.22 | 0.11 | 0.08 | 0.14 | 0.04 |
61 | 0.21 | 0.14 | 0.30 | 0.22 | 0.09 | 0.06 | 0.13 | 0.06 |
62 | 0.18 | 0.12 | 0.26 | 0.13 | 0.08 | 0.05 | 0.12 | 0.04 |
63 | 0.16 | 0.10 | 0.24 | 0.16 | 0.07 | 0.04 | 0.10 | 0.05 |
64 | 0.14 | 0.08 | 0.21 | 0.03 | 0.06 | 0.03 | 0.09 | 0.07 |
65 | 0.12 | 0.06 | 0.19 | 0.16 | 0.05 | 0.03 | 0.08 | 0.03 |
As with concurrent-claim applicants, claimants with a history of previous applications tend to have higher-than-average incidences of personality disorders, borderline intellectual functioning, schizophrenia, and affective/mood impairments. The conditional incidence probabilities associated with the latter diagnosis appear in Panel B of Chart 7. In addition, other diagnoses positively correlated with reapplicant status include epilepsy (0.12 posterior mean correlation) and asymptomatic HIV infections (0.14 mean correlation). For an asymptomatic HIV diagnosis, 50% of filers are reapplicants. Conditional incidence probabilities for this impairment appear in Panel C of Chart 7. The other diagnoses in Table 6 with low shares of reapplicants include intracranial injuries, fractures of the vertebral column, and two impairments in the nervous system/sense organs category (other cerebral degenerations and anterior horn cell disease).
Correlations Involving Neoplastic Impairments
The remaining sections in this paper discuss statistically significant correlations between diagnoses, beginning with neoplastic impairments. Many malignant cancer diagnoses have high positive correlations with one another. Metastasis is a possible factor. About 12.7% of claimants with a neoplastic primary diagnosis also have cancer as a secondary diagnosis (not shown).16 Further, 56.3% of cancers cited as a secondary diagnosis combine with another neoplasm. A malignant neoplasm is far more likely to be assigned as a primary impairment than as a secondary one; however, when a neoplasm is listed as a secondary diagnosis, the primary impairment in most cases is another cancer.
Table 7 shows 95 (of more than 100) statistically significant estimated correlations between malignant neoplasms. The highest mean correlation values (greater than 0.25) involve lung cancer with malignant neoplasms of the brain (0.42), liver (0.33), and kidney (0.27); breast cancer with ovarian (0.34) and uterine (0.32) cancers; colon cancer with liver cancer (0.47); liver cancer with cancers of the pancreas (0.45), stomach (0.32), esophagus (0.31), and kidneys (0.27); stomach cancer with cancer of the esophagus (0.35); and ovarian cancer with cancer of the uterus (0.40). Nineteen additional combinations of neoplastic disorders in Table 7 have posterior mean correlations exceeding 0.20.
Impairment code and diagnosis | Correlation (posterior mean) | t-statistic | Numerical standard error |
---|---|---|---|
1620 - Lung cancer and— | |||
1910 - Brain cancer | 0.4210 | 20.44 | 0.001438 |
1550 - Liver cancer | 0.3263 | 14.72 | 0.001727 |
1890 - Kidney cancer | 0.2656 | 8.20 | 0.003548 |
1790 - Uterine cancer | 0.2413 | 7.77 | 0.003125 |
1950 - Soft tissue tumor of the head and neck | 0.2318 | 7.70 | 0.002590 |
1570 - Pancreatic cancer | 0.2210 | 7.47 | 0.002200 |
1500 - Esophageal cancer | 0.2118 | 6.91 | 0.002047 |
1530 - Colon cancer | 0.2056 | 9.60 | 0.001529 |
1740 - Breast cancer | 0.1817 | 9.22 | 0.001261 |
1830 - Ovarian cancer | 0.1809 | 5.57 | 0.003401 |
1510 - Stomach cancer | 0.1742 | 4.10 | 0.004717 |
2070 - Leukemias | 0.1336 | 3.74 | 0.003933 |
2030 - Multiple myeloma | 0.1235 | 3.08 | 0.004317 |
1740 - Breast cancer and— | |||
1830 - Ovarian cancer | 0.3394 | 13.11 | 0.002107 |
1790 - Uterine cancer | 0.3153 | 9.50 | 0.003526 |
1550 - Liver cancer | 0.2006 | 6.78 | 0.002830 |
1910 - Brain cancer | 0.1913 | 6.77 | 0.002297 |
1570 - Pancreatic cancer | 0.1462 | 3.90 | 0.005141 |
1530 - Colon cancer | 0.1150 | 4.63 | 0.002243 |
1850 - Prostate cancer | -0.3121 | -6.16 | 0.007720 |
1530 - Colon cancer and— | |||
1550 - Liver cancer | 0.4766 | 20.74 | 0.002723 |
1510 - Stomach cancer | 0.2286 | 5.78 | 0.004662 |
1830 - Ovarian cancer | 0.2118 | 6.19 | 0.003682 |
1570 - Pancreatic cancer | 0.1802 | 5.30 | 0.002945 |
1500 - Esophageal cancer | 0.1702 | 4.40 | 0.004117 |
1790 - Uterine cancer | 0.1555 | 3.72 | 0.004876 |
2070 - Leukemias | 0.1423 | 4.02 | 0.003212 |
1910 - Brain cancer | 0.1382 | 4.30 | 0.002788 |
1890 - Kidney cancer | 0.1339 | 3.08 | 0.004981 |
1950 - Soft tissue tumor of the head and neck | 0.1045 | 2.62 | 0.004116 |
1850 - Prostate cancer and— | |||
1500 - Esophageal cancer | 0.1657 | 4.02 | 0.004971 |
1890 - Kidney cancer | 0.1494 | 3.95 | 0.005817 |
1950 - Soft tissue tumor of the head and neck | 0.1327 | 3.68 | 0.003533 |
1510 - Stomach cancer | 0.1184 | 2.37 | 0.006750 |
1830 - Ovarian cancer | -0.2803 | -4.84 | 0.009124 |
1790 - Uterine cancer | -0.2294 | -3.87 | 0.009271 |
1550 - Liver cancer and— | |||
1570 - Pancreatic cancer | 0.4494 | 15.82 | 0.003459 |
1510 - Stomach cancer | 0.3217 | 8.93 | 0.004040 |
1500 - Esophageal cancer | 0.3088 | 9.09 | 0.003238 |
1890 - Kidney cancer | 0.2709 | 6.84 | 0.004139 |
1910 - Brain cancer | 0.2214 | 6.91 | 0.003449 |
1830 - Ovarian cancer | 0.1989 | 4.93 | 0.004831 |
1950 - Soft tissue tumor of the head and neck | 0.1955 | 5.02 | 0.003985 |
2070 - Leukemias | 0.1664 | 3.60 | 0.005806 |
2030 - Multiple myeloma | 0.1474 | 3.41 | 0.005948 |
2020 - Lymphoma | 0.1378 | 3.46 | 0.004076 |
1850 - Prostate cancer | 0.1278 | 3.61 | 0.004114 |
1790 - Uterine cancer | 0.1260 | 2.93 | 0.005817 |
1500 - Esophageal cancer and— | |||
1510 - Stomach cancer | 0.3529 | 8.29 | 0.005778 |
1950 - Soft tissue tumor of the head and neck | 0.2429 | 6.23 | 0.003939 |
1910 - Brain cancer | 0.2206 | 5.61 | 0.004300 |
1570 - Pancreatic cancer | 0.2197 | 5.25 | 0.005175 |
1890 - Kidney cancer | 0.2109 | 4.53 | 0.005043 |
2070 - Leukemias | 0.1560 | 3.31 | 0.005759 |
2030 - Multiple myeloma | 0.1425 | 2.77 | 0.006648 |
1830 - Ovarian cancer | 0.1166 | 2.32 | 0.006150 |
2020 - Lymphoma | 0.1071 | 2.48 | 0.004751 |
1830 - Ovarian cancer and— | |||
1790 - Uterine cancer | 0.4007 | 10.19 | 0.004970 |
1510 - Stomach cancer | 0.2202 | 3.91 | 0.009115 |
1570 - Pancreatic cancer | 0.1904 | 4.05 | 0.006035 |
1910 - Brain cancer | 0.1579 | 3.17 | 0.007116 |
2030 - Multiple myeloma | 0.1449 | 2.39 | 0.009494 |
2070 - Leukemias | 0.1404 | 2.98 | 0.006634 |
1890 - Kidney cancer | 0.1105 | 2.56 | 0.005633 |
1910 - Brain cancer and— | |||
1570 - Pancreatic cancer | 0.2109 | 5.09 | 0.004710 |
1890 - Kidney cancer | 0.2103 | 4.80 | 0.005194 |
1950 - Soft tissue tumor of the head and neck | 0.1951 | 5.40 | 0.003545 |
1790 - Uterine cancer | 0.1918 | 4.08 | 0.006595 |
1510 - Stomach cancer | 0.1770 | 3.18 | 0.007158 |
2030 - Multiple myeloma | 0.1564 | 3.02 | 0.006878 |
2070 - Leukemias | 0.1536 | 3.60 | 0.004317 |
1510 - Stomach cancer and— | |||
1570 - Pancreatic cancer | 0.2090 | 3.82 | 0.007531 |
1950 - Soft tissue tumor of the head and neck | 0.2039 | 3.68 | 0.007717 |
1890 - Kidney cancer | 0.1444 | 2.47 | 0.007647 |
2030 - Multiple myeloma | 0.1260 | 2.14 | 0.007698 |
2020 - Lymphoma | 0.1225 | 2.25 | 0.006511 |
1790 - Uterine cancer | 0.1210 | 1.94 | 0.009892 |
2070 - Leukemias | 0.1195 | 2.05 | 0.007792 |
1570 - Pancreatic cancer and— | |||
1890 - Kidney cancer | 0.1946 | 4.38 | 0.005580 |
1950 - Soft tissue tumor of the head and neck | 0.1659 | 3.70 | 0.005486 |
2070 - Leukemias | 0.1533 | 3.07 | 0.005994 |
2030 - Multiple myeloma | 0.1483 | 2.90 | 0.006341 |
1790 - Uterine cancer | 0.1191 | 2.34 | 0.007193 |
2020 - Lymphoma | 0.1051 | 2.39 | 0.005420 |
1890 - Kidney cancer and— | |||
1950 - Soft tissue tumor of the head and neck | 0.1645 | 4.25 | 0.004067 |
1790 - Uterine cancer | 0.1411 | 3.20 | 0.005497 |
2070 - Leukemias | 0.1128 | 2.15 | 0.006981 |
2030 - Multiple myeloma | 0.1080 | 1.99 | 0.006710 |
2070 - Leukemias and— | |||
2030 - Multiple myeloma | 0.2099 | 4.37 | 0.006634 |
2020 - Lymphoma | 0.1848 | 5.10 | 0.003468 |
1950 - Soft tissue tumor of the head and neck | 0.1433 | 3.04 | 0.006110 |
2020 - Lymphoma and— | |||
1950 - Soft tissue tumor of the head and neck | 0.1232 | 2.95 | 0.004316 |
2030 - Multiple myeloma | 0.1130 | 2.00 | 0.007713 |
2030 - Multiple myeloma and— | |||
1950 - Soft tissue tumor of the head and neck | 0.1295 | 2.32 | 0.008152 |
1790 - Uterine cancer | 0.1082 | 2.12 | 0.006331 |
SOURCE: Author's calculations using MVP estimation model fitted to a DRF 10% random sample. | |||
NOTES: Correlation estimates are selected for inclusion on the basis of having absolute values for the posterior mean of at least 0.10 and, generally, a t-statistic greater than 2.00.
Data are for applicants who cleared step 1 of the disability determination process.
Diagnoses are arranged by estimated correlation value. Negative correlations are listed by absolute value.
|
The three negative correlations in Table 7 correspond to the combinations of neoplasms that are sex-specific. For instance, the estimated correlations of prostate cancer with ovarian and uterine cancers are −0.28 and −0.23, respectively. Likewise, cancers of the breast and the prostate show a negative mean correlation of −0.31. These magnitudes may seem small, considering that they involve diagnostic pairs that are impossible, at least in the first two instances (prostate with ovary and uterus). However, these estimates are shaped by the interaction with additional correlations involving sex. Specifically, recall from Table 4 that the posterior mean correlation between prostate cancer and being male is 0.53, while the mean correlation between being male and malignant neoplasm of the ovary is −0.48. Thus, the likelihood of a model prediction involving a male claimant with, say, both prostate and ovarian cancer (or prostate and uterine cancer) is far more negligible than the correlation estimate between the two neoplasms might suggest.
Brain Cancers
Frequently, diseases that affect one organ have secondary effects on others. Among the cancers, malignant and benign neoplasms of the brain seem to have the greatest potential association with impairments of other diagnostic groups. Table 8 spotlights an interesting and noteworthy cluster of diagnoses from five different diagnostic groups that are positively correlated with one another. The diagnostic categories are neoplasms, nervous system/sense organ diseases, mental disorders, injuries, and circulatory system diseases. Benign brain cancers have positive correlations ranging from 0.12 to 0.25 with impairments in the nervous system/sense organs, including migraine, epilepsy, late effects of injuries to the nervous system, other cerebral degenerations, vertiginous syndromes, visual disturbances, other disorders of the nervous system, and blindness/low vision. Similarly, malignant neoplasms of the brain are associated with four nervous system/sense organ diagnoses, where the highest posterior mean correlation is with other diseases of the spinal cord (0.18). Overall, 24.4% of the diagnoses involving a benign neoplasm of the brain combine with a disorder of the nervous system/sense organs.
Diagnostic group, impairment code, and diagnosis | Correlation (posterior mean) | t-statistic | Numerical standard error |
---|---|---|---|
2250 - Benign brain cancer and— | |||
Nervous system and sense organ diseases | |||
3460 - Migraine | 0.2477 | 6.20 | 0.004112 |
3450 - Epilepsy | 0.2233 | 6.57 | 0.003609 |
9070 - Late effects of injuries to the nervous system | 0.2209 | 5.29 | 0.004739 |
3310 - Other cerebral degenerations | 0.2057 | 3.36 | 0.008441 |
3860 - Vertiginous syndromes | 0.1760 | 3.13 | 0.008437 |
3680 - Visual disturbances | 0.1301 | 2.86 | 0.005674 |
3490 - Other nervous system disorders | 0.1233 | 2.83 | 0.004972 |
3690 - Blindness and low vision | 0.1233 | 3.02 | 0.003798 |
Mental disorders | |||
2940 - Organic mental disorders | 0.1827 | 6.04 | 0.003327 |
Injuries | |||
8540 - Intracranial (traumatic brain) injury | 0.1465 | 3.17 | 0.005488 |
Circulatory system diseases | |||
4380 - Late effects of cerebrovascular disease | 0.1433 | 3.45 | 0.004358 |
1910 - Malignant brain cancer and— | |||
Nervous system and sense organ diseases | |||
3360 - Other spinal cord disorders | 0.1788 | 3.76 | 0.005626 |
3310 - Other cerebral degenerations | 0.1677 | 3.98 | 0.005108 |
9070 - Late effects of injuries to the nervous system | 0.1407 | 3.50 | 0.004348 |
3450 - Epilepsy | 0.1287 | 4.64 | 0.002523 |
Injuries | |||
8540 - Intracranial (traumatic brain) injury | 0.1581 | 3.41 | 0.006035 |
Circulatory system diseases | |||
4380 - Late effects of cerebrovascular disease | 0.1012 | 3.70 | 0.002582 |
SOURCE: Author's calculations using MVP estimation model fitted to a DRF 10% random sample. | |||
NOTES: Correlation estimates are selected for inclusion on the basis of having absolute values for the posterior mean of at least 0.10 and, generally, a t-statistic greater than 2.00.
Data are for applicants who cleared step 1 of the disability determination process.
Diagnoses are arranged by estimated correlation value.
Diagnoses identified as "other" may be defined in the context of impairments not shown in this table.
|
Benign neoplasms of the brain have a mean correlation of 0.18 with organic mental disorders. The latter diagnosis involves diminished mental capacity that is not the result of an underlying psychiatric diagnosis, but is due to an external factor (for example, brain damage suffered because of a heart attack, blunt trauma to the head, or lead exposure). Thus, the potential for a brain tumor to interfere with normal cognitive functions explains its association with an organic mental disorder diagnosis. Although not shown in Table 8, malignant neoplasms of the brain are also positively correlated with an organic mental impairment, with an estimated posterior mean of 0.10 and a t-statistic of 3.90.
Finally, both benign and malignant neoplasms of the brain are positively associated with intracranial injury and with late effects of cerebrovascular disease. The latter diagnosis represents the long-term consequences of conditions that affect the circulation of blood to the brain. Hence, to the extent that a brain tumor impedes normal blood flow, the relationship between these diagnoses is self-evident.
Cancer and Mental Impairments
Although organic mental disorders are positively associated with brain cancer, the estimated correlations in Table 9 suggest that the single most common mental diagnosis (affective/mood disorders) is negatively correlated with 15 types of neoplasms. These include neoplasms of the brain (both malignant and benign), liver, lungs, esophagus, pancreas, head/neck, kidneys, uterus, and ovaries. Overall, about 4.8% of primary and secondary neoplastic impairments combine with an affective/mood disorder.
Impairment code and diagnosis | Correlation (posterior mean) | t-statistic | Numerical standard error |
---|---|---|---|
2960 - Affective and mood disorders and— | |||
1910 - Brain cancer | -0.1889 | -8.26 | 0.001672 |
1550 - Liver cancer | -0.1685 | -7.75 | 0.002159 |
1620 - Lung cancer | -0.1525 | -10.62 | 0.000919 |
1500 - Esophageal cancer | -0.1421 | -5.12 | 0.002425 |
1570 - Pancreatic cancer | -0.1310 | -4.80 | 0.002029 |
1530 - Colon cancer | -0.1299 | -8.18 | 0.000978 |
1510 - Stomach cancer | -0.1280 | -3.85 | 0.003701 |
2030 - Multiple myeloma | -0.1269 | -4.01 | 0.002916 |
2020 - Lymphoma | -0.1243 | -6.66 | 0.000897 |
1950 - Soft tissue tumor of the head and neck | -0.1229 | -5.67 | 0.001677 |
1890 - Kidney cancer | -0.1209 | -4.87 | 0.001971 |
2250 - Brain cancer (benign) | -0.1207 | -4.35 | 0.002146 |
1790 - Uterine cancer | -0.1130 | -3.95 | 0.002984 |
2070 - Leukemias | -0.1127 | -5.35 | 0.001386 |
1830 - Ovarian cancer | -0.1028 | -3.98 | 0.002067 |
2950 - Schizophrenic and other psychotic disorders and— | |||
1550 - Liver cancer | 0.1943 | 5.41 | 0.003349 |
1500 - Esophageal cancer | 0.1927 | 4.01 | 0.006466 |
1620 - Lung cancer | 0.1825 | 7.58 | 0.001841 |
1570 - Pancreatic cancer | 0.1753 | 4.53 | 0.004962 |
1950 - Soft tissue tumor of the head and neck | 0.1390 | 3.78 | 0.003737 |
3180 - Intellectual disability and— | |||
1620 - Lung cancer | 0.2181 | 8.25 | 0.002187 |
1550 - Liver cancer | 0.2173 | 6.08 | 0.003985 |
1570 - Pancreatic cancer | 0.1973 | 4.75 | 0.004997 |
1500 - Esophageal cancer | 0.1738 | 3.64 | 0.006628 |
1910 - Brain cancer | 0.1551 | 4.50 | 0.003289 |
1950 - Soft tissue tumor of the head and neck | 0.1509 | 4.03 | 0.004334 |
1530 - Colon cancer | 0.1217 | 3.98 | 0.002545 |
2990 - Autistic disorders and— | |||
1620 - Lung cancer | 0.1556 | 3.88 | 0.004759 |
1550 - Liver cancer | 0.1518 | 3.18 | 0.006150 |
1500 - Esophageal cancer | 0.1408 | 2.39 | 0.008139 |
1950 - Soft tissue tumor of the head and neck | 0.1358 | 2.21 | 0.008951 |
1910 - Brain cancer | 0.1305 | 2.76 | 0.005783 |
SOURCE: Author's calculations using MVP estimation model fitted to a DRF 10% random sample. | |||
NOTES: Correlation estimates are selected for inclusion on the basis of having absolute values for the posterior mean of at least 0.10 and, generally, a t-statistic greater than 2.00.
Data are for applicants who cleared step 1 of the disability determination process.
Diagnoses are arranged by estimated correlation value. Negative correlations are listed by absolute value.
Cancers are malignant unless otherwise noted.
|
Table 9 also reports positive correlation estimates of various neoplasms with schizophrenia, intellectual disability, and autism. The association between schizophrenia and cancer is considered somewhat paradoxical in epidemiology (Hodgson, Wildgust, and Bushe 2010). Life expectancy is substantially lower for people with schizophrenia than it is for the general population, which cannot be fully attributed to the higher risk of suicide among the former. Earlier studies tended to report a lower incidence of schizophrenia with lung cancer, which is quite puzzling, given that tobacco-smoking rates tend to be much higher among those diagnosed with schizophrenia.
Schizophrenics are also more susceptible to engage in other behaviors that represent higher risk factors for cancer, such as increased alcohol consumption and failure to maintain a balanced diet or exercise. Some researchers have speculated about the possibility of schizophrenia conveying protection against neoplastic diseases based on both genetic factors and the anti-tumor properties of certain antipsychotic medications. More recently, studies have found either similar or higher incidence of neoplastic impairments among schizophrenics, after accounting for a number of confounding factors. To complicate matters further, cancer is more likely to go undiagnosed in people with serious mental illness than in the general population.17
According to Kao and others (2010), autism is associated with a higher frequency of genetic aberrations, including chromosomal rearrangements, suggesting a path to positive correlation with neoplastic impairments. Using data from the Department of Education on autism and from the Centers for Disease Control and Prevention on cancer, the authors find significant state-level correlation between the incidence rates of autism and breast cancer. Autism, intellectual disability, and schizophrenia are believed to have some of the strongest genetic causal contributions among all psychiatric diseases. Recent evidence suggests that autism and schizophrenia are genetically linked, sharing similar chromosomal abnormalities (Spek and Wouters 2010). In addition, there is evidence of diagnostic substitution in medical practice, where previous cases diagnosed as intellectual or learning disabilities may have actually been cases of autism.
Cancer, Kidney Disease, and HIV Infection
Table 10 shows that 13 malignant neoplasms have statistically significant positive correlations with chronic renal failure (in the genitourinary system group). According to Levey and others (2007), chronic kidney disease is associated with infections, cardiovascular disease, and cancer. Specific cancers such as multiple myeloma are known to cause renal disease; although diminished kidney function can be a late effect of cancer treatment, kidney function in cancer patients is evaluated prior to treatment to determine the toxicity and dosage of chemotherapy. The development of chronic kidney disease in conjunction with or subsequent to cancer is also well documented. For instance, one study of Medicare patients cited by Levey and others estimates a 50% higher prevalence of cancer in subjects starting dialysis than that for a matched group of nondialysis patients.
Impairment code and diagnosis | Correlation (posterior mean) | t-statistic | Numerical standard error |
---|---|---|---|
5850 - Chronic renal failure and— | |||
2030 - Multiple myeloma | 0.2305 | 6.06 | 0.004201 |
1890 - Kidney cancer | 0.1900 | 5.18 | 0.004661 |
1500 - Esophageal cancer | 0.1885 | 5.25 | 0.003676 |
1570 - Pancreatic cancer | 0.1764 | 5.30 | 0.003553 |
1550 - Liver cancer | 0.1688 | 5.52 | 0.003128 |
1790 - Uterine cancer | 0.1661 | 4.94 | 0.003867 |
1910 - Brain cancer | 0.1587 | 5.51 | 0.002742 |
1830 - Ovarian cancer | 0.1507 | 3.91 | 0.005705 |
2070 - Leukemias | 0.1461 | 4.10 | 0.003833 |
1950 - Soft tissue tumor of the head and neck | 0.1335 | 4.64 | 0.002627 |
1620 - Lung cancer | 0.1334 | 5.72 | 0.001866 |
1510 - Stomach cancer | 0.1276 | 3.02 | 0.005548 |
1530 - Colon cancer | 0.1060 | 4.09 | 0.002047 |
0430 - Symptomatic HIV and— | |||
1550 - Liver cancer | 0.1619 | 4.25 | 0.004458 |
2020 - Lymphoma | 0.1497 | 3.91 | 0.003553 |
1950 - Soft tissue tumor of the head and neck | 0.1405 | 3.33 | 0.005105 |
1500 - Esophageal cancer | 0.1305 | 2.94 | 0.003553 |
1510 - Stomach cancer | 0.1157 | 2.29 | 0.005733 |
1620 - Lung cancer | 0.1235 | 4.29 | 0.001859 |
SOURCE: Author's calculations using MVP estimation model fitted to a DRF 10% random sample. | |||
NOTES: Correlation estimates are selected for inclusion on the basis of having absolute values for the posterior mean of at least 0.10 and, generally, a t-statistic greater than 2.00.
Data are for applicants who cleared step 1 of the disability determination process.
Diagnoses are arranged by estimated correlation value.
|
About 7.6% of cases with a secondary diagnosis of chronic renal failure combine with a malignant neoplasm as the primary impairment. The highest posterior mean correlation in Table 10 associates chronic renal failure with multiple myeloma (0.23). Mean correlations of chronic renal failure with cancers of the kidney, esophagus, pancreas, liver, uterus, brain, and ovary all exceed 0.15. Additional statistically significant correlations with chronic renal failure are seen for leukemia and neoplasms of the head/neck, lungs, stomach, and colon.
Symptomatic HIV shows positive correlation with lymphoma and cancers of the liver, head/neck, esophagus, stomach, and lungs. From a clinical perspective, several neoplasms, including Kaposi sarcoma, non-Hodgkin's lymphoma, and invasive cervical cancer, are classified as acquired immune deficiency syndrome (AIDS)-defining neoplasms, as they are directly linked to the immunosuppression associated with HIV infection. In addition, numerous epidemiological studies have found a higher incidence of many other neoplasms in the HIV/AIDS infected population (for instance, Frisch and others 2001; Patel and others 2008; and Mani, Haigentz, and Aboulafia 2012). In 8.2% of the 1,648 cases where symptomatic HIV is cited as a secondary impairment, the primary diagnosis involves a malignant neoplasm.
Cancer and Disorders Involving the Spine or Spinal Cord
Several impairments involving the spine or spinal cord are positively correlated with various cancers, as shown in Table 11. Fractures of the vertebral column have an estimated posterior mean correlation of 0.28 with multiple myeloma. Other correlations, ranging from 0.19 down to 0.11, are with malignant neoplasms of the head/neck, brain, esophagus, pancreas, liver, lungs, and kidney; and with leukemia. A separate class of injury (other fractures of bones) also shows positive correlation with multiple myeloma (0.13). Typically, one thinks of impairments in the injury category as the likely result of an accident involving a relatively young claimant. In fact, the median age of claimants with an injury diagnosis is 41, while the median age of filers with a neoplasm is 55. One plausible explanation for the relationship found in the model's estimates is metastasis.
Diagnostic group, impairment code, and diagnosis | Correlation (posterior mean) | t-statistic | Numerical standard error |
---|---|---|---|
Injuries | |||
8060 - Fracture of vertebral column and— | |||
2030 - Multiple myeloma | 0.2822 | 6.29 | 0.005928 |
1950 - Soft tissue tumor of the head and neck | 0.1888 | 3.91 | 0.005837 |
1910 - Brain cancer | 0.1726 | 3.67 | 0.006590 |
1500 - Esophageal cancer | 0.1697 | 2.41 | 0.010885 |
1570 - Pancreatic cancer | 0.1689 | 2.90 | 0.007070 |
1550 - Liver cancer | 0.1605 | 2.92 | 0.007153 |
1620 - Lung cancer | 0.1406 | 3.22 | 0.005113 |
1890 - Kidney cancer | 0.1302 | 2.04 | 0.009415 |
2070 - Leukemias | 0.1110 | 1.94 | 0.006730 |
8290 - Other fractures of bones and— | |||
2030 - Multiple myeloma | 0.1271 | 2.17 | 0.008141 |
Nervous system and sense organ diseases | |||
3350 - Anterior horn cell disease and— | |||
1570 - Pancreatic cancer | 0.2141 | 3.92 | 0.006282 |
1910 - Brain cancer | 0.2128 | 4.48 | 0.005005 |
1500 - Esophageal cancer | 0.2070 | 3.46 | 0.008931 |
1550 - Liver cancer | 0.2018 | 3.92 | 0.007254 |
1510 - Stomach cancer | 0.1980 | 2.63 | 0.012284 |
1620 - Lung cancer | 0.1825 | 4.23 | 0.004016 |
2070 - Leukemias | 0.1662 | 3.53 | 0.005751 |
1950 - Soft tissue tumor of the head and neck | 0.1602 | 2.55 | 0.009198 |
2030 - Multiple myeloma | 0.1590 | 2.37 | 0.010338 |
1830 - Ovarian cancer | 0.1517 | 3.12 | 0.006517 |
1890 - Kidney cancer | 0.1391 | 3.15 | 0.005026 |
1530 - Colon cancer | 0.1288 | 2.38 | 0.007018 |
2020 - Lymphoma | 0.1141 | 1.79 | 0.008648 |
1850 - Prostate cancer | 0.1140 | 2.49 | 0.006681 |
3360 - Other spinal cord disorders and— | |||
1510 - Stomach cancer | 0.1504 | 2.24 | 0.010320 |
2030 - Multiple myeloma | 0.1348 | 1.92 | 0.011504 |
1950 - Soft tissue tumor of the head and neck | 0.1311 | 2.17 | 0.008965 |
1500 - Esophageal cancer | 0.1271 | 1.89 | 0.010614 |
1620 - Lung cancer | 0.1144 | 2.18 | 0.007609 |
1570 - Pancreatic cancer | 0.1090 | 2.30 | 0.005397 |
SOURCE: Author's calculations using MVP estimation model fitted to a DRF 10% random sample. | |||
NOTES: Correlation estimates are selected for inclusion on the basis of having absolute values for the posterior mean of at least 0.10 and, generally, a t-statistic greater than 2.00.
Data are for applicants who cleared step 1 of the disability determination process.
Diagnoses are arranged by estimated correlation value.
|
According to Fourney and others (2003), “destructive vertebral lesions are a common source of morbidity in patients with metastatic disease and multiple myeloma. Approximately 30% of patients with various neoplastic conditions develop symptomatic spinal metastases during the course of their illness, and pain is the presenting complaint in the majority of cases.” In 6.5% of the 526 cases where a fracture of the vertebral column is the secondary impairment, the primary diagnosis is a neoplasm (most often, multiple myeloma).
Metastasis is also a plausible reason for the statistically significant positive correlations of anterior horn cell disease with a host of malignant neoplasms reported in Table 11. Specifically, the estimated posterior mean correlations are greater than 0.20 for anterior horn cell disease with cancers of the pancreas, brain, esophagus, and liver; further, positive correlations are estimated with ten additional cancers. Another impairment of the nervous system/sense organs (other diseases of the spinal cord) likewise shows positive correlation with six types of neoplasms.
Cancer and Other Disorders
Table 12 presents statistically significant correlation estimates involving malignant neoplasms and diagnoses from the other impairment categories. Disorders of the back (musculoskeletal system) are the second most common diagnosis overall. As with the most common impairment (affective/mood diagnoses), disorders of the back are negatively correlated with many of the neoplasms, with the largest absolute magnitudes corresponding to cancers of the lung and liver (−0.23 and −0.20, respectively). Only 0.9% of all primary and secondary diagnoses of disorders of the back combine with a neoplasm.
Diagnostic group, impairment code, and diagnosis | Correlation (posterior mean) | t-statistic | Numerical standard error |
---|---|---|---|
Musculoskeletal system and connective tissue diseases | |||
7240 - Disorders of back and— | |||
1620 - Lung cancer | -0.2288 | -15.04 | 0.000722 |
1550 - Liver cancer | -0.2036 | -8.55 | 0.002143 |
1740 - Breast cancer | -0.1900 | -14.17 | 0.000852 |
1950 - Soft tissue tumor of the head and neck | -0.1856 | -8.72 | 0.001625 |
1570 - Pancreatic cancer | -0.1798 | -7.54 | 0.001876 |
1890 - Kidney cancer | -0.1791 | -5.87 | 0.002867 |
1530 - Colon cancer | -0.1731 | -11.07 | 0.000668 |
1910 - Brain cancer | -0.1721 | -8.30 | 0.001315 |
1830 - Ovarian cancer | -0.1636 | -6.17 | 0.002947 |
1790 - Uterine cancer | -0.1538 | -5.34 | 0.003225 |
1510 - Stomach cancer | -0.1486 | -4.42 | 0.003659 |
1500 - Esophageal cancer | -0.1464 | -5.33 | 0.002403 |
2070 - Leukemias | -0.1432 | -6.53 | 0.001389 |
2020 - Lymphoma | -0.1378 | -7.45 | 0.000970 |
2250 - Brain cancer (benign) | -0.1173 | -4.13 | 0.002853 |
Digestive system diseases | |||
5710 - Chronic liver disease and cirrhosis and— | |||
1550 - Liver cancer | 0.2317 | 8.88 | 0.002976 |
2020 - Lymphoma | 0.1358 | 4.58 | 0.002647 |
5690 - Other gastrointestinal system disorders and— | |||
1530 - Colon cancer | 0.1100 | 3.79 | 0.002818 |
Nervous system and sense organ diseases | |||
3590 - Muscular dystrophies and— | |||
1830 - Ovarian cancer | 0.1482 | 2.42 | 0.009166 |
1570 - Pancreatic cancer | 0.1470 | 2.34 | 0.009212 |
1910 - Brain cancer | 0.1277 | 2.56 | 0.005979 |
1550 - Liver cancer | 0.1215 | 2.41 | 0.006596 |
1620 - Lung cancer | 0.1193 | 2.17 | 0.008209 |
1740 - Breast cancer | 0.1013 | 2.04 | 0.006323 |
Genitourinary system diseases | |||
5990 - Other urinary tract disorders and— | |||
1890 - Kidney cancer | 0.1268 | 2.55 | 0.006675 |
1790 - Uterine cancer | 0.1230 | 2.13 | 0.009002 |
SOURCE: Author's calculations using MVP estimation model fitted to a DRF 10% random sample. | |||
NOTES: Correlation estimates are selected for inclusion on the basis of having absolute values for the posterior mean of at least 0.10 and, generally, a t-statistic greater than 2.00.
Data are for applicants who cleared step 1 of the disability determination process.
Diagnoses are arranged by estimated correlation value. Negative correlations are listed by absolute value.
Cancers are malignant unless otherwise noted.
Diagnoses identified as "other" may be defined in the context of impairments not shown in this table.
|
Turning to the digestive system, chronic liver disease/cirrhosis has positive correlation with both malignant neoplasms of the liver and lymphoma, with posterior mean values of 0.23 and 0.14, respectively. Cirrhosis is one the biggest known risk factors for liver cancer. There is also positive correlation between colon cancer and the catch-all diagnosis of “other impairments of the gastrointestinal system.” In the nervous system/sense organs category, muscular dystrophies have positive correlations with malignant neoplasms of the ovary, pancreas, brain, liver, lungs, and breast. Muscular dystrophy refers to a group of hereditary diseases that result in progressive weakness and loss of muscle mass, as a genetic mutation interferes with normal protein production. For patients with the most common type of muscular dystrophy, Gadalla and others (2011) find evidence of significant excess risk of cancers of the ovaries, brain, colon, and pancreas. The last finding reported in Table 12 involves the catch-all diagnosis in the genitourinary body system category (other disorders of the urinary tract), which is positively associated with malignant neoplasms of the kidney and uterus.
Correlations Involving Mental Impairments
Overall, 45.7% of primary diagnoses involving a mental impairment combine with a secondary diagnosis that is also mental, suggesting a high degree of comorbidity among such disorders. In terms of the correlation estimates, the mental diagnoses can be classified into two separate groups. The first group includes affective/mood disorders, anxiety disorders, personality disorders, schizophrenia, and substance addiction (both drugs and alcohol). The second group encompasses diagnoses such as intellectual disability, learning disorder, autism, attention deficit disorder, and borderline intellectual functioning. Notice that disorders in the second group are typically diagnosed very early in the developmental stage, usually in children, while impairments in the first group tend to have a later age of onset (teenagers and young adults). A coupling of impairments from the two different groups tends to be negatively correlated, while two from within a group tend to have positive correlation. The findings appear in Table 13.
Impairment code and diagnosis | Correlation (posterior mean) | t-statistic | Numerical standard error |
---|---|---|---|
2960 - Affective and mood disorders and— | |||
3000 - Anxiety and obsessive-compulsive disorders | 0.2621 | 46.78 | 0.000141 |
3010 - Personality disorders | 0.2210 | 24.18 | 0.000287 |
3040 - Substance addiction (drugs) | 0.1907 | 16.10 | 0.000431 |
3030 - Substance addiction (alcohol) | 0.1706 | 15.47 | 0.000432 |
3180 - Intellectual disability | -0.2013 | -16.65 | 0.000620 |
2990 - Autistic disorders | -0.1400 | -5.39 | 0.002288 |
3152 - Learning disorder | -0.1344 | -7.93 | 0.000706 |
2950 - Schizophrenic and other psychotic disorders and— | |||
3040 - Substance addiction (drugs) | 0.2909 | 16.75 | 0.001236 |
3030 - Substance addiction (alcohol) | 0.1894 | 10.38 | 0.001130 |
3010 - Personality disorders | 0.1722 | 10.74 | 0.000859 |
3010 - Personality disorders and— | |||
3040 - Substance addiction (drugs) | 0.1174 | 5.44 | 0.001389 |
3030 - Substance addiction (alcohol) | 0.1109 | 5.33 | 0.001240 |
3040 - Substance addiction (drugs) and— | |||
3030 - Substance addiction (alcohol) | 0.1986 | 8.96 | 0.001507 |
3195 - Borderline intellectual functioning and— | |||
3152 - Learning disorder | 0.2034 | 7.62 | 0.001908 |
3140 - Attention deficit/hyperactivity disorder | 0.1659 | 5.43 | 0.002693 |
2990 - Autistic disorders | 0.1336 | 3.58 | 0.003754 |
3140 - Attention deficit/hyperactivity disorder and— | |||
3152 - Learning disorder | 0.2479 | 8.43 | 0.002363 |
2990 - Autistic disorders | 0.1271 | 3.15 | 0.004638 |
3180 - Intellectual disability and— | |||
2990 - Autistic disorders | 0.1471 | 4.41 | 0.002985 |
3000 - Anxiety and obsessive-compulsive disorders | -0.1144 | -6.85 | 0.001156 |
3040 - Substance addiction (drugs) | -0.1018 | -3.27 | 0.002765 |
SOURCE: Author's calculations using MVP estimation model fitted to a DRF 10% random sample. | |||
NOTES: Correlation estimates are selected for inclusion on the basis of having absolute values for the posterior mean of at least 0.10 and, generally, a t-statistic greater than 2.00.
Data are for applicants who cleared step 1 of the disability determination process.
Diagnoses are arranged by estimated correlation value. Negative correlations are listed by absolute value.
|
Affective/mood disorders have posterior mean correlations of 0.26 with anxiety disorders, 0.22 with personality disorders, 0.19 with substance addiction (drugs), and 0.17 with substance addiction (alcohol). Similarly, schizophrenia is positively associated with personality disorders and substance addiction (particularly drugs), while personality disorders have a mean correlation of about 0.11 with both types of substance addiction. Simultaneous abuse of drugs and alcohol yields a posterior mean correlation value of 0.20.
Among the mental impairments in the second group, borderline intellectual functioning has positive correlation with learning disorder (0.20), attention deficit disorder (0.17), and autism (0.13); attention deficit disorder correlates with learning disorder (0.25) and autism (0.13); and intellectual disability has a 0.15 mean correlation with autism. On the other hand, affective/mood disorders are negatively correlated with impairments from the second group (intellectual disability, learning disorder, and autism). Likewise, intellectual disability has negative correlations with impairments in the first group (anxiety and drug addiction).
Combined, drug- and alcohol-related substance addiction represent 1.8% of all primary and secondary diagnoses and result in an initial allowance for about 19% of cases. The co-occurrence of substance abuse with other psychiatric diagnoses is widespread and poses one of the biggest challenges to effective treatment (Grant and others 2004). Two-thirds (66.7%) of primary and secondary diagnoses involving alcohol addiction pair with another mental impairment. The corresponding percentage for drug addiction is higher still, at 78.6%.
Organic Mental Disorders
Organic mental disorders are unique in their likelihood of association with impairments from other diagnostic groups, as they are by definition linked to some underlying external cause. Their positive correlation with both malignant and benign neoplasms of the brain was previously discussed (Table 8). Table 14 reports additional estimated correlations. Various impairments of the nervous system/sense organs have positive correlation with an organic mental diagnosis, including other cerebral degenerations (0.25), late effects of injuries to the nervous system (0.21), epilepsy (0.16), Parkinson's disease (0.15), and multiple sclerosis (0.14). Of all primary and secondary diagnoses involving an organic mental disorder, 10.2% combine with an impairment of the nervous system/sense organs.
Diagnostic group, impairment code, and diagnosis | Correlation (posterior mean) | t-statistic | Numerical standard error |
---|---|---|---|
2940 - Organic mental disorders and— | |||
Nervous system and sense organ diseases | |||
3310 - Other cerebral degenerations | 0.2477 | 9.13 | 0.002510 |
9070 - Late effects of injuries to the nervous system | 0.2070 | 9.34 | 0.001598 |
3450 - Epilepsy | 0.1604 | 12.38 | 0.000569 |
3320 - Parkinson's disease | 0.1512 | 5.53 | 0.002550 |
3400 - Multiple sclerosis | 0.1394 | 7.51 | 0.001180 |
Injuries | |||
8540 - Intracranial (traumatic brain) injury | 0.3539 | 18.48 | 0.001353 |
Circulatory system diseases | |||
4380 - Late effects of cerebrovascular disease | 0.3283 | 28.22 | 0.000717 |
SOURCE: Author's calculations using MVP estimation model fitted to a DRF 10% random sample. | |||
NOTES: Correlation estimates are selected for inclusion on the basis of having absolute values for the posterior mean of at least 0.10 and, generally, a t-statistic greater than 2.00.
Data are for applicants who cleared step 1 of the disability determination process.
Diagnoses are arranged by estimated correlation value.
Diagnoses identified as "other" may be defined in the context of impairments not shown in this table.
|
Table 14 highlights two additional relationships. Organic mental disorders have very high positive correlations with intracranial injury (0.35) and with late effects of cerebrovascular disease (0.33), the latter being in the circulatory system category. In 41.8% of cases where an intracranial injury is the secondary diagnosis, the primary impairment is an organic mental disorder. Likewise, more than 30% of claims where late effects of cerebrovascular disease appear as the secondary diagnosis combine with an organic mental diagnosis as the primary impairment. The reasons for these associations are self-evident: diminished cognitive function that may result from an intracranial injury or any incident involving inadequate blood flow to the brain.
Mental and Musculoskeletal Disorders
Correlation estimates between mental and musculoskeletal impairments appear in Table 15. Recall that disorders of the back and affective/mood disorders are by far the two most common impairments overall, together accounting for a disproportionately high share of the diagnoses (32.4% combined; Table 1). Although not shown in Table 15, the estimated correlation between the two diagnoses is small, but it is both negative and statistically significant (a −0.062 posterior mean with a t-statistic of −10.5). This finding appears to conflict with a popular perception: that affective/mood impairments and disorders of the back are two relatively “subjective” medical diagnoses with a “suspiciously” high degree of association.
Impairment code and diagnosis | Correlation (posterior mean) | t-statistic | Numerical standard error |
---|---|---|---|
7240 - Disorders of back and— | |||
2950 - Schizophrenic and other psychotic disorders | -0.1764 | -14.56 | 0.000591 |
3010 - Personality disorders | -0.1725 | -13.24 | 0.000704 |
2940 - Organic mental disorders | -0.1713 | -18.88 | 0.000320 |
3030 - Substance addiction (alcohol) | -0.1700 | -12.49 | 0.000589 |
3000 - Anxiety and obsessive-compulsive disorders | -0.1667 | -23.57 | 0.000197 |
3040 - Substance addiction (drugs) | -0.1389 | -9.11 | 0.000669 |
7150 - Osteoarthrosis and allied disorders and— | |||
2960 - Affective and mood disorders | -0.1337 | -18.96 | 0.000197 |
2940 - Organic mental disorders | -0.1202 | -10.19 | 0.000421 |
7330 - Other bone and cartilage disorders and— | |||
2960 - Affective and mood disorders | -0.1183 | -9.11 | 0.000553 |
7160 - Other and unspecified arthropathies and— | |||
2960 - Affective and mood disorders | -0.1132 | -14.48 | 0.000196 |
7280 - Disorders of muscle, ligament, and fascia and— | |||
2960 - Affective and mood disorders | -0.1089 | -14.31 | 0.000184 |
7100 - Diffuse diseases of connective tissue and— | |||
2960 - Affective and mood disorders | -0.1076 | -5.47 | 0.000922 |
7370 - Curvature of spine and— | |||
2960 - Affective and mood disorders | -0.1076 | -5.47 | 0.001271 |
SOURCE: Author's calculations using MVP estimation model fitted to a DRF 10% random sample. | |||
NOTES: Correlation estimates are selected for inclusion on the basis of having absolute values for the posterior mean of at least 0.10 and, generally, a t-statistic greater than 2.00.
Data are for applicants who cleared step 1 of the disability determination process.
Diagnoses are arranged by estimated correlation value. Negative correlations are listed by absolute value.
Diagnoses identified as "other" may be defined in the context of impairments not shown in this table.
|
One way to understand the relationship between the two most common diagnoses is by following their conditional and joint probabilities. Chart 8 shows that the probability of a claim involving an affective/mood disorder as the primary or secondary diagnosis peaks at roughly 45% for claimants in their late twenties and early thirties (red lines and orange squares). This probability declines to about 30% at age 50, which roughly coincides with the highest likelihood of a claim involving a disorder of the back (black lines and blue squares). On the other hand, the purple lines and squares represent the probability of having both diagnoses together regardless of order (primary or secondary). The joint probability of an affective/mood impairment and a disorder of the back peaks at about 10% for claimants in their early 40s.
Estimated incidence probabilities of affective or mood disorders, disorders of the back, or both, by age: 2009 initial claims
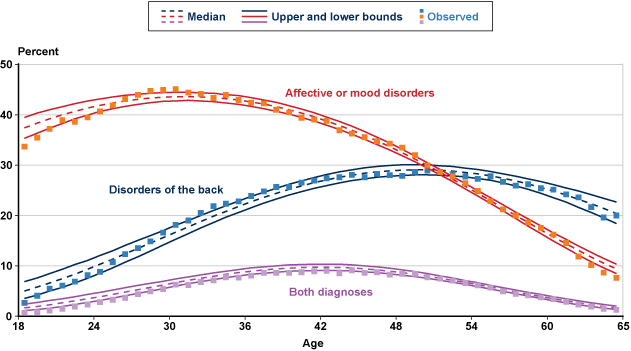
Age | Median | Lower bound | Upper bound | Observed |
---|---|---|---|---|
Affective or mood disorders | ||||
18 | 37.40 | 35.28 | 39.47 | 33.67 |
19 | 38.31 | 36.31 | 40.31 | 35.46 |
20 | 39.18 | 37.32 | 40.98 | 37.19 |
21 | 39.94 | 38.25 | 41.57 | 38.89 |
22 | 40.65 | 39.13 | 42.16 | 38.58 |
23 | 41.27 | 39.89 | 42.67 | 39.40 |
24 | 41.81 | 40.64 | 43.08 | 40.61 |
25 | 42.30 | 41.17 | 43.48 | 41.81 |
26 | 42.70 | 41.64 | 43.84 | 43.12 |
27 | 43.04 | 42.06 | 44.02 | 43.90 |
28 | 43.29 | 42.37 | 44.26 | 44.72 |
29 | 43.46 | 42.61 | 44.37 | 44.87 |
30 | 43.56 | 42.73 | 44.44 | 45.02 |
31 | 43.58 | 42.80 | 44.44 | 44.34 |
32 | 43.52 | 42.68 | 44.32 | 44.08 |
33 | 43.39 | 42.57 | 44.21 | 43.29 |
34 | 43.17 | 42.33 | 43.99 | 43.91 |
35 | 42.88 | 42.01 | 43.74 | 42.91 |
36 | 42.51 | 41.62 | 43.37 | 42.40 |
37 | 42.07 | 41.17 | 42.92 | 42.24 |
38 | 41.55 | 40.66 | 42.40 | 40.94 |
39 | 40.95 | 40.09 | 41.74 | 40.46 |
40 | 40.29 | 39.42 | 41.11 | 39.40 |
41 | 39.55 | 38.71 | 40.39 | 39.06 |
42 | 38.72 | 37.92 | 39.52 | 38.79 |
43 | 37.83 | 37.06 | 38.66 | 36.92 |
44 | 36.89 | 36.12 | 37.69 | 36.24 |
45 | 35.86 | 35.12 | 36.61 | 35.50 |
46 | 34.77 | 34.06 | 35.48 | 34.71 |
47 | 33.64 | 33.00 | 34.34 | 34.25 |
48 | 32.43 | 31.82 | 33.08 | 33.41 |
49 | 31.19 | 30.65 | 31.78 | 31.96 |
50 | 29.87 | 29.36 | 30.46 | 29.87 |
51 | 28.54 | 28.06 | 29.10 | 28.60 |
52 | 27.17 | 26.69 | 27.69 | 27.69 |
53 | 25.76 | 25.26 | 26.31 | 26.38 |
54 | 24.35 | 23.84 | 24.88 | 24.86 |
55 | 22.89 | 22.35 | 23.47 | 22.83 |
56 | 21.42 | 20.87 | 22.08 | 21.15 |
57 | 19.97 | 19.35 | 20.64 | 19.95 |
58 | 18.54 | 17.84 | 19.27 | 18.59 |
59 | 17.11 | 16.39 | 17.89 | 17.48 |
60 | 15.71 | 14.88 | 16.51 | 16.14 |
61 | 14.34 | 13.47 | 15.24 | 14.48 |
62 | 13.02 | 12.12 | 13.93 | 11.84 |
63 | 11.73 | 10.79 | 12.66 | 10.11 |
64 | 10.51 | 9.57 | 11.45 | 8.64 |
65 | 9.36 | 8.41 | 10.32 | 7.58 |
Disorders of the back | ||||
18 | 5.00 | 3.50 | 6.84 | 2.63 |
19 | 5.73 | 4.17 | 7.57 | 4.06 |
20 | 6.55 | 4.94 | 8.45 | 5.44 |
21 | 7.41 | 5.72 | 9.31 | 6.03 |
22 | 8.33 | 6.57 | 10.23 | 6.82 |
23 | 9.27 | 7.53 | 11.07 | 8.08 |
24 | 10.27 | 8.53 | 12.04 | 8.75 |
25 | 11.29 | 9.56 | 12.98 | 10.70 |
26 | 12.35 | 10.70 | 14.05 | 12.33 |
27 | 13.42 | 11.79 | 15.02 | 13.48 |
28 | 14.48 | 12.92 | 15.98 | 14.86 |
29 | 15.58 | 14.07 | 17.00 | 16.54 |
30 | 16.64 | 15.23 | 18.01 | 18.10 |
31 | 17.72 | 16.36 | 18.98 | 18.98 |
32 | 18.76 | 17.49 | 19.98 | 20.48 |
33 | 19.80 | 18.57 | 20.95 | 21.84 |
34 | 20.79 | 19.60 | 21.92 | 22.28 |
35 | 21.76 | 20.57 | 22.84 | 22.74 |
36 | 22.69 | 21.53 | 23.77 | 23.82 |
37 | 23.55 | 22.41 | 24.56 | 24.75 |
38 | 24.38 | 23.23 | 25.40 | 25.63 |
39 | 25.13 | 24.02 | 26.16 | 25.60 |
40 | 25.85 | 24.71 | 26.86 | 26.47 |
41 | 26.48 | 25.37 | 27.50 | 26.91 |
42 | 27.06 | 25.91 | 28.10 | 27.38 |
43 | 27.55 | 26.43 | 28.62 | 27.56 |
44 | 27.99 | 26.87 | 29.04 | 28.02 |
45 | 28.37 | 27.24 | 29.40 | 27.58 |
46 | 28.66 | 27.54 | 29.68 | 28.24 |
47 | 28.86 | 27.78 | 29.87 | 27.95 |
48 | 28.97 | 27.89 | 30.04 | 27.83 |
49 | 29.03 | 28.01 | 30.03 | 28.66 |
50 | 29.02 | 28.00 | 30.01 | 28.87 |
51 | 28.94 | 27.96 | 29.85 | 28.64 |
52 | 28.77 | 27.82 | 29.69 | 28.16 |
53 | 28.52 | 27.62 | 29.38 | 27.72 |
54 | 28.21 | 27.32 | 29.09 | 27.27 |
55 | 27.82 | 26.95 | 28.69 | 27.78 |
56 | 27.34 | 26.46 | 28.25 | 26.64 |
57 | 26.82 | 25.81 | 27.79 | 25.92 |
58 | 26.20 | 25.17 | 27.26 | 26.15 |
59 | 25.53 | 24.37 | 26.69 | 25.37 |
60 | 24.82 | 23.50 | 26.10 | 25.09 |
61 | 24.03 | 22.64 | 25.43 | 24.20 |
62 | 23.16 | 21.59 | 24.81 | 23.55 |
63 | 22.30 | 20.55 | 24.08 | 21.61 |
64 | 21.34 | 19.50 | 23.36 | 19.55 |
65 | 20.37 | 18.35 | 22.62 | 19.98 |
Both diagnoses | ||||
18 | 1.63 | 1.07 | 2.39 | 0.64 |
19 | 1.92 | 1.33 | 2.71 | 0.77 |
20 | 2.26 | 1.60 | 3.07 | 1.07 |
21 | 2.63 | 1.93 | 3.45 | 1.44 |
22 | 3.02 | 2.28 | 3.85 | 1.93 |
23 | 3.42 | 2.68 | 4.28 | 2.29 |
24 | 3.86 | 3.10 | 4.70 | 2.77 |
25 | 4.32 | 3.54 | 5.15 | 3.25 |
26 | 4.77 | 4.01 | 5.61 | 3.60 |
27 | 5.25 | 4.49 | 6.07 | 4.37 |
28 | 5.72 | 4.99 | 6.47 | 4.88 |
29 | 6.19 | 5.45 | 6.92 | 5.37 |
30 | 6.65 | 5.94 | 7.36 | 6.25 |
31 | 7.08 | 6.40 | 7.77 | 6.16 |
32 | 7.51 | 6.84 | 8.19 | 6.79 |
33 | 7.91 | 7.26 | 8.58 | 7.08 |
34 | 8.29 | 7.62 | 8.92 | 7.71 |
35 | 8.62 | 7.95 | 9.24 | 7.64 |
36 | 8.91 | 8.27 | 9.54 | 8.05 |
37 | 9.16 | 8.50 | 9.77 | 8.59 |
38 | 9.37 | 8.72 | 9.99 | 8.54 |
39 | 9.53 | 8.87 | 10.15 | 8.56 |
40 | 9.63 | 8.99 | 10.26 | 8.71 |
41 | 9.69 | 9.06 | 10.33 | 8.91 |
42 | 9.70 | 9.06 | 10.33 | 8.96 |
43 | 9.64 | 9.02 | 10.27 | 8.51 |
44 | 9.54 | 8.94 | 10.17 | 9.07 |
45 | 9.38 | 8.78 | 9.99 | 8.59 |
46 | 9.18 | 8.60 | 9.76 | 8.67 |
47 | 8.93 | 8.38 | 9.50 | 8.23 |
48 | 8.64 | 8.12 | 9.18 | 8.38 |
49 | 8.30 | 7.82 | 8.82 | 8.34 |
50 | 7.94 | 7.50 | 8.41 | 7.71 |
51 | 7.53 | 7.12 | 7.98 | 7.40 |
52 | 7.12 | 6.73 | 7.55 | 7.00 |
53 | 6.67 | 6.29 | 7.06 | 6.56 |
54 | 6.21 | 5.85 | 6.61 | 6.12 |
55 | 5.75 | 5.38 | 6.12 | 5.61 |
56 | 5.27 | 4.90 | 5.64 | 4.90 |
57 | 4.80 | 4.43 | 5.18 | 4.43 |
58 | 4.32 | 3.96 | 4.73 | 4.11 |
59 | 3.87 | 3.50 | 4.27 | 3.70 |
60 | 3.44 | 3.06 | 3.82 | 3.38 |
61 | 3.01 | 2.65 | 3.43 | 2.82 |
62 | 2.62 | 2.27 | 3.03 | 2.30 |
63 | 2.26 | 1.91 | 2.67 | 1.88 |
64 | 1.92 | 1.59 | 2.29 | 1.40 |
65 | 1.62 | 1.29 | 2.00 | 1.23 |
A little more than 30% of 2009 claimants had an affective/mood disorder as a primary or a secondary diagnosis. About 25% of claimants had a primary or secondary diagnosis involving a disorder of the back. If both impairments were uncorrelated, one would expect about 30% of cases with a disorder of the back to combine with an affective/mood disorder (roughly mirroring the proportion among all claimants). Instead, only 20% of cases with a disorder of the back combine with an affective/mood disorder. To put it differently, conditional on observing a diagnosis of a disorder of the back, the likelihood of an affective/mood disorder (20%) is smaller than its frequency among all claimants (30%); hence, the negative correlation estimate between the two impairments. This association applies strictly to qualified claimants at the initial determination level. The sign of the correlation estimate may reverse at later stages of the appeals process. Overall, a little more than 6% of 2009 claimants had a disorder of the back in combination with an affective/mood disorder.
Many of the mental impairments show negative correlation with most of the musculoskeletal diagnoses; note that all correlations shown in Table 15 are negative. Specifically, affective/mood disorders have negative correlation with the third most commonly diagnosed impairment (osteoarthrosis/allied disorders), as well as with disorders of the muscle/ligament/fascia, diffuse diseases of the connective tissue, other diseases of the bone/cartilage, other/unspecified arthropathies, and curvature of the spine. Similarly, disorders of the back are negatively correlated with several mental impairments besides affective/mood disorders, such as schizophrenia, personality disorders, organic mental impairments, anxiety disorders, and substance addiction.
Finally, the rapid expansion in the use of opioid pain medication in the last decade and a half (Centers for Disease Control and Prevention 2011) suggests a plausible positive link between disorders of the back and substance addiction. For example, Morden and others (2014) analyze the prescription pattern of DI-Medicare beneficiaries aged younger than 65 and find that almost 95% of chronic opioid users (defined as beneficiaries filling 6 or more prescriptions) had a musculoskeletal comorbidity. Yet, the correlation estimates of both alcohol and drug addiction with a disorder of the back in Table 15 are unambiguously negative. These findings are not necessarily at odds, given that they describe patterns in two very different populations (initial qualified claimants versus DI beneficiaries with Medicare).18
Mental Diagnoses and Other Impairments
Table 16 presents all remaining statistically significant correlation estimates of mental impairments with diagnoses from other body systems. Chronic liver disease/cirrhosis has posterior mean correlation values of 0.22 and 0.10 with alcohol addiction and drug addiction, respectively. Alcoholism and hepatitis infection (spread via intravenous drug use) are both well-established risk factors for liver disease. In addition, epilepsy has a 0.13 mean correlation with alcohol addiction, while intellectual disability is positively associated with emphysema and chronic pulmonary insufficiency (respiratory system diseases).
Diagnostic group, impairment code, and diagnosis | Correlation (posterior mean) | t-statistic | Numerical standard error |
---|---|---|---|
3030 - Substance addiction (alcohol) and— | |||
Digestive system diseases | |||
5710 - Chronic liver disease and cirrhosis | 0.2191 | 11.51 | 0.001173 |
Nervous system and sense organ diseases | |||
3450 - Epilepsy | 0.1322 | 6.69 | 0.001217 |
Endocrine, nutritional, and metabolic diseases | |||
2780 - Obesity | -0.1319 | -5.34 | 0.002218 |
3180 - Intellectual disability and— | |||
Respiratory system diseases | |||
4920 - Emphysema | 0.1371 | 3.22 | 0.004429 |
4960 - Chronic pulmonary insufficiency | 0.1009 | 4.45 | 0.001720 |
3040 - Substance addiction (drugs) and— | |||
Digestive system diseases | |||
5710 - Chronic liver disease and cirrhosis | 0.1026 | 3.94 | 0.002301 |
Endocrine, nutritional, and metabolic diseases | |||
2780 - Obesity | -0.1121 | -3.92 | 0.002671 |
2960 - Affective and mood disorders and— | |||
Circulatory system diseases | |||
4010 - Essential hypertension | -0.2307 | -32.31 | 0.000256 |
4160 - Chronic pulmonary heart disease | -0.1859 | -6.42 | 0.002687 |
4280 - Heart failure | -0.1794 | -14.54 | 0.000441 |
4430 - Peripheral vascular disease | -0.1554 | -9.20 | 0.000901 |
4380 - Late effects of cerebrovascular disease | -0.1511 | -14.15 | 0.000406 |
4250 - Cardiomyopathy | -0.1393 | -10.08 | 0.000697 |
4590 - Other circulatory system diseases | -0.1371 | -8.23 | 0.000705 |
4540 - Varicose veins of lower extremities | -0.1309 | -4.94 | 0.002257 |
4020 - Hypertensive vascular disease | -0.1122 | -6.12 | 0.001240 |
4100 - Acute myocardial infarction | -0.1107 | -5.44 | 0.001311 |
3950 - Diseases of aortic valve | -0.1095 | -4.84 | 0.001439 |
Injuries | |||
8060 - Fracture of vertebral column | -0.2009 | -8.32 | 0.001800 |
8390 - Dislocations—all types | -0.1679 | -6.14 | 0.002683 |
8540 - Intracranial (traumatic brain) injury | -0.1551 | -8.26 | 0.001067 |
8270 - Fractures of lower limb | -0.1533 | -16.03 | 0.000275 |
8940 - Open soft tissue wound of lower limb | -0.1452 | -5.55 | 0.001914 |
8290 - Other fractures of bones | -0.1344 | -9.03 | 0.000519 |
8180 - Fractures of upper limb | -0.1282 | -9.76 | 0.000473 |
8840 - Open soft tissue wound upper limb | -0.1159 | -4.43 | 0.002123 |
9050 - Late effects of musculoskeletal and connective tissue injuries (amputation) | -0.1025 | -7.72 | 0.000454 |
Respiratory system diseases | |||
7800 - Sleep-related breathing disorders | -0.1229 | -6.42 | 0.000982 |
5190 - Other respiratory system disorders | -0.1089 | -6.71 | 0.000692 |
Skin and subcutaneous tissue diseases | |||
7090 - Other skin and subcutaneous tissue disorders | -0.1248 | -5.21 | 0.001463 |
Nervous system and sense organ diseases | |||
3430 - Cerebral palsy | -0.2125 | -7.44 | 0.002123 |
3690 - Blindness and low vision | -0.1938 | -15.21 | 0.000597 |
3590 - Muscular dystrophies | -0.1898 | -5.33 | 0.003961 |
3620 - Other retina disorders | -0.1771 | -6.81 | 0.002661 |
3360 - Other spinal cord diseases | -0.1713 | -6.60 | 0.001788 |
3890 - Deafness | -0.1570 | -10.70 | 0.000545 |
3650 - Glaucoma | -0.1561 | -6.46 | 0.001962 |
9070 - Late effects injuries to the nervous system | -0.1487 | -8.20 | 0.000998 |
3570 - Diabetic and other peripheral neuropathy | -0.1471 | -11.91 | 0.000433 |
3680 - Visual disturbances | -0.1304 | -7.81 | 0.000763 |
Infectious and parasitic diseases | |||
1350 - Sarcoidosis | -0.1245 | -4.15 | 0.002999 |
Endocrine, nutritional, and metabolic diseases | |||
2780 - Obesity | -0.2680 | -32.65 | 0.000313 |
2500 - Diabetes | -0.1835 | -26.44 | 0.000214 |
2740 - Gout | -0.1372 | -5.74 | 0.001449 |
Genitourinary system diseases | |||
5850 - Chronic renal failure | -0.2543 | -20.22 | 0.000488 |
3000 - Anxiety and obsessive-compulsive disorders and— | |||
Circulatory system diseases | |||
4010 - Essential hypertension | -0.1323 | -12.28 | 0.000312 |
Injuries | |||
8060 - Fracture of vertebral column | -0.1164 | -3.53 | 0.003241 |
Endocrine, nutritional, and metabolic diseases | |||
2780 - Obesity | -0.1811 | -14.09 | 0.000481 |
2500 - Diabetes | -0.1455 | -14.05 | 0.000347 |
2940 - Organic mental disorders and— | |||
Endocrine, nutritional, and metabolic diseases | |||
2780 - Obesity | -0.1203 | -8.10 | 0.000794 |
3010 - Personality disorders and— | |||
Endocrine, nutritional, and metabolic diseases | |||
2780 - Obesity | -0.1034 | -4.83 | 0.001287 |
SOURCE: Author's calculations using MVP estimation model fitted to a DRF 10% random sample. | |||
NOTES: Correlation estimates are selected for inclusion on the basis of having absolute values for the posterior mean of at least 0.10 and, generally, a t-statistic greater than 2.00.
Data are for applicants who cleared step 1 of the disability determination process.
Diagnoses are arranged by estimated correlation value. Negative correlations are listed by absolute value.
Diagnoses identified as "other" may be defined in the context of impairments not shown in this table.
|
The rest of the correlation estimates in Table 16 are negative. For instance, 11 circulatory impairments present negative correlation with affective/mood disorders. Of those 11, the largest (absolute) magnitudes involve essential hypertension (−0.23), chronic pulmonary heart disease (−0.19), and heart failure (−0.18). These estimates run counter to an increasing body of literature recognizing depression as a risk factor for cardiovascular disease, wherein direct physiological pathways and indirect mechanisms such as poor health behavior are both likely to play a role (Gordon and others 2012). Anxiety disorders are also negatively correlated with essential hypertension.
In the injuries category, fractures of the vertebral column are negatively associated with both affective/mood and anxiety disorders. Affective/mood disorders also show negative correlation with eight additional injuries, ten impairments of the nervous system/sense organs, two impairments of the respiratory system (sleep-related breathing disorders and other disorders of the respiratory system), other disorders of the skin, sarcoidosis in the infectious/parasitic disease category, and chronic renal failure in the genitourinary system.
Finally, affective/mood disorders are negatively associated with obesity (−0.27), diabetes (−0.18), and gout (−0.14) in the endocrine/nutritional/metabolic category. Obesity is also negatively correlated with anxiety disorders, personality disorders, organic mental impairments, and substance addiction (drugs and alcohol), while diabetes shows negative correlation with anxiety disorders.
In summary, other than positive correlations with some related mental impairments (Table 13), the strongest statistically significant correlation patterns of affective/mood disorders with impairments from other body systems tend to be negative (Tables 15 and 16).
Correlations Involving Circulatory Impairments
There is a great deal of comorbidity among the circulatory system impairments. In 23.4% of claims with a circulatory primary impairment, the secondary diagnosis is another circulatory disorder. Table 17 identifies 41 positive and statistically significant correlation estimates between circulatory diagnoses. The highest posterior mean magnitude (0.42) is for heart failure with cardiomyopathy. Posterior mean values above 0.20 include heart failure with cardiac dysrhythmias (0.29) and chronic pulmonary heart disease (0.27); cardiomyopathy with cardiac dysrhythmias (0.33), valvular heart disease (0.23), and chronic ischemic heart disease (0.21); chronic ischemic heart disease with acute myocardial infarction (0.26) and peripheral vascular disease (0.21); chronic pulmonary heart disease with valvular heart disease (0.23); and cardiac dysrhythmias with valvular heart disease (0.22).
Impairment code and diagnosis | Correlation (posterior mean) | t-statistic | Numerical standard error |
---|---|---|---|
4280 - Heart failure and— | |||
4250 - Cardiomyopathy | 0.4187 | 27.60 | 0.000760 |
4270 - Cardiac dysrhythmias | 0.2854 | 12.12 | 0.001639 |
4160 - Chronic pulmonary heart disease | 0.2686 | 8.77 | 0.002905 |
4240 - Valvular heart disease | 0.1977 | 6.17 | 0.003513 |
4100 - Acute myocardial infarction | 0.1867 | 7.23 | 0.002241 |
4140 - Chronic ischemic heart disease | 0.1671 | 10.65 | 0.000645 |
3950 - Diseases of aortic valve | 0.1611 | 5.27 | 0.002559 |
4010 - Essential hypertension | 0.1516 | 12.12 | 0.000456 |
4020 - Hypertensive vascular disease | 0.1304 | 4.59 | 0.002259 |
4250 - Cardiomyopathy and— | |||
4270 - Cardiac dysrhythmias | 0.3345 | 13.64 | 0.002065 |
4240 - Valvular heart disease | 0.2328 | 5.88 | 0.004058 |
4140 - Chronic ischemic heart disease | 0.2107 | 12.12 | 0.001019 |
4160 - Chronic pulmonary heart disease | 0.1921 | 5.24 | 0.003965 |
4020 - Hypertensive vascular disease | 0.1803 | 6.04 | 0.002485 |
4100 - Acute myocardial infarction | 0.1442 | 4.28 | 0.003036 |
3950 - Diseases of aortic valve | 0.1347 | 3.52 | 0.003860 |
4590 - Other circulatory system diseases | 0.1191 | 3.98 | 0.002260 |
4010 - Essential hypertension | 0.1122 | 7.43 | 0.000620 |
4140 - Chronic ischemic heart disease and— | |||
4100 - Acute myocardial infarction | 0.2608 | 11.48 | 0.001688 |
4430 - Peripheral vascular disease | 0.2112 | 11.80 | 0.000953 |
4240 - Valvular heart disease | 0.1411 | 4.29 | 0.003095 |
4270 - Cardiac dysrhythmias | 0.1316 | 5.76 | 0.001688 |
4160 - Chronic pulmonary heart disease | 0.1267 | 1.90 | 0.009659 |
4160 - Chronic pulmonary heart disease and— | |||
4240 - Valvular heart disease | 0.2291 | 4.72 | 0.006387 |
4270 - Cardiac dysrhythmias | 0.1572 | 3.66 | 0.004810 |
4430 - Peripheral vascular disease | 0.1061 | 2.62 | 0.004193 |
4270 - Cardiac dysrhythmias and— | |||
4240 - Valvular heart disease | 0.2163 | 5.15 | 0.005017 |
4020 - Hypertensive vascular disease | 0.1067 | 2.54 | 0.004465 |
4380 - Late effects of cerebrovascular disease and— | |||
4010 - Essential hypertension | 0.1897 | 16.65 | 0.000505 |
3950 - Diseases of aortic valve | 0.1067 | 3.51 | 0.001969 |
4590 - Other circulatory system diseases and— | |||
4540 - Varicose veins of lower extremities | 0.1445 | 3.05 | 0.005317 |
4430 - Peripheral vascular disease | 0.1409 | 4.48 | 0.002736 |
3950 - Diseases of aortic valve and— | |||
4240 - Valvular heart disease | 0.1796 | 3.43 | 0.006732 |
4430 - Peripheral vascular disease | 0.1472 | 4.19 | 0.003676 |
4100 - Acute myocardial infarction | 0.1461 | 2.96 | 0.005976 |
4100 - Acute myocardial infarction and— | |||
4020 - Hypertensive vascular disease | 0.1307 | 3.39 | 0.003171 |
4590 - Other circulatory system diseases | 0.1266 | 3.42 | 0.003254 |
4430 - Peripheral vascular disease | 0.1189 | 3.22 | 0.003625 |
4240 - Valvular heart disease | 0.1034 | 1.78 | 0.007477 |
4240 - Valvular heart disease and— | |||
4020 - Hypertensive vascular disease | 0.1065 | 1.85 | 0.008439 |
4430 - Peripheral vascular disease | 0.1060 | 2.60 | 0.004317 |
SOURCE: Author's calculations using MVP estimation model fitted to a DRF 10% random sample. | |||
NOTES: Correlation estimates are selected for inclusion on the basis of having absolute values for the posterior mean of at least 0.10 and, generally, a t-statistic greater than 2.00.
Data are for applicants who cleared step 1 of the disability determination process.
Diagnoses are arranged by estimated correlation value.
Diagnoses identified as "other" may be defined in the context of impairments not shown in this table.
|
Circulatory and Respiratory Diagnoses
The respiratory and circulatory systems are closely related. After all, the main function of the respiratory system is to remove carbon dioxide from the blood and supply the blood with oxygen. The circulatory system's job is to transport the oxygenated blood to the body cells, and transport deoxygenated blood from the body cells back to the lungs. Several circulatory impairments (particularly chronic pulmonary heart disease and heart failure) are positively associated with respiratory diagnoses, as shown in Table 18.19
Impairment code and diagnosis | Correlation (posterior mean) | t-statistic | Numerical standard error |
---|---|---|---|
5190 - Other respiratory system disorders and— | |||
4160 - Chronic pulmonary heart disease | 0.2218 | 5.10 | 0.005227 |
4280 - Heart failure | 0.1286 | 4.23 | 0.002404 |
4590 - Other circulatory system diseases | 0.1120 | 3.23 | 0.004097 |
4960 - Chronic pulmonary insufficiency and— | |||
4160 - Chronic pulmonary heart disease | 0.2114 | 7.64 | 0.003076 |
4280 - Heart failure | 0.1526 | 9.71 | 0.000812 |
4140 - Chronic ischemic heart disease | 0.1056 | 8.41 | 0.000542 |
4920 - Emphysema and— | |||
4160 - Chronic pulmonary heart disease | 0.1917 | 4.02 | 0.005618 |
4100 - Acute myocardial infarction | 0.1411 | 3.29 | 0.004924 |
4280 - Heart failure | 0.1143 | 3.68 | 0.002645 |
7800 - Sleep-related breathing disorders and— | |||
4160 - Chronic pulmonary heart disease | 0.1914 | 4.24 | 0.005609 |
4280 - Heart failure | 0.1475 | 4.90 | 0.002219 |
4250 - Cardiomyopathy | 0.1221 | 3.52 | 0.003275 |
4020 - Hypertensive vascular disease | 0.1194 | 2.86 | 0.003788 |
4270 - Cardiac dysrhythmias | 0.1026 | 2.18 | 0.005448 |
SOURCE: Author's calculations using MVP estimation model fitted to a DRF 10% random sample. | |||
NOTES: Correlation estimates are selected for inclusion on the basis of having absolute values for the posterior mean of at least 0.10 and, generally, a t-statistic greater than 2.00.
Data are for applicants who cleared step 1 of the disability determination process.
Diagnoses are arranged by estimated correlation value.
Diagnoses identified as "other" may be defined in the context of impairments not shown in this table.
|
Chronic pulmonary heart disease shows a posterior mean correlation of 0.22 with other disorders of the respiratory system, 0.21 with chronic pulmonary insufficiency, and 0.19 with both emphysema and sleep-related breathing disorders. Heart failure is positively correlated with the same four respiratory impairments. There is positive association between emphysema and acute myocardial infarction, between chronic pulmonary insufficiency and chronic ischemic heart disease, and between the catch-all diagnoses of other diseases of the respiratory and circulatory systems. Finally, sleep-related breathing disorders have positive correlation with several other circulatory diagnoses, including cardiomyopathy, hypertensive vascular disease, and cardiac dysrhythmias. Overall, 15% of respiratory diagnoses combine with a circulatory impairment. More specifically, 23% of claims in which chronic pulmonary heart disease is the secondary diagnosis combine with a disorder of the respiratory system.
Circulatory Impairments and Endocrine, Nutritional, and Metabolic Impairments
Obesity and diabetes are well-established risk factors for cardiovascular disease. Table 19 displays some of the estimated correlations involving circulatory impairments and endocrine/nutritional/metabolic disorders. About 13% of obesity diagnoses and 25% of diabetes diagnoses combine with a circulatory impairment. Obesity has its highest posterior mean correlation with varicose veins of the lower extremities (0.20), followed by chronic pulmonary heart disease (0.17), other diseases of the circulatory system (0.16), and heart failure (0.12). Diabetes is positively correlated with essential hypertension (0.18), peripheral vascular disease (0.16), chronic ischemic heart disease and heart failure (both 0.13), other diseases of the circulatory system (0.11), and hypertensive vascular disease (0.10).
Impairment code and diagnosis | Correlation (posterior mean) | t-statistic | Numerical standard error |
---|---|---|---|
2780 - Obesity and— | |||
4540 - Varicose veins of lower extremities | 0.2011 | 7.03 | 0.001965 |
4160 - Chronic pulmonary heart disease | 0.1726 | 5.53 | 0.003275 |
4590 - Other circulatory system diseases | 0.1633 | 9.02 | 0.001034 |
4280 - Heart failure | 0.1171 | 7.83 | 0.000634 |
2500 - Diabetes and— | |||
4010 - Essential hypertension | 0.1751 | 22.10 | 0.000175 |
4430 - Peripheral vascular disease | 0.1603 | 10.24 | 0.000790 |
4280 - Heart failure | 0.1335 | 10.22 | 0.000576 |
4140 - Chronic ischemic heart disease | 0.1313 | 12.43 | 0.000371 |
4590 - Other circulatory system diseases | 0.1132 | 6.36 | 0.001125 |
4020 - Hypertensive vascular disease | 0.1047 | 5.46 | 0.000962 |
2740 - Gout and— | |||
4010 - Essential hypertension | 0.1603 | 6.52 | 0.001949 |
4280 - Heart failure | 0.1104 | 2.80 | 0.005923 |
2460 - All disorders of thyroid and— | |||
4270 - Cardiac dysrhythmias | 0.1159 | 2.85 | 0.004555 |
SOURCE: Author's calculations using MVP estimation model fitted to a DRF 10% random sample. | |||
NOTES: Correlation estimates are selected for inclusion on the basis of having absolute values for the posterior mean of at least 0.10 and, generally, a t-statistic greater than 2.00.
Data are for applicants who cleared step 1 of the disability determination process.
Diagnoses are arranged by estimated correlation value.
Diagnoses identified as "other" may be defined in the context of impairments not shown in this table.
|
Two other metabolic impairments show positive statistically significant correlation with circulatory disorders. Hypertension is a common comorbidity among patients with gout. Gout is also positively associated with heart failure (see Zhu, Pandya, and Choi 2012). In addition, thyroid disease (specifically, all disorders of the thyroid except malignant neoplasm) shows positive correlation with cardiac dysrhythmias.
Circulatory and Musculoskeletal Diagnoses
Estimated correlations between circulatory and musculoskeletal impairments appear in Table 20. The two most common musculoskeletal diagnoses, disorders of the back and osteoarthrosis/allied disorders, show negative correlation with various circulatory disorders. Specifically, back disorders—the second most frequent diagnosis overall—is negatively correlated with 11 circulatory impairments, with the largest absolute magnitudes for late effects of cerebrovascular disease and heart failure. Similarly, osteoarthrosis/allied disorders (the third most common impairment overall) has negative correlations with late effects of cerebrovascular disease, heart failure, and peripheral vascular disease. The only significant positive association of a musculoskeletal disorder with the circulatory system involves diffuse diseases of the connective tissue, which correlates with valvular heart disease and chronic pulmonary heart disease.
Impairment code and diagnosis | Correlation (posterior mean) | t-statistic | Numerical standard error |
---|---|---|---|
7100 - Diffuse diseases of connective tissue and— | |||
4160 - Chronic pulmonary heart disease | 0.1468 | 2.95 | 0.006516 |
4240 - Valvular heart disease | 0.1019 | 2.40 | 0.004343 |
7240 - Disorders of back and— | |||
4380 - Late effects of cerebrovascular disease | -0.2439 | -21.36 | 0.000427 |
4280 - Heart failure | -0.2412 | -18.61 | 0.000544 |
4250 - Cardiomyopathy | -0.1948 | -13.07 | 0.000635 |
4140 - Chronic ischemic heart disease | -0.1668 | -16.93 | 0.000418 |
4430 - Peripheral vascular disease | -0.1383 | -9.07 | 0.000741 |
4100 - Acute myocardial infarction | -0.1319 | -6.26 | 0.001379 |
4160 - Chronic pulmonary heart disease | -0.1318 | -4.50 | 0.002355 |
4240 - Valvular heart disease | -0.1302 | -5.45 | 0.001920 |
3950 - Diseases of aortic valve | -0.1226 | -5.53 | 0.001075 |
4590 - Other circulatory system diseases | -0.1168 | -7.54 | 0.000715 |
4270 - Cardiac dysrhythmias | -0.1168 | -6.51 | 0.001071 |
7150 - Osteoarthrosis and allied disorders and— | |||
4380 - Late effects of cerebrovascular disease | -0.1375 | -9.97 | 0.000611 |
4430 - Peripheral vascular disease | -0.1311 | -6.47 | 0.001478 |
4280 - Heart failure | -0.1162 | -7.95 | 0.000742 |
SOURCE: Author's calculations using MVP estimation model fitted to a DRF 10% random sample. | |||
NOTES: Correlation estimates are selected for inclusion on the basis of having absolute values for the posterior mean of at least 0.10 and, generally, a t-statistic greater than 2.00.
Data are for applicants who cleared step 1 of the disability determination process.
Diagnoses are arranged by estimated correlation value. Negative correlations are listed by absolute value.
Diagnoses identified as "other" may be defined in the context of impairments not shown in this table.
|
Circulatory and Other Diagnoses
Table 21 shows additional statistically significant estimated correlations for circulatory system diseases with impairments from all other remaining categories, beginning with genitourinary system diseases. As previously discussed, chronic kidney disease is a risk factor for both infections and cancer. The risk for cardiovascular disease also increases notably in individuals with chronic kidney disease. Overall, 24% of chronic renal failure diagnoses combine with a circulatory disorder. Chronic renal failure has correlations of 0.23 with heart failure, 0.20 with essential hypertension, 0.16 with hypertensive vascular disease, and 0.11 with three other circulatory diagnoses (cardiomyopathy, peripheral vascular disease, and late effects of cerebrovascular disease). In addition, essential hypertension is positively associated with a second genitourinary diagnosis (the catch-all category of other disorders of the urinary tract).
Diagnostic group, impairment code, and diagnosis | Correlation (posterior mean) | t-statistic | Numerical standard error |
---|---|---|---|
4280 - Heart failure and— | |||
Genitourinary system diseases | |||
5850 - Chronic renal failure | 0.2270 | 13.48 | 0.001007 |
4430 - Peripheral vascular disease and— | |||
Injuries | |||
9050 - Late effects of musculoskeletal and connective tissue injuries (amputation) | 0.2111 | 7.88 | 0.002591 |
8940 - Open soft tissue wound of lower limb | 0.1905 | 4.29 | 0.004534 |
Genitourinary system diseases | |||
5850 - Chronic renal failure | 0.1115 | 4.24 | 0.002192 |
4010 - Essential hypertension and— | |||
Genitourinary system diseases | |||
5850 - Chronic renal failure | 0.1973 | 15.35 | 0.000619 |
5990 - Other urinary tract disorders | 0.1144 | 4.29 | 0.002026 |
4540 - Varicose veins of lower extremities and— | |||
Skin and subcutaneous tissue diseases | |||
7090 - Other skin and subcutaneous tissue disorders | 0.1577 | 3.10 | 0.005817 |
4020 - Hypertensive vascular disease and— | |||
Genitourinary system diseases | |||
5850 - Chronic renal failure | 0.1570 | 5.52 | 0.002243 |
4380 - Late effects of cerebrovascular disease and— | |||
Injuries | |||
8540 - Intracranial (traumatic brain) injury | 0.1443 | 5.32 | 0.002368 |
Nervous system and sense organ diseases | |||
3680 - Visual disturbances | 0.1291 | 5.10 | 0.001836 |
9070 - Late effects of injuries to the nervous system | 0.1246 | 4.17 | 0.003035 |
3690 - Blindness and low vision | 0.1074 | 4.81 | 0.001836 |
3450 - Epilepsy | 0.1061 | 5.41 | 0.001154 |
3620 - Other retina disorders | 0.1048 | 3.32 | 0.002168 |
Genitourinary system diseases | |||
5850 - Chronic renal failure | 0.1117 | 6.06 | 0.001210 |
4160 - Chronic pulmonary heart disease and— | |||
Infectious and parasitic diseases | |||
1350 - Sarcoidosis | 0.1401 | 2.93 | 0.004991 |
4590 - Other circulatory system diseases and— | |||
Injuries | |||
8940 - Open soft tissue wound of lower limb | 0.1278 | 2.78 | 0.005207 |
4250 - Cardiomyopathy and— | |||
Genitourinary system diseases | |||
5850 - Chronic renal failure | 0.1111 | 4.70 | 0.001796 |
SOURCE: Author's calculations using MVP estimation model fitted to a DRF 10% random sample. | |||
NOTES: Correlation estimates are selected for inclusion on the basis of having absolute values for the posterior mean of at least 0.10 and, generally, a t-statistic greater than 2.00.
Data are for applicants who cleared step 1 of the disability determination process.
Diagnoses are arranged by estimated correlation value.
Diagnoses identified as "other" may be defined in the context of impairments not shown in this table.
|
Among the circulatory diagnoses, late effects of cerebrovascular disease (the long-term consequences of inadequate supply of blood to the brain) is the impairment with the greatest likelihood of a positive association with diagnoses from many other diagnostic groups. I previously discussed its positive correlation with benign and malignant neoplasms of the brain and with organic mental disorders. Late effects of cerebrovascular disease also have positive correlations with intracranial injury and with multiple impairments in the nervous system/sense organs category, including visual disturbances, epilepsy, blindness/low vision, other retina disorders, and late effects of injuries to the nervous system. In about 10.8% of cases for which the secondary impairment is a late effect of cerebrovascular disease, the primary diagnosis is a disorder of the nervous system/sense organs.
Several injuries other than intracranial injury (which is positively correlated with late effects of cerebrovascular disease) have positive association with circulatory system diagnoses. Specifically, open wounds of the lower limb have a 0.13 mean correlation with other disorders of the circulatory system, while peripheral vascular disease is positively associated with open wounds of the lower limb (0.19) and with late effects of musculoskeletal/connective tissue injury (0.21). Finally, the catch-all diagnosis of other disorders of the skin has an estimated correlation of 0.16 with varicose veins of the lower extremities, whereas the correlation between sarcoidosis (infectious/parasitic category) and chronic pulmonary heart disease is 0.14.
Correlations Involving Musculoskeletal Impairments
The findings covered in previous sections suggest that, for disorders of the back and other musculoskeletal system diagnoses, the estimated correlations with the greatest absolute magnitudes tend to be negative. For instance, disorders of the back has statistically significant negative correlations with multiple malignant neoplasms, various mental impairments (including affective/mood disorders), and multiple circulatory system diagnoses. Table 22 provides additional estimates for the two most common musculoskeletal diagnoses (disorders of the back and osteoarthrosis/allied disorders).
Diagnostic group, impairment code, and diagnosis | Correlation (posterior mean) | t-statistic | Numerical standard error |
---|---|---|---|
7240 - Disorders of back and— | |||
Musculoskeletal system and connective tissue diseases | |||
7100 - Diffuse diseases of connective tissue | -0.1318 | -7.46 | 0.001172 |
7140 - Rheumatoid arthritis | -0.1078 | -8.58 | 0.000421 |
Respiratory system diseases | |||
5190 - Other respiratory system disorders | -0.1403 | -7.56 | 0.001177 |
4960 - Chronic pulmonary insufficiency | -0.1335 | -14.72 | 0.000291 |
4920 - Emphysema | -0.1173 | -5.39 | 0.001638 |
Nervous system and sense organ diseases | |||
3540 - Carpal tunnel syndrome | 0.1065 | 8.66 | 0.000440 |
3620 - Other retina disorders | -0.1690 | -7.11 | 0.001621 |
3350 - Anterior horn cell disease | -0.1481 | -4.33 | 0.003731 |
3450 - Epilepsy | -0.1464 | -12.79 | 0.000491 |
3690 - Blindness and low vision | -0.1438 | -10.53 | 0.000421 |
3310 - Other cerebral degenerations | -0.1253 | -4.79 | 0.001759 |
3400 - Multiple sclerosis | -0.1172 | -8.71 | 0.000639 |
3680 - Visual disturbances | -0.1164 | -6.86 | 0.000906 |
3650 - Glaucoma | -0.1132 | -4.65 | 0.001406 |
Injuries | |||
8540 - Intracranial (traumatic brain) injury | -0.1541 | -7.50 | 0.001592 |
8270 - Fractures of lower limb | -0.1036 | -9.78 | 0.000287 |
Endocrine, nutritional, and metabolic diseases | |||
2500 - Diabetes | -0.1702 | -22.25 | 0.000253 |
Genitourinary system diseases | |||
5850 - Chronic renal failure | -0.2293 | -16.75 | 0.000666 |
Digestive system diseases | |||
5710 - Chronic liver disease and cirrhosis | -0.1599 | -14.16 | 0.000413 |
5690 - Other gastrointestinal system disorders | -0.1105 | -8.76 | 0.000514 |
Infectious and parasitic diseases | |||
0430 - Symptomatic HIV | -0.1855 | -10.71 | 0.000977 |
0440 - Asymptomatic HIV | -0.1377 | -7.20 | 0.001322 |
1350 - Sarcoidosis | -0.1212 | -4.30 | 0.002080 |
7150 - Osteoarthrosis and allied disorders and— | |||
Respiratory system diseases | |||
4920 - Emphysema | -0.1095 | -4.31 | 0.001871 |
4960 - Chronic pulmonary insufficiency | -0.1091 | -9.97 | 0.000431 |
Endocrine, nutritional, and metabolic diseases | |||
2780 - Obesity | 0.2305 | 28.44 | 0.000264 |
Genitourinary system diseases | |||
5850 - Chronic renal failure | -0.1105 | -6.65 | 0.000976 |
SOURCE: Author's calculations using MVP estimation model fitted to a DRF 10% random sample. | |||
NOTES: Correlation estimates are selected for inclusion on the basis of having absolute values for the posterior mean of at least 0.10 and, generally, a t-statistic greater than 2.00.
Data are for applicants who cleared step 1 of the disability determination process.
Diagnoses are arranged by estimated correlation value. Negative correlations are listed by absolute value.
Diagnoses identified as "other" may be defined in the context of impairments not shown in this table.
|
A disorder of the back is negatively correlated with two other musculoskeletal impairments (rheumatoid arthritis and diffuse diseases of the connective tissue). Disorders of the back likewise shows negative correlations with three respiratory impairments (emphysema, chronic pulmonary insufficiency, and other disorders of the respiratory system), two injuries (fractures of the lower limb and intracranial injuries), one endocrine/nutritional/metabolic impairment (diabetes), and one genitourinary system impairment (chronic renal failure).
Additional negative correlations with disorders of the back involve other disorders of the gastrointestinal system and chronic liver disease/cirrhosis (digestive system), sarcoidosis and both symptomatic and asymptomatic HIV (infectious/parasitic group), and multiple impairments of the nervous system/sense organs. In fact, the only significant positive estimated correlation involving disorders of the back is with a nervous system impairment (carpal tunnel syndrome).
As previously discussed, the second most common musculoskeletal impairment (osteoarthrosis/allied disorders) has negative correlations with affective/mood and organic mental disorders (Table 15). Table 22 highlights additional negative estimated correlations: with chronic renal failure, emphysema, and chronic pulmonary insufficiency. There is, however, a high positive estimated correlation between osteoarthrosis and obesity (0.23 posterior mean). Overall, 14% of musculoskeletal diagnoses combine with another musculoskeletal impairment.
Other Musculoskeletal Diagnoses
Recalling the discussion based on the estimates presented in Table 4, two musculoskeletal disorders (rheumatoid arthritis and diffuse diseases of the connective tissue) are characterized by a disproportionately high incidence among female claimants. As shown in Table 23, these are the only two musculoskeletal diagnoses with high positive statistically significant correlation with one another (0.28 posterior mean). In 10.6% of cases with a diffuse disease of the connective tissue as a secondary impairment, the primary diagnosis is rheumatoid arthritis. Indeed, rheumatoid arthritis (a chronic inflammatory condition typically affecting the joints) is one of the most common connective tissue disorders. In addition, diffuse diseases of the connective tissue is the musculoskeletal diagnosis most likely to have a positive association with disorders in other body systems.
Diagnostic group, impairment code, and diagnosis | Correlation (posterior mean) | t-statistic | Numerical standard error |
---|---|---|---|
7100 - Diffuse diseases of connective tissue and— | |||
Musculoskeletal system and connective tissue diseases | |||
7140 - Rheumatoid arthritis | 0.2775 | 10.19 | 0.002317 |
Respiratory system diseases | |||
5190 - Other respiratory system disorders | 0.1313 | 3.51 | 0.004229 |
4960 - Chronic pulmonary insufficiency | 0.1002 | 3.54 | 0.002423 |
Nervous system and sense organ diseases | |||
3590 - Muscular dystrophies | 0.1504 | 2.87 | 0.007619 |
3540 - Carpal tunnel syndrome | 0.1028 | 2.54 | 0.004382 |
Genitourinary system diseases | |||
5850 - Chronic renal failure | 0.1794 | 6.78 | 0.002319 |
Endocrine, nutritional, and metabolic diseases | |||
2460 - All disorders of thyroid | 0.1107 | 2.34 | 0.006204 |
7140 - Rheumatoid arthritis and— | |||
Endocrine, nutritional, and metabolic diseases | |||
2460 - All disorders of thyroid | 0.1342 | 3.85 | 0.003145 |
2740 - Gout | 0.1212 | 3.16 | 0.004615 |
7330 - Other bone and cartilage disorders and— | |||
Injuries | |||
8390 - Dislocations—all types | 0.1237 | 2.84 | 0.004422 |
8290 - Other fractures of bones | 0.1171 | 3.78 | 0.002310 |
7280 - Disorders of muscle, ligament, and fascia and— | |||
Nervous system and sense organ diseases | |||
3540 - Carpal tunnel syndrome | 0.1008 | 5.37 | 0.000956 |
SOURCE: Author's calculations using MVP estimation model fitted to a DRF 10% random sample. | |||
NOTES: Correlation estimates are selected for inclusion on the basis of having absolute values for the posterior mean of at least 0.10 and, generally, a t-statistic greater than 2.00.
Data are for applicants who cleared step 1 of the disability determination process.
Diagnoses are arranged by estimated correlation value.
Diagnoses identified as "other" may be defined in the context of impairments not shown in this table.
|
Diffuse diseases of the connective tissue are positively associated with chronic pulmonary insufficiency and other disorders of the respiratory system; with two diagnoses of the nervous system/sense organ category (muscular dystrophies and carpal tunnel syndrome); and with chronic renal failure (genitourinary system) and all disorders of the thyroid (endocrine/nutritional/metabolic group). On the other hand, rheumatoid arthritis is positively associated with both gout and all disorders of the thyroid (endocrine/nutritional/metabolic group).
A third musculoskeletal impairment (other disorders of the bone and cartilage) shows positive correlation with other fractures of bones and with dislocations of all types (injuries category). Finally, a fourth musculoskeletal diagnosis (disorders of the muscle/ligament/fascia) has a positive correlation with carpal tunnel syndrome (nervous system/sense organs).
Correlations Involving Respiratory Disorders
Table 18 highlighted estimated positive associations between circulatory system diagnoses (especially chronic pulmonary heart disease and heart failure) and respiratory system diagnoses. Not surprisingly, respiratory impairments also tend to be positively associated with one another (Table 24). The highest mean correlation magnitudes associate chronic pulmonary insufficiency with emphysema (0.36), asthma (0.22), other disorders of the respiratory system (0.19), and sleep-related breathing disorders (0.18). Asthma has positive correlations with emphysema and other disorders of the respiratory system, while emphysema is associated with other disorders of the respiratory system and sleep-related breathing disorders. Of all primary diagnoses of a respiratory impairment, 8.3% combine with a secondary respiratory disorder.
Impairment code and diagnosis | Correlation (posterior mean) | t-statistic | Numerical standard error |
---|---|---|---|
4960 - Chronic pulmonary insufficiency and— | |||
4920 - Emphysema | 0.3566 | 18.24 | 0.001420 |
4930 - Asthma | 0.2222 | 15.72 | 0.000567 |
5190 - Other respiratory system disorders | 0.1910 | 8.54 | 0.001249 |
7800 - Sleep-related breathing disorders | 0.1782 | 7.14 | 0.001911 |
4930 - Asthma and— | |||
5190 - Other respiratory system disorders | 0.1504 | 5.89 | 0.001848 |
4920 - Emphysema | 0.1258 | 3.75 | 0.003344 |
4920 - Emphysema and— | |||
7800 - Sleep-related breathing disorders | 0.1479 | 3.50 | 0.004734 |
5190 - Other respiratory system disorders | 0.1130 | 3.30 | 0.003661 |
5190 - Other respiratory system disorders and— | |||
7800 - Sleep-related breathing disorders | 0.1291 | 3.34 | 0.003912 |
SOURCE: Author's calculations using MVP estimation model fitted to a DRF 10% random sample. | |||
NOTES: Correlation estimates are selected for inclusion on the basis of having absolute values for the posterior mean of at least 0.10 and, generally, a t-statistic greater than 2.00.
Data are for applicants who cleared step 1 of the disability determination process.
Diagnoses are arranged by estimated correlation value.
Diagnoses identified as "other" may be defined in the context of impairments not shown in this table.
|
Respiratory Impairments and Other Diagnoses
Table 25 summarizes significant correlations between respiratory disorders and other impairments not yet covered. Sarcoidosis (in the infectious/parasitic category) shows positive correlations with multiple respiratory diagnoses, including other disorders of the respiratory system, asthma, chronic pulmonary insufficiency, and emphysema. By some accounts, sarcoidosis may affect the lungs in as many as 90% of cases (American Thoracic Society 1999). Overall, about 12% of sarcoidosis diagnoses combine with a respiratory impairment.
Diagnostic group, impairment code, and diagnosis | Correlation (posterior mean) | t-statistic | Numerical standard error |
---|---|---|---|
5190 - Other respiratory system disorders and— | |||
Infectious and parasitic diseases | |||
1350 - Sarcoidosis | 0.1847 | 3.97 | 0.005438 |
0430 - Symptomatic HIV | 0.1219 | 3.55 | 0.003129 |
Nervous system and sense organ diseases | |||
3590 - Muscular dystrophies | 0.1291 | 2.08 | 0.009287 |
Digestive system diseases | |||
5690 - Other gastrointestinal system disorders | 0.1154 | 3.32 | 0.003401 |
5550 - Crohn's disease | 0.1012 | 2.33 | 0.004514 |
Endocrine, nutritional, and metabolic diseases | |||
2780 - Obesity | 0.1045 | 4.83 | 0.001190 |
4960 - Chronic pulmonary insufficiency and— | |||
Infectious and parasitic diseases | |||
1350 - Sarcoidosis | 0.1327 | 3.76 | 0.003004 |
Endocrine, nutritional, and metabolic diseases | |||
2500 - Diabetes | -0.1010 | -8.07 | 0.000621 |
7800 - Sleep-related breathing disorders and— | |||
Endocrine, nutritional, and metabolic diseases | |||
2780 - Obesity | 0.2708 | 12.52 | 0.001702 |
4930 - Asthma and— | |||
Infectious and parasitic diseases | |||
1350 - Sarcoidosis | 0.1592 | 4.50 | 0.003386 |
4920 - Emphysema and— | |||
Infectious and parasitic diseases | |||
1350 - Sarcoidosis | 0.1031 | 2.26 | 0.005148 |
SOURCE: Author's calculations using MVP estimation model fitted to a DRF 10% random sample. | |||
NOTES: Correlation estimates are selected for inclusion on the basis of having absolute values for the posterior mean of at least 0.10 and, generally, a t-statistic greater than 2.00.
Data are for applicants who cleared step 1 of the disability determination process.
Diagnoses are arranged by estimated correlation value.
Diagnoses identified as "other" may be defined in the context of impairments not shown in this table.
|
The highest estimated correlation in Table 25 involves an endocrine/nutritional/metabolic disorder. Obesity and sleep-related breathing disorders have a 0.27 posterior mean correlation. The comorbidity of sleep-related breathing disorders with obesity and cardiovascular disease is well-established. In addition, the catch-all category of other disorders of the respiratory system is positively associated with symptomatic HIV, obesity, muscular dystrophies, and two digestive-system diagnoses (Crohn's disease and other disorders of the gastrointestinal system).20 The only negative estimated correlation in Table 25 is between diabetes and chronic pulmonary insufficiency.
Correlations Involving Endocrine, Nutritional, and Metabolic Disorders
In this data set, the endocrine/nutritional/metabolic category comprises four impairments: obesity, diabetes, gout, and all disorders of the thyroid. Diabetes and obesity are the fifth and seventh most common diagnoses, respectively (Table 1). As previously discussed, the endocrine impairments positively correlate with various circulatory diagnoses, while obesity shows very high positive association with osteoarthrosis/allied disorders and sleep-related breathing disorders. Table 26 presents additional significant estimated correlations with other diagnoses, which for the most part reflect the fact that diabetes is a leading cause of limb amputation, blindness, and kidney dialysis in the United States.
Diagnostic group, impairment code, and diagnosis | Correlation (posterior mean) | t-statistic | Numerical standard error |
---|---|---|---|
2500 - Diabetes and— | |||
Nervous system and sense organ diseases | |||
3620 - Other retina disorders | 0.3430 | 17.83 | 0.001692 |
3570 - Diabetic and other peripheral neuropathy | 0.2646 | 23.93 | 0.000418 |
3690 - Blindness and low vision | 0.1347 | 9.44 | 0.000561 |
3680 - Visual disturbances | 0.1204 | 6.10 | 0.001015 |
3650 - Glaucoma | 0.1117 | 4.88 | 0.001662 |
Genitourinary system diseases | |||
5850 - Chronic renal failure | 0.2395 | 20.21 | 0.000615 |
Injuries | |||
9050 - Late effects of musculoskeletal and connective tissue injuries (amputation) | 0.1711 | 11.34 | 0.000768 |
8940 - Open soft tissue wound of lower limb | 0.1410 | 5.03 | 0.002567 |
Skin and subcutaneous tissue diseases | |||
7090 - Other skin and subcutaneous tissue disorders | 0.1184 | 4.76 | 0.001490 |
2740 - Gout and— | |||
Injuries | |||
8940 - Open soft tissue wound of lower limb | 0.1342 | 2.16 | 0.010043 |
Infectious and parasitic diseases | |||
0430 - Symptomatic HIV | 0.1297 | 2.51 | 0.006387 |
Nervous system and sense organ diseases | |||
3680 - Visual disturbances | 0.1169 | 2.65 | 0.004774 |
Genitourinary system diseases | |||
5850 - Chronic renal failure | 0.1086 | 2.92 | 0.004269 |
SOURCE: Author's calculations using MVP estimation model fitted to a DRF 10% random sample. | |||
NOTES: Correlation estimates are selected for inclusion on the basis of having absolute values for the posterior mean of at least 0.10 and, generally, a t-statistic greater than 2.00.
Data are for applicants who cleared step 1 of the disability determination process.
Diagnoses are arranged by estimated correlation value.
Diagnoses identified as "other" may be defined in the context of impairments not shown in this table.
|
Diabetes and gout have positive correlation with open wounds of the lower limb in the injuries category. About 11.8% of claims involving an open wound of the lower limb combine with diabetes. Diabetes is also positively associated with late effects of musculoskeletal/connective tissue injuries. Patients with diabetes can develop peripheral vascular disease that may eventually lead to amputation. In a 10-year population-based cohort study, Johannesson and others (2009) find that people with diabetes are eight times more likely to suffer a lower limb amputation than are members of the general population.
Both diabetes and gout show positive correlation with chronic renal failure (genitourinary category), although the relationship is much stronger for diabetes (a 0.24 posterior mean correlation, versus 0.11 for gout). Diabetes is in fact one of the most common causes of kidney disease, and in the case of gout, untreated kidney stones can lead to a chronic form of kidney disease. Overall, diabetes is slightly more likely to appear as a secondary diagnosis; and for 20% of those claims, the primary diagnosis is chronic renal failure.
Diabetes has positive correlation with multiple diagnoses in the nervous system/sense organs category, including other retina disorders (0.34), diabetic/other peripheral neuropathies (0.26), blindness/low vision (0.13), visual disturbances (0.12), and glaucoma (0.11). Overall, about 11% of diabetes diagnoses combine with a disorder of the nervous system/sense organs. Gout is also associated with visual disturbances. Finally, diabetes has positive correlation with other disorders of the skin, while gout is associated with symptomatic HIV.21
Correlations Involving the Nervous System and Sense Organs
Many impairments in the nervous system/sense organs category show positive correlations with one another. Table 27 summarizes some of the findings. Impairments with posterior mean correlations higher than 0.30 include blindness/low vision with other retina disorders and with glaucoma, glaucoma with other retina disorders, and vertiginous syndromes with deafness. Mean correlations higher than 0.20 include those of visual disturbances with blindness/low vision, glaucoma, and other retina disorders.
Impairment code and diagnosis | Correlation (posterior mean) | t-statistic | Numerical standard error |
---|---|---|---|
3690 - Blindness and low vision and— | |||
3620 - Other retina disorders | 0.3551 | 12.68 | 0.003300 |
3650 - Glaucoma | 0.3469 | 13.15 | 0.002561 |
3680 - Visual disturbances | 0.2401 | 9.23 | 0.001843 |
3650 - Glaucoma and— | |||
3620 - Other retina disorders | 0.3379 | 9.49 | 0.004095 |
3680 - Visual disturbances | 0.2798 | 7.69 | 0.004251 |
3890 - Deafness | 0.1260 | 2.79 | 0.005340 |
3460 - Migraine | 0.1112 | 2.62 | 0.004500 |
3860 - Vertiginous syndromes and— | |||
3890 - Deafness | 0.3128 | 9.94 | 0.003480 |
3460 - Migraine | 0.1561 | 3.79 | 0.004333 |
3620 - Other retina disorders and— | |||
3680 - Visual disturbances | 0.2140 | 5.34 | 0.003879 |
3570 - Diabetic and other peripheral neuropathy | 0.1963 | 6.86 | 0.002564 |
3490 - Other nervous system disorders and— | |||
3460 - Migraine | 0.1621 | 6.79 | 0.001263 |
3310 - Other cerebral degenerations | 0.1449 | 3.29 | 0.004983 |
3360 - Other spinal cord disorders | 0.1263 | 3.03 | 0.004456 |
3360 - Other spinal cord disorders and— | |||
3350 - Anterior horn cell disease | 0.1617 | 2.80 | 0.008310 |
3450 - Epilepsy and— | |||
3460 - Migraine | 0.1573 | 8.17 | 0.001229 |
3310 - Other cerebral degenerations | 0.1058 | 2.99 | 0.003530 |
3310 - Other cerebral degenerations and— | |||
3350 - Anterior horn cell disease | 0.1554 | 2.55 | 0.008918 |
3360 - Other spinal cord disorders | 0.1217 | 2.05 | 0.007097 |
3460 - Migraine | 0.1136 | 2.47 | 0.005674 |
3400 - Multiple sclerosis | 0.1062 | 2.45 | 0.004962 |
3430 - Cerebral palsy and— | |||
3590 - Muscular dystrophies | 0.1530 | 2.24 | 0.010282 |
3680 - Visual disturbances and— | |||
3400 - Multiple sclerosis | 0.1521 | 5.51 | 0.002408 |
3460 - Migraine | 0.1039 | 3.37 | 0.002375 |
9070 - Late effects of injuries to the nervous system and— | |||
3310 - Other cerebral degenerations | 0.1479 | 3.19 | 0.005748 |
3360 - Other spinal cord disorders | 0.1303 | 2.35 | 0.006539 |
3350 - Anterior horn cell disease | 0.1221 | 2.09 | 0.007216 |
3580 - Myoneural disorders and— | |||
3540 - Carpal tunnel syndrome | 0.1274 | 2.64 | 0.005614 |
SOURCE: Author's calculations using MVP estimation model fitted to a DRF 10% random sample. | |||
NOTES: Correlation estimates are selected for inclusion on the basis of having absolute values for the posterior mean of at least 0.10 and, generally, a t-statistic greater than 2.00.
Data are for applicants who cleared step 1 of the disability determination process.
Diagnoses are arranged by estimated correlation value.
Some diagnoses are identified with a shortened version of their official designation.
Diagnoses identified as "other" may be defined in the context of impairments not shown in this table.
|
Other estimates with relatively high correlation magnitudes include vertiginous syndrome with migraine; diabetic/other peripheral neuropathies with other retina disorders; migraine with epilepsy and with other disorders of the nervous system; visual disturbances with multiple sclerosis; cerebral palsy with muscular dystrophy; and anterior horn cell disease with both other cerebral degenerations and other diseases of the spinal cord. In 15.7% of secondary diagnoses involving the nervous system/sense organs, the primary diagnosis is in the same category.
Nervous System and Other Impairments
As noted earlier, many impairments of the nervous system/sense organs correlate positively with certain specific diagnoses from other body systems; those diagnoses include brain cancers, organic mental disorders, late effects of cerebrovascular disease, diabetes, and diffuse diseases of the connective tissue. Table 28 summarizes the other diagnoses with relevant correlation findings. Several of the disorders classified as injuries show significant positive association. Intracranial injury and fracture of the vertebral column have posterior mean correlations with late effects of injuries to the nervous system of 0.30 and 0.21, respectively. Intracranial injuries also display positive correlations with other cerebral degenerations, visual disturbances, and migraines, while a fracture of the vertebral column has additional positive correlations with other disorders of the spinal cord, anterior horn cell disease, and muscular dystrophies. In addition, other retina disorders and diabetic/other peripheral neuropathies are further associated with late effects of injury to the musculoskeletal/connective tissue (another injury diagnosis).
Diagnostic group, impairment code, and diagnosis | Correlation (posterior mean) | t-statistic | Numerical standard error |
---|---|---|---|
9070 - Late effects of injuries to the nervous system and— | |||
Injuries | |||
8540 - Intracranial (traumatic brain) injury | 0.2995 | 9.29 | 0.002815 |
8060 - Fracture of vertebral column | 0.2126 | 4.41 | 0.007312 |
3360 - Other spinal cord disorders and— | |||
Injuries | |||
8060 - Fracture of vertebral column | 0.1977 | 3.74 | 0.007003 |
3620 - Other retina disorders and— | |||
Genitourinary system diseases | |||
5850 - Chronic renal failure | 0.1901 | 6.70 | 0.004750 |
Injuries | |||
9050 - Late effects of musculoskeletal and connective tissue injuries (amputation) | 0.1125 | 3.10 | 0.003793 |
3310 - Other cerebral degenerations and— | |||
Injuries | |||
8540 - Intracranial (traumatic brain) injury | 0.1722 | 3.44 | 0.006251 |
3650 - Glaucoma and— | |||
Infectious and parasitic diseases | |||
1350 - Sarcoidosis | 0.1700 | 3.24 | 0.006435 |
3350 - Anterior horn cell disease and— | |||
Genitourinary system diseases | |||
5850 - Chronic renal failure | 0.1652 | 4.20 | 0.004161 |
Injuries | |||
8060 - Fracture of vertebral column | 0.1611 | 2.40 | 0.009499 |
3590 - Muscular dystrophies and— | |||
Injuries | |||
8060 - Fracture of vertebral column | 0.1408 | 2.62 | 0.007125 |
3460 - Migraine and— | |||
Injuries | |||
8540 - Intracranial (traumatic brain) injury | 0.1147 | 3.03 | 0.004006 |
3680 - Visual disturbances and— | |||
Injuries | |||
8540 - Intracranial (traumatic brain) injury | 0.1129 | 2.87 | 0.003472 |
3860 - Vertiginous syndromes and— | |||
Skin and subcutaneous tissue diseases | |||
7090 - Other skin and subcutaneous tissue disorders | 0.1124 | 2.26 | 0.005687 |
3430 - Cerebral palsy and— | |||
Genitourinary system diseases | |||
5850 - Chronic renal failure | 0.1092 | 2.75 | 0.004750 |
3570 - Diabetic and other peripheral neuropathy and— | |||
Injuries | |||
9050 - Late effects of musculoskeletal and connective tissue injuries (amputation) | 0.1077 | 4.11 | 0.001764 |
Genitourinary system diseases | |||
5850 - Chronic renal failure | 0.1069 | 5.26 | 0.001050 |
SOURCE: Author's calculations using MVP estimation model fitted to a DRF 10% random sample. | |||
NOTES: Correlation estimates are selected for inclusion on the basis of having absolute values for the posterior mean of at least 0.10 and, generally, a t-statistic greater than 2.00.
Data are for applicants who cleared step 1 of the disability determination process.
Diagnoses are arranged by estimated correlation value.
Diagnoses identified as "other" may be defined in the context of impairments not shown in this table.
|
In the genitourinary category, chronic renal failure exhibits positive correlation with several neurological/sense organ impairments such as other retina disorders (0.19), anterior horn cell disease (0.17), cerebral palsy (0.11), and diabetic/other peripheral neuropathies (0.11). Sarcoidosis, an infectious/parasitic disease which often has ophthalmologic manifestations, shows a mean correlation of 0.17 with glaucoma. Finally, there is positive association between vertiginous syndromes and a diagnosis of other disorders of the skin.
Correlations Involving Injuries
Many injury diagnoses are positively associated with one another. In 20.4% of claims with an injury as a secondary diagnosis, the primary impairment is another injury. The findings appear in Table 29. The highest estimated correlations, with mean values higher than 0.20, associate fractures of a lower limb with fractures of an upper limb (0.33), fractures of the lower limb with other fractures of bones (0.27), fractures of a lower limb with dislocations of all types (0.20), and fractures of an upper limb with other fractures of bones (0.29). In total, Table 29 identifies 22 statistically significant correlations among the injury diagnoses.
Impairment code and diagnosis | Correlation (posterior mean) | t-statistic | Numerical standard error |
---|---|---|---|
8270 - Fractures of lower limb and— | |||
8180 - Fractures of upper limb | 0.3307 | 20.04 | 0.001005 |
8290 - Other fractures of bones | 0.2652 | 13.37 | 0.001092 |
8390 - Dislocations—all types | 0.2026 | 6.00 | 0.002771 |
8940 - Open soft tissue wound of lower limb | 0.1898 | 5.56 | 0.003417 |
8060 - Fracture of vertebral column | 0.1345 | 3.93 | 0.003206 |
9050 - Late effects of musculoskeletal and connective tissue injuries (amputation) | 0.1208 | 5.31 | 0.001005 |
8180 - Fractures of upper limb and— | |||
8290 - Other fractures of bones | 0.2870 | 12.14 | 0.001805 |
8390 - Dislocations—all types | 0.1805 | 4.21 | 0.004954 |
8840 - Open soft tissue wound of upper limb | 0.1459 | 3.39 | 0.005223 |
9050 - Late effects of musculoskeletal and connective tissue injuries (amputation) | 0.1427 | 5.41 | 0.002173 |
8060 - Fracture of vertebral column | 0.1156 | 3.32 | 0.005675 |
8540 - Intracranial (traumatic brain) injury | 0.1156 | 3.32 | 0.003565 |
8940 - Open soft tissue wound of lower limb | 0.1030 | 2.35 | 0.003972 |
8480 - Sprains and strains—all types | 0.1006 | 3.75 | 0.002311 |
8540 - Intracranial (traumatic brain) injury and— | |||
8060 - Fracture of vertebral column | 0.1875 | 4.47 | 0.004695 |
8940 - Open soft tissue wound of lower limb and— | |||
8840 - Open soft tissue wound of upper limb | 0.1757 | 3.19 | 0.007194 |
9050 - Late effects of musculoskeletal and connective tissue injuries (amputation) | 0.1001 | 2.23 | 0.004878 |
8290 - Other fractures of bones and— | |||
8540 - Intracranial (traumatic brain) injury | 0.1430 | 3.46 | 0.005244 |
8060 - Fracture of vertebral column | 0.1329 | 3.12 | 0.004380 |
8390 - Dislocations—all types | 0.1328 | 2.53 | 0.006754 |
8390 - Dislocations—all types and— | |||
8940 - Open soft tissue wound of lower limb | 0.1222 | 1.90 | 0.009346 |
9050 - Late effects of musculoskeletal and connective tissue injuries (amputation) | 0.1009 | 2.20 | 0.005590 |
SOURCE: Author's calculations using MVP estimation model fitted to a DRF 10% random sample. | |||
NOTES: Correlation estimates are selected for inclusion on the basis of having absolute values for the posterior mean of at least 0.10 and, generally, a t-statistic greater than 2.00.
Data are for applicants who cleared step 1 of the disability determination process.
Diagnoses are arranged by estimated correlation value.
Diagnoses identified as "other" may be defined in the context of impairments not shown in this table.
|
Most of the highest associations between injuries and diagnoses from other categories were discussed earlier. To summarize, intracranial injury has positive correlation with malignant and benign brain cancers (neoplasms), organic mental disorders (mental impairments), late effects of cerebrovascular disease (circulatory system), and a host of impairments of the nervous system/sense organs. Fractures of the vertebral column are positively associated with various cancers. Several injuries have positive correlations with peripheral vascular disease and other circulatory system diagnoses, with other disorders of the bone and cartilage (musculoskeletal system), and with various impairments of the nervous system/sense organs. Finally, open wounds of a lower limb and late effects of musculoskeletal/connective tissue injuries are associated with diabetes.
Other Statistically Significant Estimated Correlations
Table 30 lists all significant correlation estimates not shown in the preceding tables. Chronic renal failure (genitourinary system) has posterior mean correlations of 0.12 with symptomatic HIV and 0.14 with fractures of the vertebral column. The link between HIV and chronic kidney disease is well-established. In addition, patients with chronic kidney disease can suffer complications involving mineral and bone disorders (Nikolov, Ivanovski, and Joki 2012).
Diagnostic group, impairment code, and diagnosis | Correlation (posterior mean) | t-statistic | Numerical standard error |
---|---|---|---|
5690 - Other gastrointestinal system disorders and— | |||
Digestive system diseases | |||
5550 - Crohn's disease | 0.2643 | 10.27 | 0.001928 |
5530 - Hernias | 0.1938 | 6.07 | 0.003013 |
5710 - Chronic liver disease and cirrhosis | 0.1623 | 7.92 | 0.001174 |
Genitourinary system diseases | |||
5990 - Other urinary tract disorders | 0.1277 | 3.07 | 0.004544 |
5710 - Chronic liver disease and cirrhosis and— | |||
Infectious and parasitic diseases | |||
0440 - Asymptomatic HIV | 0.1836 | 6.69 | 0.002186 |
0430 - Symptomatic HIV | 0.1486 | 5.94 | 0.001796 |
Digestive system diseases | |||
5530 - Hernias | 0.1220 | 3.80 | 0.003173 |
Genitourinary system diseases | |||
5850 - Chronic renal failure | 0.1151 | 6.01 | 0.000984 |
5530 - Hernias and— | |||
Genitourinary system diseases | |||
5990 - Other urinary tract disorders | 0.1563 | 3.43 | 0.005377 |
5850 - Chronic renal failure and— | |||
Injuries | |||
8060 - Fractures of the vertebral column | 0.1437 | 4.29 | 0.003436 |
Infectious and parasitic diseases | |||
0430 - Symptomatic HIV | 0.1161 | 4.44 | 0.001860 |
7090 - Other skin and subcutaneous tissue disorders and— | |||
Digestive system diseases | |||
5690 - Other disorders of gastrointestinal system | 0.1124 | 2.97 | 0.004187 |
5710 - Chronic liver disease and cirrhosis | 0.1114 | 2.92 | 0.003813 |
SOURCE: Author's calculations using MVP estimation model fitted to a DRF 10% random sample. | |||
NOTES: Correlation estimates are selected for inclusion on the basis of having absolute values for the posterior mean of at least 0.10 and, generally, a t-statistic greater than 2.00.
Data are for applicants who cleared step 1 of the disability determination process.
Diagnoses are arranged by estimated correlation value.
Diagnoses identified as "other" may be defined in the context of impairments not shown in this table.
|
Many of the entries in Table 30 involve a digestive system diagnosis. The catch-all diagnosis of other disorders of the gastrointestinal system exhibits positive correlations with the digestive impairments Crohn's disease (0.26), hernias (0.19), and chronic liver disease/cirrhosis (0.16), and the catch-all genitourinary impairment other disorders of the urinary tract (0.13). Chronic liver disease/cirrhosis shows positive correlations with both symptomatic and asymptomatic HIV (infectious/parasitic diseases), with hernias (digestive system), with chronic renal failure (genitourinary system), as well as with other disorders of the skin (skin/subcutaneous tissue category). Finally, hernias have a posterior mean correlation of 0.16 with a diagnosis of other disorders of the urinary tract, and other disorders of the gastrointestinal system are correlated with other disorders of the skin.
Summary
This paper finds evidence of strong impairment comorbidity patterns among individuals filing initial claims to the Social Security disability programs in 2009. Understanding which combinations of impairments appear frequently in claims for disability benefits, and which combinations do not, is useful for a number of reasons. First, ignoring the secondary impairments yields a distorted picture of diagnostic incidence. Second, many epidemiological studies have found a significant effect of multimorbidity on the likelihood of disability, poor quality of life, and high health care costs. Third, the secondary diagnoses can improve the out-of-sample prediction of disability determination outcomes. Thus, a better understanding of impairment comorbidity in DI and SSI is important. A summary of the main findings follows:
- From the disability programs' perspective, the percentage of claimants with an assigned secondary diagnosis has steadily increased over time. In 1997, a little over half of them (56.3%) had a secondary diagnosis code. By 2010, that share had increased to 71.4%.
- The individual diagnoses show a great deal of heterogeneity in their initial allowance rates, sex composition, peak age of onset, and relative incidence. The two most common individual impairments (affective/mood disorders and disorders of the back) dwarf the remaining diagnoses in frequency.
- Male claimants are somewhat older and experience higher initial allowance rates. They have a higher incidence of diagnoses of the circulatory system, injuries, infectious/parasitic diseases (in which symptomatic HIV is the most common impairment), and digestive impairments (because of the influence of chronic liver disease/cirrhosis). Men also dominate cases with diagnoses in the genitourinary category, in which chronic renal failure is the most common. Women have higher shares of cases with mental-disorder diagnoses, respiratory impairments, and malignant neoplasms (breast cancer is the second most commonly diagnosed neoplasm overall).
- Relative to male claimants, women dominate cases of affective/mood disorders and anxiety disorders, which together constitute 22.8% of all mentioned primary and secondary diagnoses. As a result, any policy that would make the disability determination process for mental impairments more stringent could end in a disproportionately larger share of women being denied or delayed eventual entry into the disability rolls.
- Filers of concurrent DI and SSI claims are substantially younger than DI-only applicants (with a median age difference of 8 years). Contributing to the wide gap in initial allowance rates between the two groups is their different diagnostic composition. Concurrent-claim applicants have fewer cancers and impairments of the nervous system/sense organs. They also have fewer of the high allowance-rate circulatory impairments, but more cases of essential hypertension, which is associated with a low allowance rate. On the other hand, concurrent-claim applicants have higher shares of chronic liver disease/cirrhosis, HIV infection, and mental disorder diagnoses (including more than three-quarters of the cases involving addiction, personality disorders, and schizophrenia).
- Claimants who have applied to the DI program in the past and who subsequently reapply to DI are more likely to apply simultaneously to both DI and SSI than to apply to DI alone. It is likely that over time, denied unemployed claimants consume enough of their savings and assets to meet the SSI means test as well.
- The estimated correlation between two impairments must be understood in terms of their relative frequency in the sample. A high positive correlation suggests that the two impairments combine far more often than expected, given their overall frequencies.
- One cluster of impairments from five different diagnostic groups exhibits high positive correlation. Those impairments are (1) organic mental disorders; (2) intracranial injury; (3) malignant and benign neoplasms of the brain; (4) late effects of cerebrovascular disease (a circulatory impairment); and (5) several disorders of the nervous system/sense organs, such as epilepsy, migraine, late effects of injuries to the nervous system, other cerebral degenerations, and visual disturbances. Diagnoses between the five groups show a strong degree of association with one another.
- Malignant neoplasms tend to have strong patterns of comorbidity with one another, presumably resulting from metastasis. More than half of the cancers listed as secondary diagnoses combine with another neoplasm as the primary diagnosis. Various cancers show positive association with chronic renal failure, symptomatic HIV, muscular dystrophies, chronic liver disease/cirrhosis, and several disorders of the spine such as fractures of the vertebral column, anterior horn cell disease, and other spinal cord disorders. Various cancers are also positively correlated with schizophrenia, autistic disorders, and intellectual disability. On the other hand, most of the cancers have significant negative correlation estimates with disorders of the back and affective/mood disorders.
- Although 45.7% of mental-impairment primary diagnoses combine with a mental-impairment secondary diagnosis, the correlation estimates reveal two distinct groups of impairments. The impairments in each group tend to be negatively correlated with those in the other group but are often positively correlated with their intragroup counterparts. One group includes affective/mood diagnoses, anxiety/personality disorders, schizophrenia, and substance addiction. The other group comprises early developmental cognitive disorders such as autism, borderline intellectual functioning, intellectual disability, and learning/attention deficit disorders.
- The two most common impairments (affective/mood diagnoses and disorders of the back) combine less frequently than one would expect, based on their relative shares of diagnoses overall. In fact, at the initial-claim level, the correlation estimates contradict presumptive expectations of high comorbidity between mental and musculoskeletal diagnoses. Affective/mood disorders have negative estimated correlations with many of the musculoskeletal diagnoses (osteoarthrosis/allied disorders; disorders of the muscle/ligament/fascia; diffuse diseases of the connective tissue; other diseases of the bone/cartilage; other/unspecified arthropathies; and curvature of the spine). Conversely, disorders of the back show statistically significant negative correlation with schizophrenia, personality/anxiety disorders, organic mental impairments, and even addiction.
- The comorbidity of substance abuse and other psychiatric disorders is well known. Alcohol and drug addiction combine to represent 1.8% of all primary and secondary diagnoses. In the large majority of cases, addiction combines with another mental impairment (66.7% and 78.6%, respectively, for alcohol and drugs). The addiction codes are also associated with chronic liver disease/cirrhosis, for obvious reasons.
- Most of the circulatory diagnoses have high positive correlations with one another. In 23.4% of claims with the primary diagnosis of a circulatory impairment, the secondary diagnosis is another circulatory disorder. Several circulatory impairments (particularly chronic pulmonary heart disease and heart failure) have positive associations with various respiratory disorders. A circulatory impairment coincides with 13% of obesity diagnoses and 25% of diabetes diagnoses (two endocrine/nutritional/metabolic impairments) and with 24% of chronic renal failure diagnoses (a genitourinary impairment). A few specific circulatory diagnoses, such as peripheral vascular disease, are positively correlated with various injuries such as open wounds to a lower limb and late effects of musculoskeletal/connective tissue injury (amputation).
- Overall, 14% of musculoskeletal system diagnoses combine with another musculoskeletal impairment. Most of the highest estimated absolute-value correlations involving the two most common musculoskeletal diagnoses (disorders of the back and osteoarthrosis) are negative, although there are a few exceptions. For example, osteoarthrosis and obesity exhibit a high positive correlation. Disorders of the back, disorders of the muscle/ligament/fascia, and diffuse diseases of the connective tissue all have positive associations with carpal tunnel syndrome. A diagnosis of other disorders of the bone/cartilage is also associated with two kinds of injuries (dislocations and other fractures of bones). Finally, the only two musculoskeletal impairments with high positive correlation with one another are rheumatoid arthritis and diffuse diseases of the connective tissue, both of which are much more likely to be diagnosed for female claimants. A diagnosis of diffuse disease of the connective tissue also has positive associations with chronic renal failure and muscular dystrophies.
- Although respiratory disorders constitute only 3.7% of all diagnoses, 8.3% of respiratory impairments cited as a primary diagnosis combine with a secondary respiratory disorder, suggesting some degree of comorbidity (particularly for the combination of chronic pulmonary insufficiency and the catch-all diagnosis of other respiratory system disorders). There is very strong positive association of sleep-related breathing disorders with obesity, and of several respiratory impairments with sarcoidosis (an infectious/parasitic disease). In addition, a diagnosis of other disorders of the respiratory system is positively associated with symptomatic HIV, obesity, muscular dystrophies, other disorders of the gastrointestinal system, and Crohn's disease.
- Diabetes is the fifth most common diagnosis overall. It is the leading cause of blindness, limb amputation, and kidney dialysis in the United States, a fact that appears to be mirrored by its association with those and other impairments. About 11% of diabetes diagnoses combine with a disorder of the nervous system/sense organs such as other retina disorders, diabetic/other peripheral neuropathies, blindness/low vision, and visual disturbances, among others. In 20% of the claims for which diabetes is the secondary diagnosis, chronic renal failure is the primary diagnosis. In addition, diabetes is positively associated with injuries such as open wounds of a lower limb and amputations (late effects of musculoskeletal/connective tissue injuries).
- Many of the impairments in the nervous system/sense organs category are positively correlated with one another. In 15.7% of secondary diagnoses involving the nervous system/sense organs, the primary diagnosis is in the same category. Some of the highest correlations are for blindness/low vision with other retina disorders and glaucoma; for glaucoma with other retina disorders; and for vertiginous syndromes with deafness. Several of the disorders classified as injuries also show significant positive associations. Intracranial injury has positive correlation with late effects of injuries to the nervous system, other cerebral degenerations, visual disturbances, and migraines. Fractures of the vertebral column are associated with late effects of injuries to the nervous system, other diseases of the spinal cord, anterior horn cell disease, and muscular dystrophies. Chronic renal failure also exhibits positive correlation with several disorders of the nervous system/sense organs.
- Diagnoses in the digestive-system category exhibit some comorbidity with one another, as well as with impairments of the genitourinary system and infectious/parasitic disease groups. The catch-all diagnosis of other disorders of the gastrointestinal system has positive correlation with Crohn's disease, hernias, chronic liver disease/cirrhosis, and other disorders of the urinary tract. Chronic liver disease/cirrhosis shows positive correlation with both symptomatic and asymptomatic HIV (infectious/parasitic diseases), with hernias (another digestive system impairment), and with chronic renal failure (a genitourinary system impairment). Symptomatic HIV is also associated with chronic renal failure.
Appendix
The MVP Model
Let = (yi1, yi 2,…, yip) denote a vector of binary responses corresponding to the th individual or observation ( = 1, 2,…, ), and let = (z i1, z i 2,…, zip) represent a normally distributed latent variable defined by the following relationship:
Here, I consider the general case of covariates (including intercepts) that are allowed to have different coefficients for the responses, so that is a dimensional matrix, where is a vector of covariates corresponding to the th observation. In addition, represents an identity matrix and is the dimensional vector of coefficients associated with the explanatory variables and responses.
In the MVP model, the probability of observing response , given the parameters and covariates , is defined by
where is the density of a -variate normal distribution with
This parametrization of the model in terms of a covariance matrix is not likelihood-identified, as it is possible to scale the mean of each by a positive constant without changing the observed binary data. In other words, only the correlation matrix of is identified. Thus, the identification restrictions imposed typically involve22
where is a correlation matrix with unique elements, represents a matrix comprising the rearranged coefficients, and is the following diagonal matrix:
with denoting the th diagonal element in .
The observed data-likelihood in the MVP model is the product of the various probabilities associated with :
Its evaluation is only feasible if the -dimensional integrals involved can be calculated accurately, which is notoriously difficult for large . In practice, numerical integration techniques such as quadrature are feasible when is less than or equal to three or four. For larger dimensional problems, because of the computational burden, researchers have often turned to assuming restrictive structures for the correlation matrix that can more easily approximate the multivariate normal probabilities.
An alternative approach, known as dimensionality reduction, involves the use of exploratory factor analysis models. For example, Gibbons and Wilcox-Gök (1998) assume a correlation matrix with the following form
where is a matrix of factor loadings and is a diagonal matrix. If a small number of latent factors can adequately model the correlation among the choices, then the dimension of the required integrals is substantially reduced.
Exploiting the latent variable formulation of discrete dependent-variable models enables the design of more effective estimation procedures via data augmentation. Notice, for example, that the complete-data likelihood that results from augmenting the binary outcomes with the latent variables in the MVP model is
where denotes the indicator function. This complete-data likelihood is reminiscent of the likelihood function in the standard normal linear model and provides the basis for maximum-likelihood estimation via some variant of the Monte Carlo Expectation-Maximization algorithm (Chib and Greenberg 1998; Xu and Craig 2010). The complete-data likelihood is also at the heart of Bayesian estimation of the model's parameters using the Gibbs sampler.
From a Bayesian standpoint, Albert and Chib (1993) pioneered estimation of the binary probit model using Gibbs sampling with data augmentation. Again, the basic insight is to augment the observed binary data with the latent variables using a scheme for easily generating a random sample of the joint distribution of and , bypassing the need to explicitly evaluate the integral probabilities in the observed data-likelihood. McCulloch and Rossi (1994) generalized the approach to the multinomial probit (MNP) model, which is similar in structure to the MVP, but it employs a different mechanism for censoring the latent variables because the responses in this case are mutually exclusive.23
The sampler proposed by McCulloch and Rossi (1994) for the MNP model relies on a proper but diffuse prior specification of the unidentified parameter space. This is a unique feature of Bayesian inference using MCMC algorithms. As long as a proper prior is specified, the identification restrictions need not be imposed to estimate the model. The algorithm simply navigates the unidentified space and the resulting sample is postprocessed to estimate the identified posterior quantities of interest. Nobile (1998) suggested adding a Metropolis step at each iteration of the Gibbs chain as a way to improve convergence in some pathological cases. McCulloch, Polson, and Rossi (2000) proposed an alternative sampler that traverses the identified parameter space, but that approach results in slower convergence than does the original algorithm of McCulloch and Rossi (1994). In fact, one of the advantages of defining a sampler over the higher-dimensional unidentified parameter space is that it generally yields better mixing properties than does a chain traversing the identified space. In practice, this means more accurate posterior estimates for a fixed number of simulated iterations.
From a computational perspective, the identification constraints in the MVP model are more problematic than those in the MNP model. This is because sampling the covariance matrix can be straightforward, relative to the correlation matrix . In addition to the symmetry and positive definiteness of the draws, the individual elements of must be constrained in value to the interval [−1, 1]. In the context of the MVP model, Chib and Greenberg (1998) proposed a Gibbs sampler on the identified parameter space using a Metropolis-Hastings step to generate candidate draws of the correlation matrix. However, in high-dimensional problems, many draws of typically have to be produced before an adequate candidate is accepted, suggesting a slow converging algorithm. Barnard, McCulloch, and Meng (2000) sidestep this problem via the Griddy-Gibbs sampler, by sequentially simulating every element of the correlation matrix, subject to the appropriate constraints. Again, however, the method is cumbersome and computationally taxing for large .
The sampler used for this paper follows Edwards and Allenby (2003). It specifies the following conjugate prior on the full set of unidentified parameters:
leading to standard conjugate results. This Gibbs sampling algorithm involves sequentially drawing from a multivariate normal distribution, drawing from an inverse Whishart distribution, and drawing the latent variables from a truncated multivariate normal distribution. The latter is accomplished by sequentially simulating univariate truncated draws. Rossi, Allenby, and McCulloch (2005) provide further implementation details.24 The authors also point out that improper priors on lead to a peculiar trade-off, in that they produce a sampler with better mixing properties, but can be very informative in terms of the implied correlations, placing high probability mass on the tails. Following the recommendations in Rossi, Allenby, and McCulloch (2005), I assign the following values to the prior hyperparameters:
resulting in a proper but sufficiently diffuse prior. The size of the fitted data (157,835 observations) further rules out any undue prior influence on the resulting posterior estimates. A single Gibbs chain is then simulated, using zeroes as starting values for the regression coefficients and an initial identity matrix for the covariance .
Sampling Performance
The MVP model offers several advantages over alternative approaches, including better sampling performance. For example, Pearson's correlation estimator has a known downward bias when applied to binomial data. This is evident in Chart A-1, which presents a scatter plot of the posterior mean correlation estimates from the MVP model against corresponding Pearson's correlation coefficients. Evidently, the two sets of estimates vary widely, as few points in the graph fall on the 45-degree line. Consistent with their downward bias, Pearson's correlation coefficients cluster closely around zero. By contrast, a substantial portion of the posterior means exceed 0.1 in absolute magnitude, suggesting strong patterns of association among many of the impairments.
Pearson's correlation estimates versus Bayesian posterior means
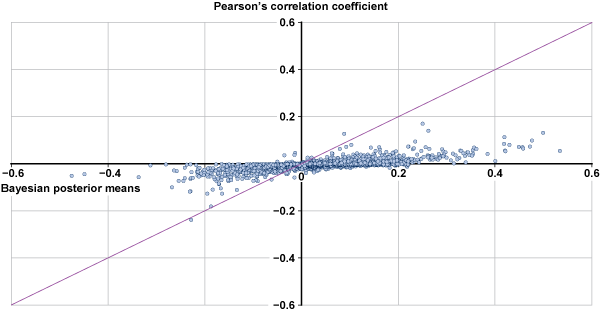
An alternative to Pearson's correlation coefficients is a likelihood-based tetrachoric correlation estimator, which does not suffer from the downward bias problem. Edwards and Allenby (2003) conducted a simulation study investigating the small sample properties of their Bayes estimator. Their findings indicated consistent improvement (lower mean squared error) compared to the tetrachoric approach. The authors further illustrated one important drawback of the tetrachoric estimator shared by other estimation approaches: When the pairwise correlation estimates are collected to form a square symmetric matrix, there is no guarantee of positive definiteness. This is important because the full correlation matrix is required to simulate any probabilities of interest. In addition, neither Pearson's nor the tetrachoric-based estimators can accommodate exogenous predictors.
Dimensionality Reduction
An alternative to the computationally demanding endeavor of estimating a high-dimensional MVP model is to rely on exploratory factor analysis. Such an approach rests on the assumption that a small number of latent factors can explain most of the variation in the data. I explore this possibility by performing principal-component analysis on the posterior mean of the MVP model's correlation matrix. Chart A-2 displays a scatter plot of the component loading associated with the first two eigenvectors. In observing the horizontal range of plotted coordinates, notice that the musculoskeletal impairments, which have low allowance rates, tend to appear on the left side of the graph—particularly so for disorders of the back. Circulatory diagnoses and injuries tend to cluster in the middle, while neoplasms, with some of the highest allowance rates, appear further to the right. The rightmost point in the plot corresponds to the binary variable indicating an initial allowance. Thus, it seems that the first principal component is associated with the initial allowance rate.
Principal components of the posterior mean correlation matrix
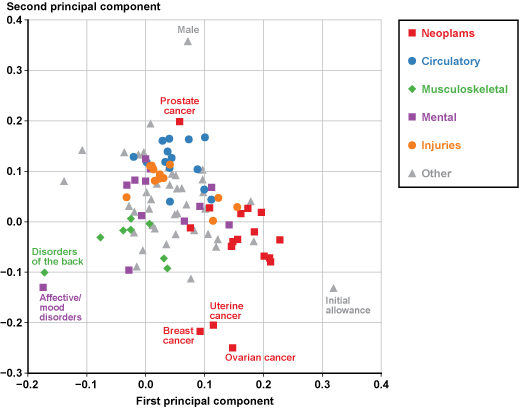
In observing the vertical range, note that the highest plotted coordinate in Chart A-2 corresponds to the binary variable indicating sex (a value of 1 for men). Prostate cancer, many of the circulatory system diagnoses, and many of the injuries (which are more prevalent among male applicants) also appear near the upper part of the vertical axis. Conversely, malignant neoplasms of the breast, ovary, and uterus take the lowest values of the second principal component. Affective/mood disorders, which are more prevalent among women, appear as the lowest plotted purple square in the graph. From this distribution, it seems that the second principal component tends to be associated with the sex prevalence of the diagnoses.
Based on Chart A-2, it might appear that dimensionality reduction could be a viable alternative to our modeling approach, which entertains a fully unrestricted correlation specification. However, the first and second principal components combine to explain less than 10% of total variance. Moreover, 34 of the 104 eigenvalues exceed one, accounting for 57% of the variance. It would take the use of 27 and 56 principal components, respectively, to explain one-half and three-fourths of the variation in the data. In this context, it is clear that a small-scale latent factor model would be a poor substitute for the estimation approach pursued in this paper.25
Evaluating the Impact of the Correlations on Prediction
In practice, given the identified posterior parameter estimates, any conditional probability can be calculated by simulating a random sample of multivariate normal draws. The relative frequency of the outcomes observed in the generated latent variables serves as an estimate of the conditional probability of interest. Table A-1 illustrates the use of conditional probabilities. For the fitted data (the 10% random sample of 2009 claimants), the top panel of the table reports the percentages of initial allowances, initial denials, and all initial determinations that are correctly predicted by the model. Conditional on each claimant's sex, concurrent-claim status, application history, age, and combination of impairments, I use the model to compute the mean probability of an initial allowance. If the predicted probability exceeds 0.5 and the actual determination was an initial allowance (or if the probability is below 0.5 and the claimant received an initial denial), I count the observation as correctly predicted. As seen in Table A-1, the estimated model correctly predicts 72.6% of the initial determinations in the fitted sample, including 53.6% of the allowances and 84.3% of the denials.
Model | All | Allowances | Denials |
---|---|---|---|
10% random sample (157,835 observations) | |||
Full MVP model | 72.59 | 53.55 | 84.27 |
MVP model zero correlation | 66.87 | 42.97 | 81.53 |
All 2009 applicants (1,578,354 observations) | |||
Full MVP model | 71.95 | 51.93 | 84.18 |
MVP model zero correlation | 66.49 | 42.50 | 81.13 |
All 2001 applicants (1,141,717 observations) | |||
Full MVP model | 71.17 | 55.87 | 81.54 |
MVP model zero correlation | 63.75 | 38.47 | 80.87 |
SOURCE: Author's calculations using DRF and MVP estimation model. |
The second row in the top panel of Table A-1 provides prediction estimates based on the erroneous assumption of zero correlation among the binary variables. In other words, I use the posterior values of the regression coefficients, but I substitute an identity correlation matrix to generate the simulations required to estimate the conditional probabilities. The rationale for this approach is to try to isolate the net effect of the estimated correlations in the prediction process. As expected, the accuracy of the predictions deteriorates significantly when the estimated correlation patterns are ignored. In this case, only 66.9% of the determinations are predicted correctly.
The second panel of Table A-1 extends the prediction exercise to all 2009 claimants (1,578,354 records). The findings are similar to those for the 10% random sample used to fit the model. Ignoring the correlation estimates yields correct predictions for 66.5% of the claimants, while the full model correctly predicts 71.9% of all initial determinations.
I also tested the out-of-sample predictive ability of the model fitted with 2009 data, applying its predictions to a set of claimants from 8 years earlier. I used the same filtering mechanism to identify workers filing claims in 2001 and diagnosed with impairments that were included among the 100 diagnoses considered in this application, yielding 1,141,717 records. The results appear in the third panel of Table A-1. The estimated MVP model successfully classifies 71.2% of the initial determinations received by all 2001 claimants. Surprisingly, the model correctly predicts a higher share of initial allowances (55.9%) for the 2001 claimants than for the fitted data corresponding to 2009. Meanwhile, the predictive ability of the zero-correlation assumption is notably diminished, with only 38.5% of allowances classified accurately.
MCMC Convergence
MCMC samplers involve indirect simulations of draws from an often-complex multivariate target distribution by generating a Markov chain whose stationary density is the target distribution. The draws from the Markov chain can be used to approximate any features of the target density, which in a Bayesian context is the posterior density of interest. In practice, the algorithm is started at some arbitrary point in the parameter space. Thus, in order to allow for the influence of the initial conditions to dissipate, an initial number of iterations representing the burn-in phase of the chain is usually discarded.
Unlike the typically independent and identically distributed draws in traditional Monte Carlo methods, the iterates from MCMC samplers are dependent. The efficiency of the sampler hinges on the speed with which the chain navigates the state space. Samplers with high autocorrelation take much longer to fully traverse the parameter space and produce an adequate sample (one that is truly representative of the posterior density). Usually, some form of statistical analysis of the chain's output is performed to assess convergence. Cowles and Carlin (1996) review some of these methods.
In this paper, I rely on the convergence diagnostic method suggested by Raftery and Lewis (1992), which is designed to calculate the number of iterations required to estimate a posterior quantile using a single-run Markov chain. That approach suggests the number of burn-in draws to discard, the minimum number of iterations required to achieve a desired level of accuracy, and the optimal amount of “thinning” (the practice of using only every kth iteration for inference to reduce autocorrelation). Based on this diagnostic tool, I generate 72,000 draws, discard the initial 12,000 as burn-in, and thin the other 60,000 iterations, keeping only every 12th draw. The remaining sample of 5,000 parameter simulations is used for inference.26
Notes
1 The law defines disability as the inability to engage in substantial gainful activity by reason of any medically determinable physical or mental impairment that can be expected to result in death or which has lasted or can be expected to last for a continuous period of not less than 12 months. To meet this definition, a claimant must have a severe impairment(s) that renders him or her unable to do past relevant work or any other substantial gainful work that exists in the national economy.
2 In most states, a DE and a SAMC make disability determinations jointly. In Single-Decisionmaker states, no SAMC evaluates the medical evidence, except in cases with mental impairments.
3 These percentages are based on 100% extracts of the Disability Research File, encompassing the entire population of initial claimants in a given year.
4 Technical denials (over 800,000 records) are omitted because they generally lack any evaluation of the medical evidence, as the determination involves a variety of nonmedical reasons (such as lacking the required number of work credits or engaging in substantial gainful activity). In addition, I disregard claims with no applicable impairment codes. These include “no predetermined list code of medical nature applicable” (diagnosis code 2480) and “medical evidence in file but insufficient to establish diagnosis” (diagnosis code 6490).
5 The number of correlation estimates involved grows exponentially with the number of impairments included in the analysis. Focusing on the most common 100 diagnoses reasonably balances the goals of basing estimates on the overwhelming majority of claims and providing ample detail for some relatively infrequent diagnoses.
6 For an explanation of the sequential steps in the disability determination process, see Wixon and Strand (2013).
7 If the objective were simply to forecast the initial determination outcome, a univariate model with the impairments as exogenous predictors would suffice (although typically, data for primary and secondary diagnoses are only available simultaneously with the initial decision).
8 Taking account of the order of the diagnoses with the existing modeling framework would require the addition of 100 binary fields to the dependent variable side, rendering the study computationally unfeasible.
9 By Chebyshev's inequality, a t-statistic of 2 implies that at least 75% of a distribution's values are contained within 2 standard deviations from its mean (88.9% for a t-statistic of 3). These are, of course, lower bounds applying to any arbitrary distribution. For a normally distributed (or approximately normal) random variate, about 95.4% of its mass lies within 2 standard deviations of the mean.
10 See also appendix Chart A-1.
11 The numerical standard error is a measure of the asymptotic standard deviation of the posterior mean, where the asymptotic argument refers to the sample size of the simulated draws and not the data (Geweke 1992).
12 Other mental impairments with low initial-allowance percentages are omitted from Table 3 because their posterior mean correlations do not exceed the 0.10 absolute-value cutoff for inclusion. For example, substance addiction (whether alcohol or drugs) has a mean correlation with initial allowance of −0.08 and a t-statistic around −6. An anxiety disorder diagnosis is also associated with a below-average share of initial allowances (32%).
13 Anterior horn cells are the motor neurons in the gray matter of the spinal cord.
14 Not shown in Table 4, chronic renal failure has an estimated 0.092 mean correlation with the sex binary variable and a corresponding t-statistic of 8.90.
15 In the disability determination process, a claimant shall not be considered disabled if drug or alcohol addiction (DAA) is a contributing factor material to the determination that the individual is disabled. Addiction is considered material if the evidence establishes that the individual would not be disabled if he or she stopped using drugs or alcohol. The DE decides materiality and the SAMC determines the medical aspects of the DAA analysis—that is, what limitations a claimant would have in the absence of DAA.
16 In the remainder of this paper, I often cite percentages with which a given diagnosis (or diagnostic category) combines with another specific diagnosis (or category) in the 2009 initial claims. For brevity, I do not repeatedly indicate that those percentages are not shown in the correlation tables. Statistics on these comorbidity frequencies are available on request, subject to nondisclosure rules (Javier.Meseguer@ssa.gov).
17 In a Swedish national cohort study of over 6 million adults, Crump and others (2013) find that the leading causes of premature death in schizophrenics are heart disease and cancer, which tend to be underdiagnosed.
18 Note that under the disability determination criteria, DI beneficiaries with Medicare have a nonaddiction disability. In other words, if addiction entered the disability determination, it was not material. In addition, DI beneficiaries receive disability benefits for at least 2 years before qualifying for Medicare—thus, they have a longer period of disability with potentially increasing need for pain medication. As Morden and others point out, “Our claims-based analysis has important limitations. For our annual enrollment cohorts, we do not know the medical condition or combination of conditions that resulted in qualification for disability insurance. Because disability assessments precede Medicare enrollment by two years, Medicare claims are not reliable for determining cause of disability.” Hence, to the extent that DI beneficiaries with musculoskeletal diagnoses have an addiction to opioids, the root cause likely lies in the overprescription of Medicare-covered opioids, which mirrors the behavior of insurers in the general population.
19 For epidemiological findings of comorbid respiratory and cardiovascular diseases, see Mannino, Davis, and Disantostefano (2013).
20 D'Andrea, Vigliarolo, and Sanguinetti (2010) discuss comorbidity between digestive and respiratory impairments.
21 There is some evidence that certain anti-HIV drugs may increase uric acid levels in HIV-positive patients (Creighton and others 2005).
22 As pointed out by Rossi, Allenby, and McCulloch (2005), if one wishes to restrict the coefficients of a particular explanatory variable to be equal across the p responses, then the identification constraints must be modified accordingly.
23 In a series of detailed Monte Carlo studies, Geweke, Keane, and Runkle (1994, 1997) compared the sampling performance of various estimators for the MNP and multinomial multiperiod probit models. Their findings point to a clear advantage of the Gibbs sampler over simulated maximum likelihood (SML) estimation and the method of simulated moments (MSM) using the Geweke-Hajivassilou-Keane (GHK) probability simulator.
24 Notice that from a classical inferential perspective, each iteration of the Monte Carlo Expectation-Maximization algorithm suggested by Xu and Craig (2010) involves multiple iterations of a Gibbs sampler in the expectation step to generate draws of the truncated multivariate normal latent variables. This approach is quite inefficient compared with the sampler of Edwards and Allenby (2003).
25 To the best of my knowledge, one of the highest dimensional applications of an unrestricted MVP model in the econometrics literature involves the so-called “scotch data set” comprising household supermarket purchase decisions between p = 21 different brands of Scotch whisky. Edwards and Allenby (2003) discuss the feasibility of their proposed sampler to easily handle problems involving 50 or more dimensions. Hahn, Carvalho, and Scott (2012) consider an application of congressional voting patterns with p = 30, as well as simulated observations from a data-generating process with p = 100. However, the authors rely on a latent factor probit model with a much smaller number of factor loadings. Their approach appears particularly promising when p exceeds the number of observations n.
26 I used the Matlab CODA function (LeSage 2010) to diagnose convergence.
References
Albert, James H., and Siddhartha Chib. 1993. “Bayesian Analysis of Binary and Polychotomous Response Data.” Journal of the American Statistical Association 88(422): 669–679.
American Cancer Society. 2018. “Key Statistics for Breast Cancer in Men.” https://www.cancer.org/cancer/breast-cancer-in-men/about/key-statistics.html.
American Thoracic Society. 1999. “Statement on Sarcoidosis.” American Journal of Respiratory Critical Care Medicine 160(2): 736–755.
Barnard, John, Robert McCulloch, and Xiao-Li Meng. 2000. “Modeling Covariance Matrices in Terms of Standard Deviations and Correlations, with Application to Shrinkage.” Statistica Sinica 10(4): 1281–1311.
Centers for Disease Control and Prevention. 2011. “Vital Signs: Overdoses of Prescription Opioid Pain Relievers—United States, 1999–2008.” Morbidity and Mortality Weekly Report 60(43): 1487–1492.
Chib, Siddhartha, and Edward Greenberg. 1998. “Analysis of Multivariate Probit Models.” Biometrika 85(2): 347–361.
Cowles, Mary Kathryn, and Bradley P. Carlin. 1996. “Markov Chain Monte Carlo Convergence Diagnostics: A Comparative Review.” Journal of the American Statistical Association 91(434): 883–904.
Creighton, S., Robert F. Miller, Simon Edwards, A. Copas, and Patrick French. 2005. “Is Ritonavir Boosting Associated with Gout?” International Journal of STD and AIDS 16(5): 362–364.
Crump, Casey, Marilyn A. Winkleby, Kristina Sundquist, and Jan Sundquist. 2013. “Comorbidities and Mortality in Persons with Schizophrenia: A Swedish National Cohort Study.” American Journal of Psychiatry 170(3): 324–333.
D'Andrea, Nadia, Rossana Vigliarolo, and Claudio M. Sanguinetti. 2010. “Respiratory Involvement in Inflammatory Bowel Diseases.” Multidisciplinary Respiratory Medicine 5(3): 173–182.
Edwards, Yancy D., and Greg M. Allenby. 2003. “Multivariate Analysis of Multiple Response Data.” Journal of Marketing Research 40(3): 321–334.
Fourney, Daryl R., Donald F. Schomer, Remi Nader, Jennifer Chlan-Fourney, Dima Suki, Kamran Ahrar, Laurence D. Rhines, and Ziya L. Gokaslan. 2003. “Percutaneous Vertebroplasty and Kyphoplasty for Painful Vertebral Body Fractures in Cancer Patients.” Journal of Neurosurgery: Spine 98(1): 21–30.
Frisch, Morten, Robert J. Biggar, Eric A. Engels, and James J. Goedert. 2001. “Association of Cancer with AIDS-Related Immunosuppression in Adults.” Journal of the American Medical Association 285(13): 1736–1745.
Gadalla, Shahinaz M., Marie Lund, Ruth M. Pfeiffer, Sanne Gørtz, Christine M. Mueller, Richard T. Moxley III, Sigurdur Y. Kristinsson, Magnus Björkholm, Fatma M. Shebl, James E. Hilbert, Ola Landgren, Jan Wohlfahrt, Mads Melbye, and Mark H. Greene. 2011. “Cancer Risk among Patients with Myotonic Muscular Dystrophy.” Journal of the American Medical Association, 306(22): 2480–2486.
Geweke, John. 1992. “Evaluating the Accuracy of Sampling-Based Approaches to the Calculation of Posterior Moments.” In Bayesian Statistics 4, edited by J. M. Bernardo, J. O. Berger, A. P. Dawid, and A. F. M. Smith, 169–193. New York, NY: Oxford University Press.
Geweke, John, Michael Keane, and David Runkle. 1994. “Alternative Computational Approaches to Inference in the Multinomial Probit Model.” The Review of Economics and Statistics 76(4): 609–632.
———. 1997. “Statistical Inference in the Multinomial Multiperiod Probit Model.” Journal of Econometrics 80(1): 125–165.
Gibbons, Robert D., and Virginia Wilcox-Gök. 1998. “Health Service Utilization and Insurance Coverage: A Multivariate Probit Analysis.” Journal of the American Statistical Association 93(441): 63–72.
Gordon, Jennifer L., Kim L. Lavoie, André Arsenault, Blaine Ditto, and Simon L. Bacon. 2012. “Mood Disorders and Cardiovascular Disease.” In Clinical, Research and Treatment Approaches to Affective Disorders, edited by Mario Francisco Juruena, 73–102. Rijeka (Croatia): InTech.
Grant, Bridget F., Frederick S. Stinson, Deborah A. Dawson, S. Patricia Chou, Mary C. Dufour, Wilson Compton, Roger P. Pickering, and Kenneth Kaplan. 2004. “Prevalence and Co-Occurrence of Substance Use Disorders and Independent Mood and Anxiety Disorders: Results from the National Epidemiologic Survey on Alcohol and Related Conditions.” Archives of General Psychiatry 61(8): 807–816.
Hahn, P. Richard, Carlos M. Carvalho, and James G. Scott. 2012. “A Sparse Factor Analytic Probit Model for Congressional Voting Patterns.” Journal of the Royal Statistical Society (Series C: Applied Statistics) 61(4): 619–635.
Hodgson, Richard, Hiram J. Wildgust, and Chris J. Bushe. 2010. “Cancer and Schizophrenia: Is There a Paradox?” Journal of Psychopharmacology 24(4_suppl): 51–60.
Huntley, Alyson L., Rachel Johnson, Sarah Purdy, Jose M. Valderas, and Chris Salisbury. 2012. “Measures of Multimorbidity and Morbidity Burden for Use in Primary Care and Community Settings: A Systematic Review and Guide.” Annals of Family Medicine 10(2): 134–141.
Johannesson, Anton, Gert-Uno Larsson, Nerrolyn Ramstrand, Alekdandra Turkiewicz, Ann-Britt Wiréhn, and Isam Atroshi. 2009. “Incidence of Lower-Limb Amputation in the Diabetic and Nondiabetic General Population.” Diabetes Care 32(2): 275–280.
Kao, Hung-Teh, Stephen L. Buka, Karl T. Kelsey, David F. Gruber, and Barbara Porton. 2010. “The Correlation between Rates of Cancer and Autism: An Exploratory Ecological Investigation,” PloS One 5(2): 1–8.
LeSage, James P. 2010. “Econometrics Toolbox.” http://www.spatial-econometrics.com/.
Levey, A. S., R. Atkins, J. Coresh, E. P. Cohen, A. J. Collins, K.-U. Eckardt, M. E. Nahas, B. L. Jaber, M. Jadoul, A. Levin, N. R. Powe, J. Rossert, D. C. Wheeler, N. Lameire, and G. Eknoyan. 2007. “Chronic Kidney Disease as a Global Public Health Problem: Approaches and Initiatives—A Position Statement from Kidney Disease Improving Global Outcomes.” Kidney International 72(3): 247–259.
Mani, Deepthi, Missak Haigentz, Jr., and David M. Aboulafia. 2012. “Lung Cancer in HIV Infection.” Clinical Lung Cancer 13(1): 6–13.
Mannino, David M., Kourtney J. Davis, and Rachael L. Disantostefano. 2013. “Chronic Respiratory Disease, Comorbid Cardiovascular Disease and Mortality in a Representative Adult US Cohort.” Respirology 18(7): 1083–1088.
Marengoni, Alessandra, Sara Angleman, René Melis, Francesca Mangialasche, Anita Karp, Annika Garmen, Bettina Meinow, and Laura Fratiglioni. 2011. “Aging with Multimorbidity: A Systematic Review of the Literature.” Ageing Research Reviews 10(4): 430–439.
McCulloch, Robert E., Nicholas G. Polson, and Peter E. Rossi. 2000. “A Bayesian Analysis of the Multinomial Probit Model with Fully Identified Parameters.” Journal of Econometrics 99(1): 173–193.
McCulloch, Robert E., and Peter E. Rossi. 1994. “An Exact Likelihood Analysis of the Multinomial Probit Model.” Journal of Econometrics 64(1–2): 207–240.
Meseguer, Javier. 2013. “Outcome Variation in the Social Security Disability Insurance Program: The Role of Primary Diagnoses.” Social Security Bulletin 73(2): 39–75.
Morden, Nancy E., Jeffrey C. Munson, Carrie H. Colla, Jonathan S. Skinner, Julie P. W. Bynum, Weiping Zhou, and Ellen Meara. 2014. “Prescription Opioid Use Among Disabled Medicare Beneficiaries: Intensity, Trends, and Regional Variation.” Medical Care 52(9): 852–859.
Nikolov, Igor G., Ognen Ivanovski, and Nobuhiko Joki. 2012. “The New Kidney and Bone Disease: Chronic Kidney Disease-Mineral and Bone Disorder (CKD-MBD).” In Chronic Kidney Disease, edited by Monika Göőz, 25–46. Rijeka (Croatia): InTech.
Nobile, Agostino. 1998. “A Hybrid Markov Chain for the Bayesian Analysis of the Multinomial Probit Model.” Statistics and Computing 8(3): 229–242.
Patel, Pragna, Debra L. Hanson, Patrick S. Sullivan, Richard M. Novak, Anne C. Moorman, Tony C. Tong, Scott D. Holmberg, and John T. Brooks. 2008. “Incidence of Types of Cancer among HIV-Infected Persons Compared with the General Population in the United States, 1992–2003.” Annals of Internal Medicine 148(10): 728–736.
Raftery, Adrian E., and Steven M. Lewis. 1992. “How Many Iterations in the Gibbs Sampler?” In Bayesian Statistics 4, edited by J. M. Bernardo, J. O. Berger, A. P. Dawid, and A. F. M. Smith, 763–773. New York, NY: Oxford University Press.
Rossi, Peter E., Greg M. Allenby, and Robert McCulloch. 2005. Bayesian Statistics and Marketing. Chichester (England): John Wiley & Sons.
Sinnige, Judith, Joke C. Korevaar, Gert P. Westert, Peter Spreeuwenberg, François G. Schellevis, and Jozé C. C. Braspenning. 2015. “Multimorbidity Patterns in a Primary Care Population Aged 55 Years and Over.” Family Practice 32(5): 505–513.
Social Security Administration. 2017. “Program Operations Manual System (POMS) Section DI 26510.015. Completing Item 16A and 16B (Primary and Secondary Diagnosis, Body System Code, and Impairment Code) on the SSA-831 Disability Determination and Transmittal.” http://policy.ssa.gov/poms.nsf/lnx/0426510015.
Spek, Annelies A., and Saskia G. M. Wouters. 2010. “Autism and Schizophrenia in High Functioning Adults: Behavioral Differences and Overlap.” Research in Autism Spectrum Disorders 4(4): 709–717.
[SSA] See Social Security Administration.
Wixon, Bernard, and Alexander Strand. 2013. “Identifying SSA's Sequential Disability Determination Steps Using Administrative Data.” Research and Statistics Note No. 2013-01. Washington, DC: Social Security Administration. https://www.ssa.gov/policy/docs/rsnotes/rsn2013-01.html.
Xu, Huiping, and Bruce A. Craig. 2010. “Likelihood Analysis of Multivariate Probit Models Using a Parameter Expanded MCEM Algorithm.” Technometrics 52(2): 340–348.
Zhu, Yanyan, Bhavik J. Pandya, and Hyon K. Choi. 2012. “Comorbidities of Gout and Hyperuricemia in the US General Population: The National Health and Nutrition Examination Survey 2007–2008.” The American Journal of Medicine 125(7): 679–687.